کد مقاله | کد نشریه | سال انتشار | مقاله انگلیسی | نسخه تمام متن |
---|---|---|---|---|
529887 | 869719 | 2015 | 14 صفحه PDF | دانلود رایگان |
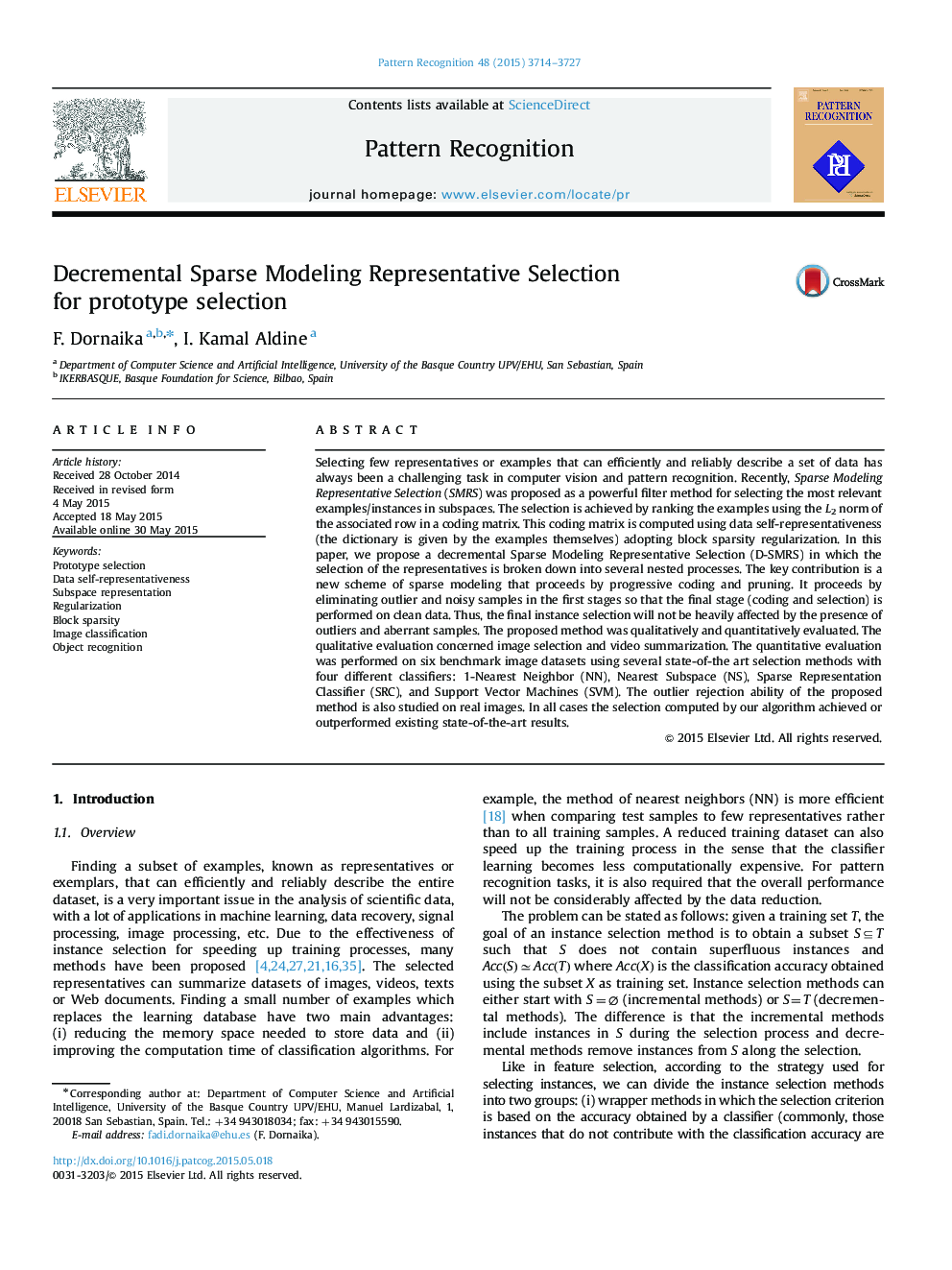
• A novel framework for prototype selection in subspaces is proposed.
• A recursive prototype elimination is adopted.
• It iterates a data self-representativeness coding with a block sparsity constraint.
• Classification performance after selection is assessed on five public image datasets.
• We use K-NN, Nearest subspace, Sparse Representation Classifier, and SVM.
Selecting few representatives or examples that can efficiently and reliably describe a set of data has always been a challenging task in computer vision and pattern recognition. Recently, Sparse Modeling Representative Selection (SMRS) was proposed as a powerful filter method for selecting the most relevant examples/instances in subspaces. The selection is achieved by ranking the examples using the L2 norm of the associated row in a coding matrix. This coding matrix is computed using data self-representativeness (the dictionary is given by the examples themselves) adopting block sparsity regularization. In this paper, we propose a decremental Sparse Modeling Representative Selection (D-SMRS) in which the selection of the representatives is broken down into several nested processes. The key contribution is a new scheme of sparse modeling that proceeds by progressive coding and pruning. It proceeds by eliminating outlier and noisy samples in the first stages so that the final stage (coding and selection) is performed on clean data. Thus, the final instance selection will not be heavily affected by the presence of outliers and aberrant samples. The proposed method was qualitatively and quantitatively evaluated. The qualitative evaluation concerned image selection and video summarization. The quantitative evaluation was performed on six benchmark image datasets using several state-of-the art selection methods with four different classifiers: 1-Nearest Neighbor (NN), Nearest Subspace (NS), Sparse Representation Classifier (SRC), and Support Vector Machines (SVM). The outlier rejection ability of the proposed method is also studied on real images. In all cases the selection computed by our algorithm achieved or outperformed existing state-of-the-art results.
Journal: Pattern Recognition - Volume 48, Issue 11, November 2015, Pages 3714–3727