کد مقاله | کد نشریه | سال انتشار | مقاله انگلیسی | نسخه تمام متن |
---|---|---|---|---|
521977 | 867803 | 2008 | 26 صفحه PDF | دانلود رایگان |
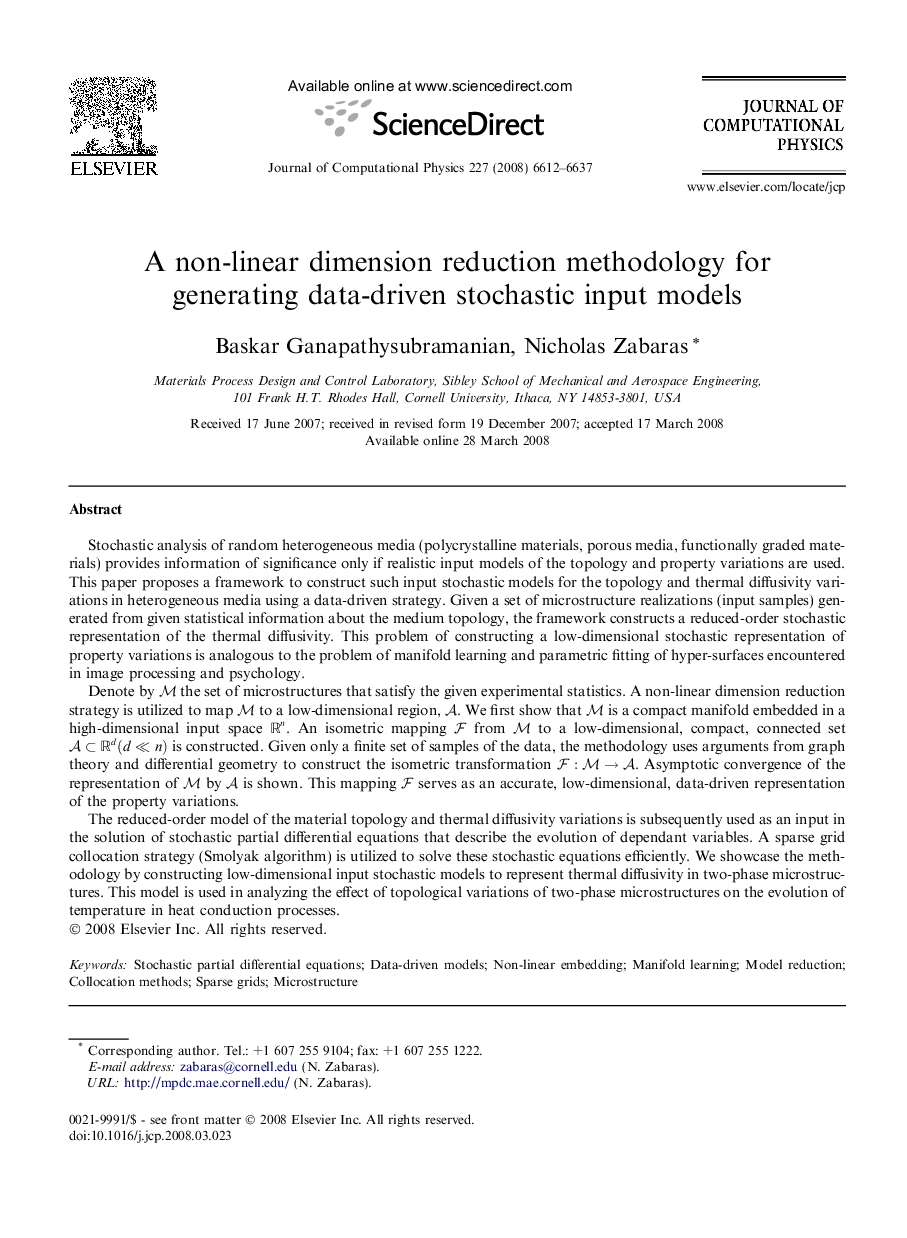
Stochastic analysis of random heterogeneous media (polycrystalline materials, porous media, functionally graded materials) provides information of significance only if realistic input models of the topology and property variations are used. This paper proposes a framework to construct such input stochastic models for the topology and thermal diffusivity variations in heterogeneous media using a data-driven strategy. Given a set of microstructure realizations (input samples) generated from given statistical information about the medium topology, the framework constructs a reduced-order stochastic representation of the thermal diffusivity. This problem of constructing a low-dimensional stochastic representation of property variations is analogous to the problem of manifold learning and parametric fitting of hyper-surfaces encountered in image processing and psychology.Denote by MM the set of microstructures that satisfy the given experimental statistics. A non-linear dimension reduction strategy is utilized to map MM to a low-dimensional region, AA. We first show that MM is a compact manifold embedded in a high-dimensional input space RnRn. An isometric mapping FF from MM to a low-dimensional, compact, connected set A⊂Rd(d≪n)A⊂Rd(d≪n) is constructed. Given only a finite set of samples of the data, the methodology uses arguments from graph theory and differential geometry to construct the isometric transformation F:M→AF:M→A. Asymptotic convergence of the representation of MM by AA is shown. This mapping FF serves as an accurate, low-dimensional, data-driven representation of the property variations.The reduced-order model of the material topology and thermal diffusivity variations is subsequently used as an input in the solution of stochastic partial differential equations that describe the evolution of dependant variables. A sparse grid collocation strategy (Smolyak algorithm) is utilized to solve these stochastic equations efficiently. We showcase the methodology by constructing low-dimensional input stochastic models to represent thermal diffusivity in two-phase microstructures. This model is used in analyzing the effect of topological variations of two-phase microstructures on the evolution of temperature in heat conduction processes.
Journal: Journal of Computational Physics - Volume 227, Issue 13, 20 June 2008, Pages 6612–6637