کد مقاله | کد نشریه | سال انتشار | مقاله انگلیسی | نسخه تمام متن |
---|---|---|---|---|
515538 | 867041 | 2009 | 15 صفحه PDF | دانلود رایگان |
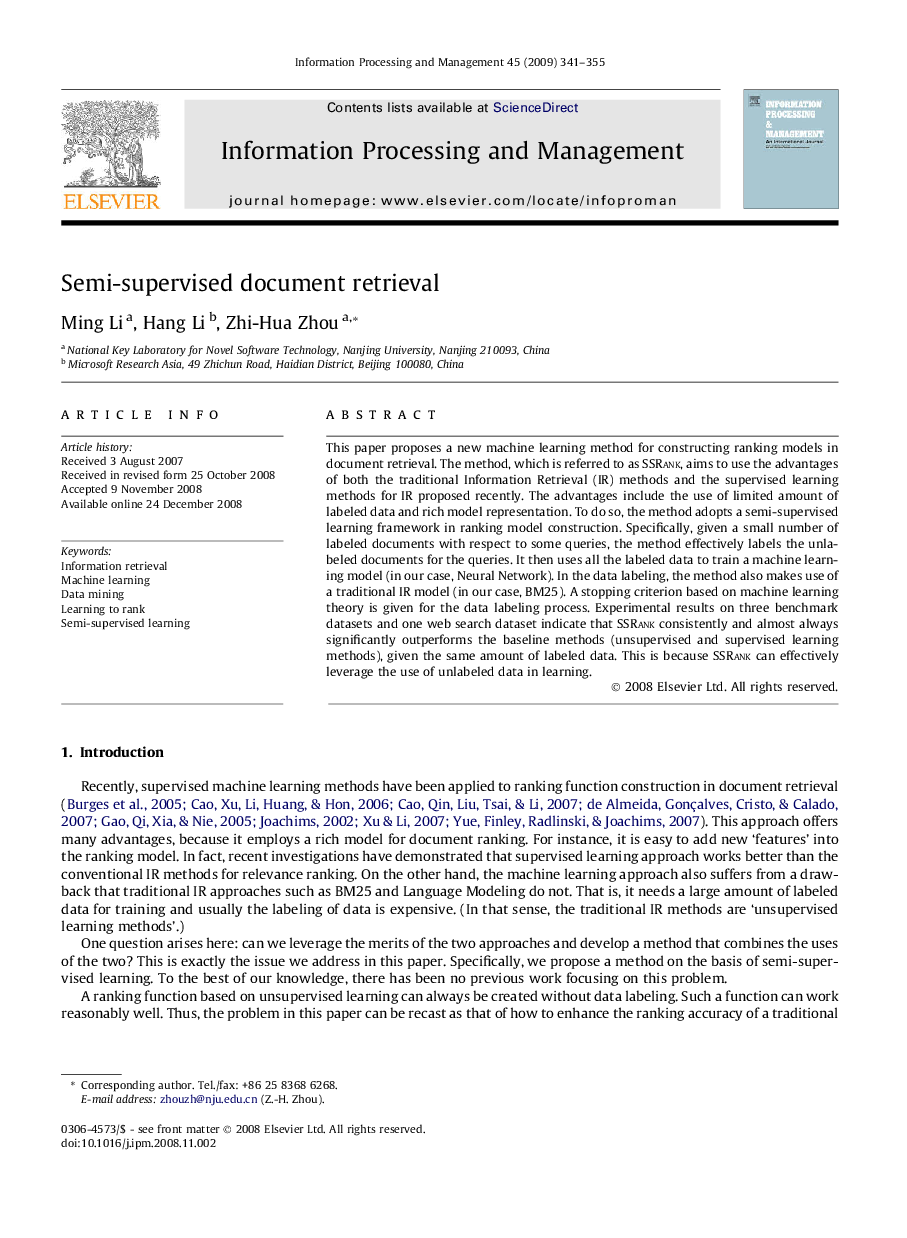
This paper proposes a new machine learning method for constructing ranking models in document retrieval. The method, which is referred to as SSRank, aims to use the advantages of both the traditional Information Retrieval (IR) methods and the supervised learning methods for IR proposed recently. The advantages include the use of limited amount of labeled data and rich model representation. To do so, the method adopts a semi-supervised learning framework in ranking model construction. Specifically, given a small number of labeled documents with respect to some queries, the method effectively labels the unlabeled documents for the queries. It then uses all the labeled data to train a machine learning model (in our case, Neural Network). In the data labeling, the method also makes use of a traditional IR model (in our case, BM25). A stopping criterion based on machine learning theory is given for the data labeling process. Experimental results on three benchmark datasets and one web search dataset indicate that SSRank consistently and almost always significantly outperforms the baseline methods (unsupervised and supervised learning methods), given the same amount of labeled data. This is because SSRank can effectively leverage the use of unlabeled data in learning.
Journal: Information Processing & Management - Volume 45, Issue 3, May 2009, Pages 341–355