کد مقاله | کد نشریه | سال انتشار | مقاله انگلیسی | نسخه تمام متن |
---|---|---|---|---|
530143 | 869745 | 2012 | 12 صفحه PDF | دانلود رایگان |
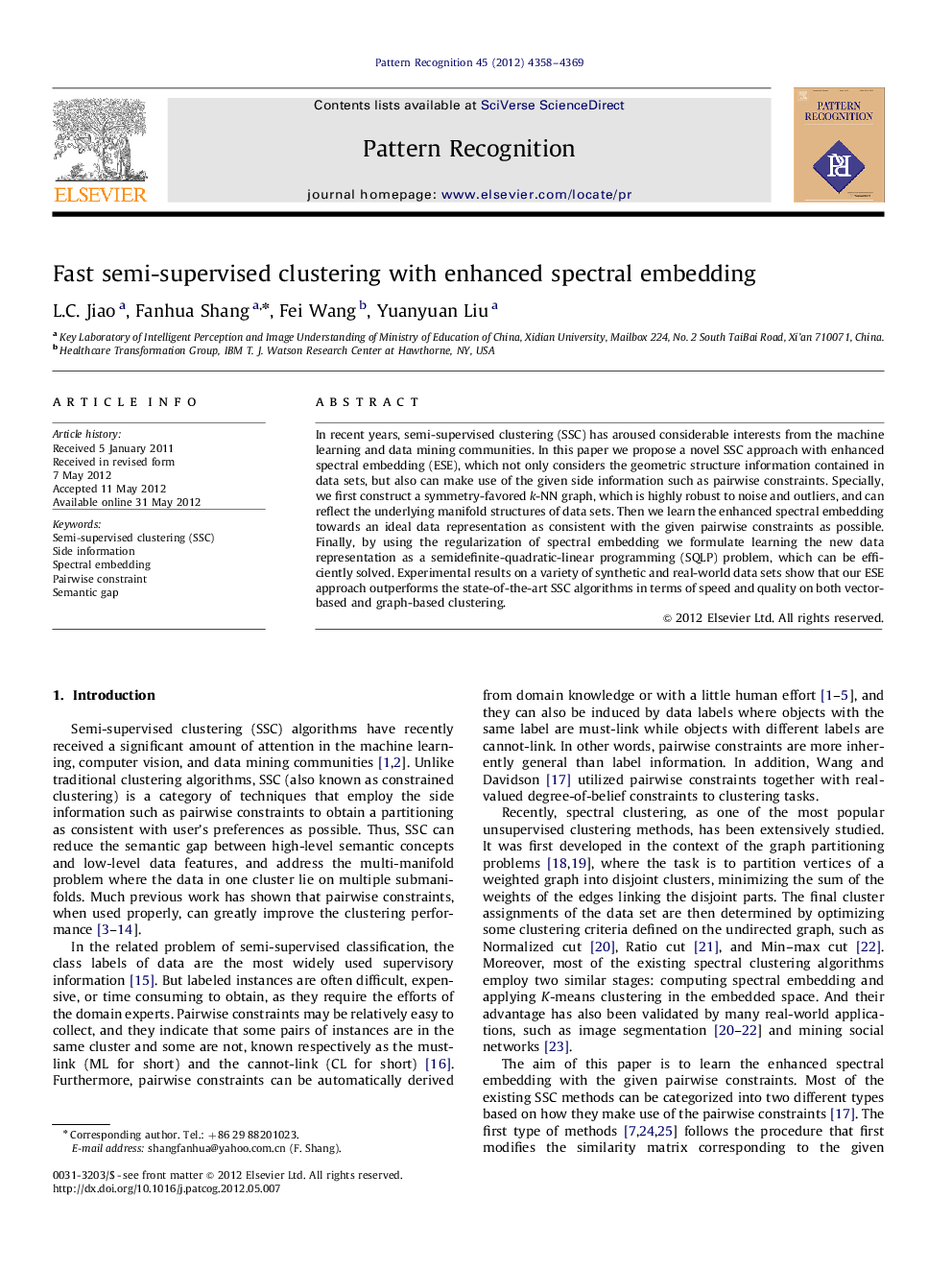
In recent years, semi-supervised clustering (SSC) has aroused considerable interests from the machine learning and data mining communities. In this paper we propose a novel SSC approach with enhanced spectral embedding (ESE), which not only considers the geometric structure information contained in data sets, but also can make use of the given side information such as pairwise constraints. Specially, we first construct a symmetry-favored k-NN graph, which is highly robust to noise and outliers, and can reflect the underlying manifold structures of data sets. Then we learn the enhanced spectral embedding towards an ideal data representation as consistent with the given pairwise constraints as possible. Finally, by using the regularization of spectral embedding we formulate learning the new data representation as a semidefinite-quadratic-linear programming (SQLP) problem, which can be efficiently solved. Experimental results on a variety of synthetic and real-world data sets show that our ESE approach outperforms the state-of-the-art SSC algorithms in terms of speed and quality on both vector-based and graph-based clustering.
► A symmetry-favored k-NN graph is constructed.
► Learning the spectral representation can be formulated as an SQLP problem.
► An SSC approach with enhanced spectral embedding (ESE) is proposed.
► Promising experimental results on vector and graph based clustering are presented.
Journal: Pattern Recognition - Volume 45, Issue 12, December 2012, Pages 4358–4369