کد مقاله | کد نشریه | سال انتشار | مقاله انگلیسی | نسخه تمام متن |
---|---|---|---|---|
515933 | 867150 | 2009 | 12 صفحه PDF | دانلود رایگان |
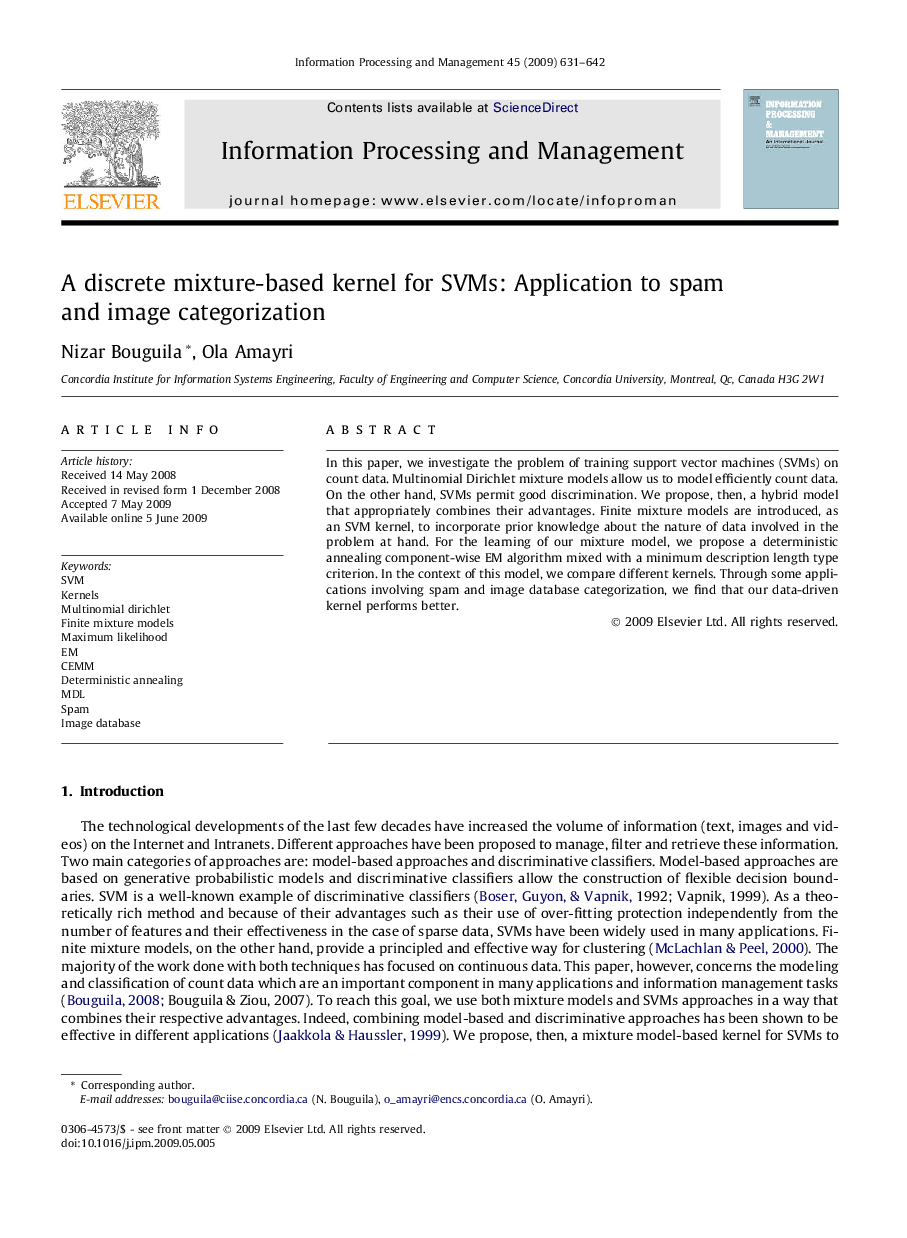
In this paper, we investigate the problem of training support vector machines (SVMs) on count data. Multinomial Dirichlet mixture models allow us to model efficiently count data. On the other hand, SVMs permit good discrimination. We propose, then, a hybrid model that appropriately combines their advantages. Finite mixture models are introduced, as an SVM kernel, to incorporate prior knowledge about the nature of data involved in the problem at hand. For the learning of our mixture model, we propose a deterministic annealing component-wise EM algorithm mixed with a minimum description length type criterion. In the context of this model, we compare different kernels. Through some applications involving spam and image database categorization, we find that our data-driven kernel performs better.
Journal: Information Processing & Management - Volume 45, Issue 6, November 2009, Pages 631–642