کد مقاله | کد نشریه | سال انتشار | مقاله انگلیسی | نسخه تمام متن |
---|---|---|---|---|
529790 | 869708 | 2014 | 9 صفحه PDF | دانلود رایگان |
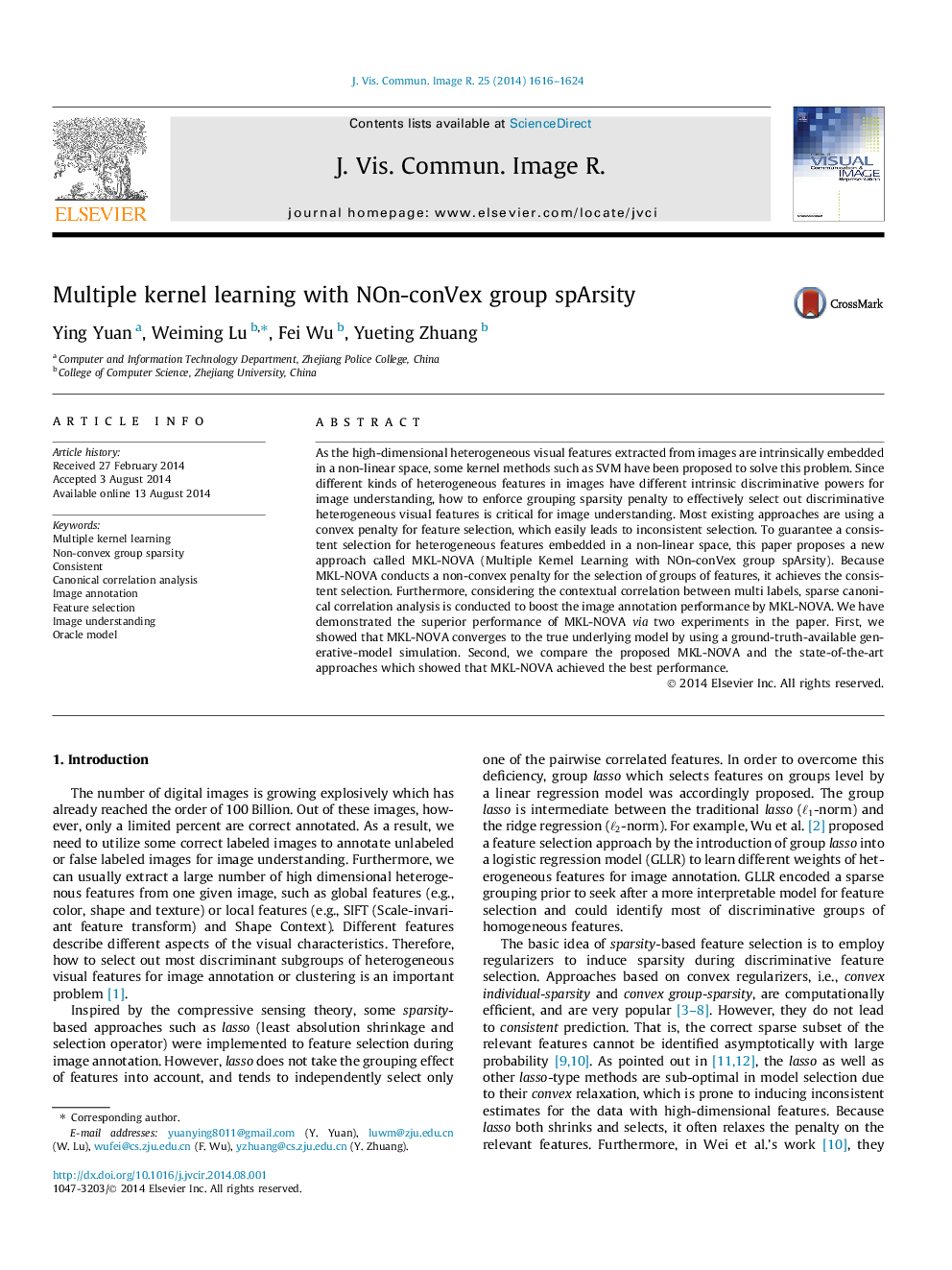
• Enforce grouping sparsity penalty to select out discriminative visual features.
• Propose non-convex penalty to guarantee a consistent selection for features.
• Introduce sparse canonical correlation analysis to boost image annotation.
As the high-dimensional heterogeneous visual features extracted from images are intrinsically embedded in a non-linear space, some kernel methods such as SVM have been proposed to solve this problem. Since different kinds of heterogeneous features in images have different intrinsic discriminative powers for image understanding, how to enforce grouping sparsity penalty to effectively select out discriminative heterogeneous visual features is critical for image understanding. Most existing approaches are using a convex penalty for feature selection, which easily leads to inconsistent selection. To guarantee a consistent selection for heterogeneous features embedded in a non-linear space, this paper proposes a new approach called MKL-NOVA (Multiple Kernel Learning with NOn-conVex group spArsity). Because MKL-NOVA conducts a non-convex penalty for the selection of groups of features, it achieves the consistent selection. Furthermore, considering the contextual correlation between multi labels, sparse canonical correlation analysis is conducted to boost the image annotation performance by MKL-NOVA. We have demonstrated the superior performance of MKL-NOVA via two experiments in the paper. First, we showed that MKL-NOVA converges to the true underlying model by using a ground-truth-available generative-model simulation. Second, we compare the proposed MKL-NOVA and the state-of-the-art approaches which showed that MKL-NOVA achieved the best performance.
Journal: Journal of Visual Communication and Image Representation - Volume 25, Issue 7, October 2014, Pages 1616–1624