کد مقاله | کد نشریه | سال انتشار | مقاله انگلیسی | نسخه تمام متن |
---|---|---|---|---|
530084 | 869740 | 2013 | 7 صفحه PDF | دانلود رایگان |
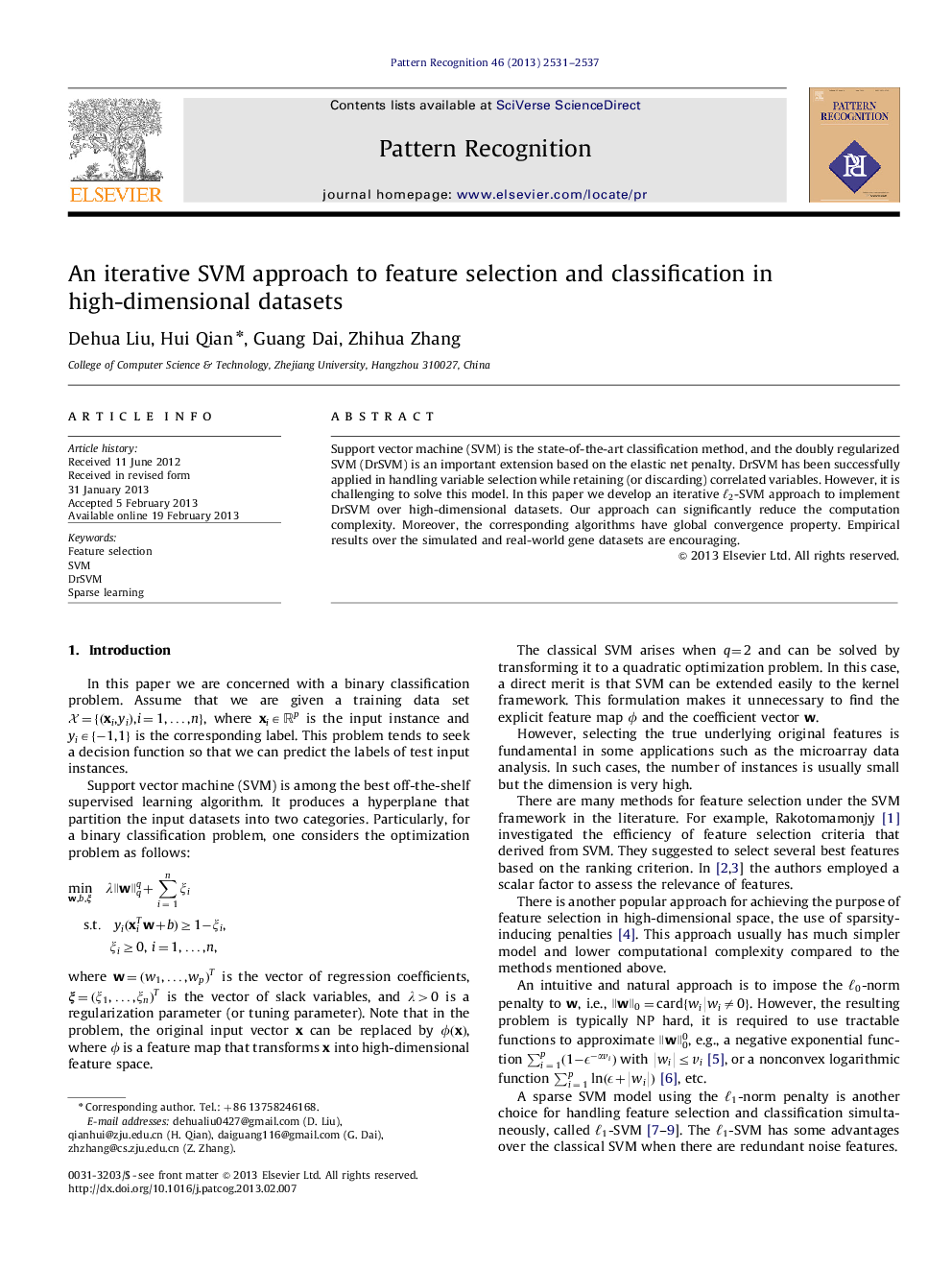
Support vector machine (SVM) is the state-of-the-art classification method, and the doubly regularized SVM (DrSVM) is an important extension based on the elastic net penalty. DrSVM has been successfully applied in handling variable selection while retaining (or discarding) correlated variables. However, it is challenging to solve this model. In this paper we develop an iterative ℓ2-SVMℓ2-SVM approach to implement DrSVM over high-dimensional datasets. Our approach can significantly reduce the computation complexity. Moreover, the corresponding algorithms have global convergence property. Empirical results over the simulated and real-world gene datasets are encouraging.
► We developed the efficient algorithms for DrSVM model with high-dimensional features.
► Our algorithms transform the large p problem into a series of small n problems.
► Our algorithms have complexity O(K(n3+np))O(K(n3+np)) and global convergence property.
► We also explored the effect of tuning parameters on the speed of algorithms.
Journal: Pattern Recognition - Volume 46, Issue 9, September 2013, Pages 2531–2537