کد مقاله | کد نشریه | سال انتشار | مقاله انگلیسی | نسخه تمام متن |
---|---|---|---|---|
530303 | 869756 | 2012 | 10 صفحه PDF | دانلود رایگان |
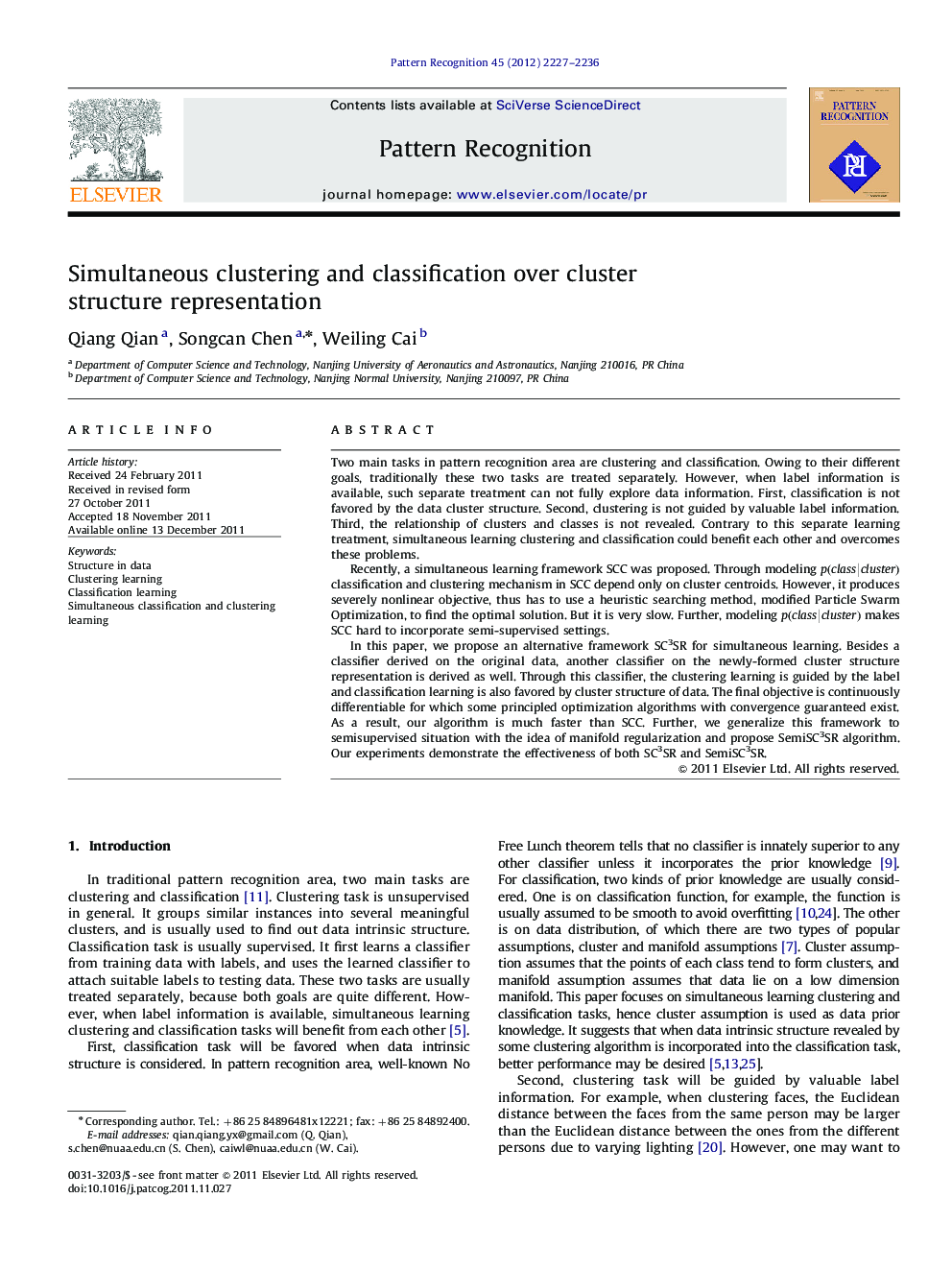
Two main tasks in pattern recognition area are clustering and classification. Owing to their different goals, traditionally these two tasks are treated separately. However, when label information is available, such separate treatment can not fully explore data information. First, classification is not favored by the data cluster structure. Second, clustering is not guided by valuable label information. Third, the relationship of clusters and classes is not revealed. Contrary to this separate learning treatment, simultaneous learning clustering and classification could benefit each other and overcomes these problems.Recently, a simultaneous learning framework SCC was proposed. Through modeling p(class|cluster)p(class|cluster) classification and clustering mechanism in SCC depend only on cluster centroids. However, it produces severely nonlinear objective, thus has to use a heuristic searching method, modified Particle Swarm Optimization, to find the optimal solution. But it is very slow. Further, modeling p(class|cluster)p(class|cluster) makes SCC hard to incorporate semi-supervised settings.In this paper, we propose an alternative framework SC3SR for simultaneous learning. Besides a classifier derived on the original data, another classifier on the newly-formed cluster structure representation is derived as well. Through this classifier, the clustering learning is guided by the label and classification learning is also favored by cluster structure of data. The final objective is continuously differentiable for which some principled optimization algorithms with convergence guaranteed exist. As a result, our algorithm is much faster than SCC. Further, we generalize this framework to semisupervised situation with the idea of manifold regularization and propose SemiSC3SR algorithm. Our experiments demonstrate the effectiveness of both SC3SR and SemiSC3SR.
► Propose a new simultaneously learning classification and clustering algorithm SC3SR.
► Generalize SC3SR to semi-supervised situation and develop SemiSC3SR.
► Examine their effectiveness and efficiency through experiments.
Journal: Pattern Recognition - Volume 45, Issue 6, June 2012, Pages 2227–2236