کد مقاله | کد نشریه | سال انتشار | مقاله انگلیسی | نسخه تمام متن |
---|---|---|---|---|
530147 | 869745 | 2012 | 14 صفحه PDF | دانلود رایگان |
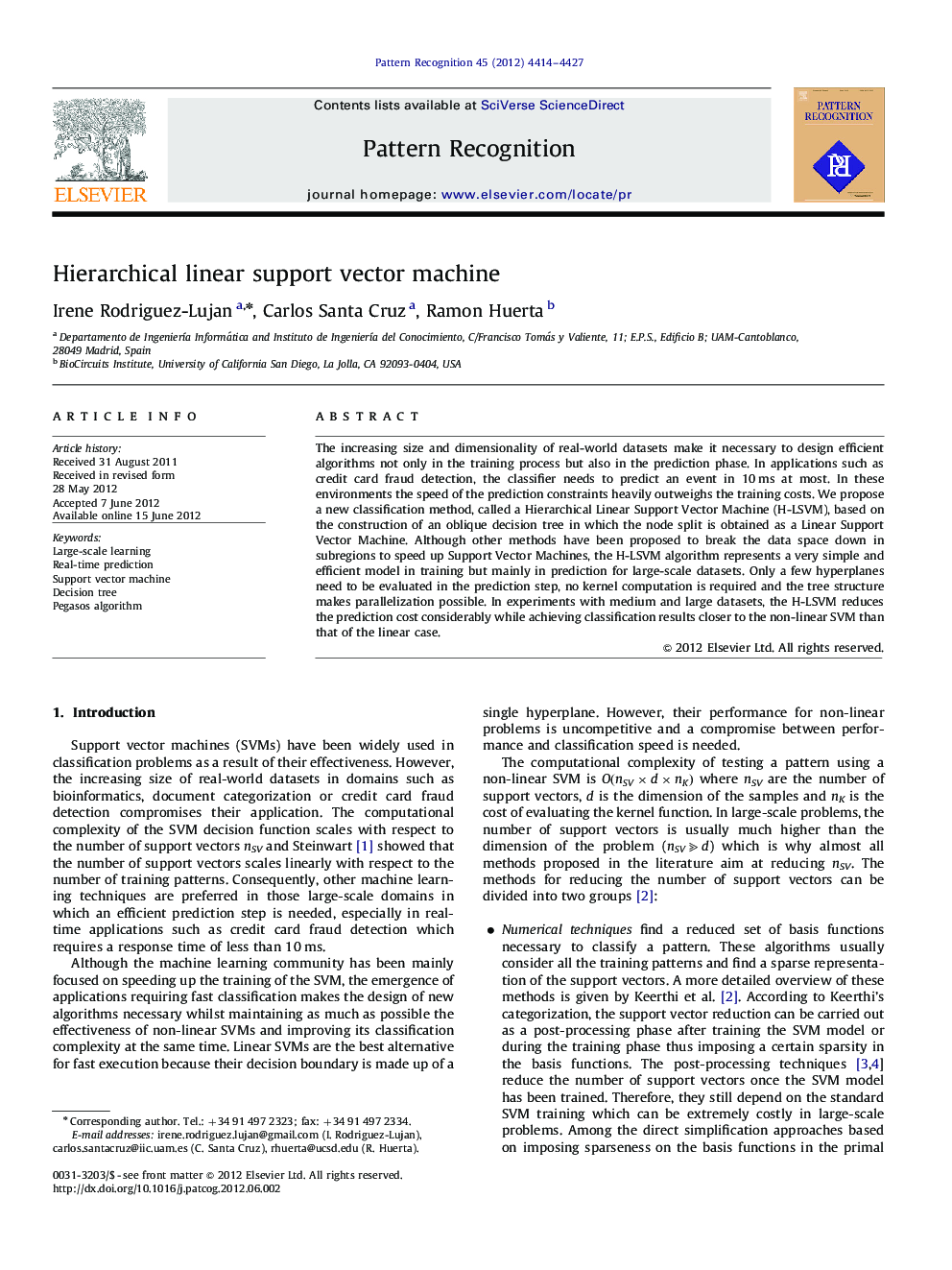
The increasing size and dimensionality of real-world datasets make it necessary to design efficient algorithms not only in the training process but also in the prediction phase. In applications such as credit card fraud detection, the classifier needs to predict an event in 10 ms at most. In these environments the speed of the prediction constraints heavily outweighs the training costs. We propose a new classification method, called a Hierarchical Linear Support Vector Machine (H-LSVM), based on the construction of an oblique decision tree in which the node split is obtained as a Linear Support Vector Machine. Although other methods have been proposed to break the data space down in subregions to speed up Support Vector Machines, the H-LSVM algorithm represents a very simple and efficient model in training but mainly in prediction for large-scale datasets. Only a few hyperplanes need to be evaluated in the prediction step, no kernel computation is required and the tree structure makes parallelization possible. In experiments with medium and large datasets, the H-LSVM reduces the prediction cost considerably while achieving classification results closer to the non-linear SVM than that of the linear case.
► Simple and efficient classification method for real-time classification.
► Oblique decision tree in which the node split is a Linear Support Vector Machine.
► Training and prediction complexities and generalization error bound.
► Significant reduction of the nonlinear SVM prediction cost.
► Classification accuracy closer to the nonlinear SVM than to the linear SVM in most cases.
Journal: Pattern Recognition - Volume 45, Issue 12, December 2012, Pages 4414–4427