کد مقاله | کد نشریه | سال انتشار | مقاله انگلیسی | نسخه تمام متن |
---|---|---|---|---|
530465 | 869769 | 2014 | 14 صفحه PDF | دانلود رایگان |
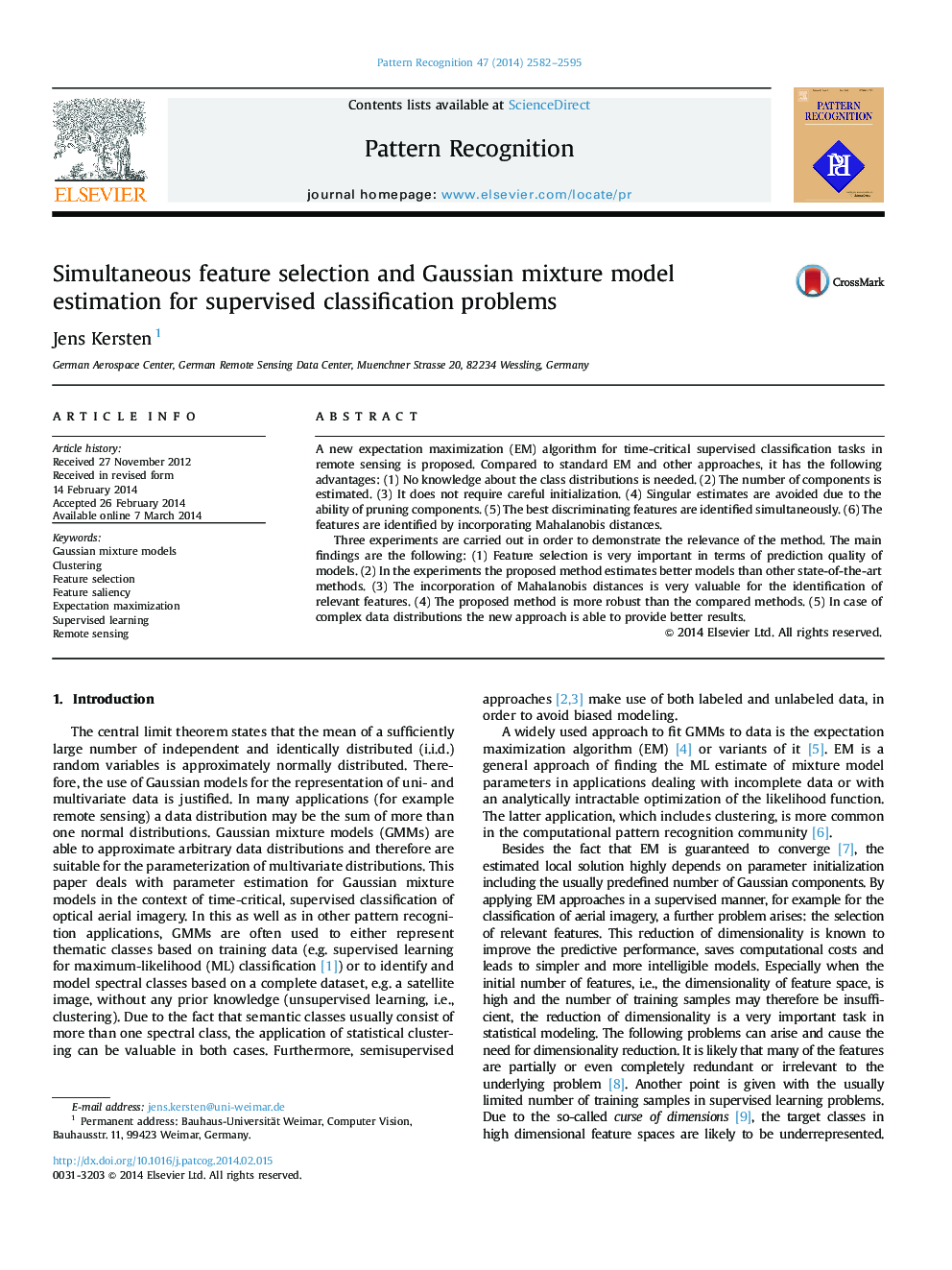
• A new EM algorithm for feature selection and model estimation is proposed.
• The algorithm is compared to other state-of-the-art approaches.
• Similar good or better results are obtained by the proposed method.
• Regarding time-critical applications and complex data, it outperforms other methods.
A new expectation maximization (EM) algorithm for time-critical supervised classification tasks in remote sensing is proposed. Compared to standard EM and other approaches, it has the following advantages: (1) No knowledge about the class distributions is needed. (2) The number of components is estimated. (3) It does not require careful initialization. (4) Singular estimates are avoided due to the ability of pruning components. (5) The best discriminating features are identified simultaneously. (6) The features are identified by incorporating Mahalanobis distances.Three experiments are carried out in order to demonstrate the relevance of the method. The main findings are the following: (1) Feature selection is very important in terms of prediction quality of models. (2) In the experiments the proposed method estimates better models than other state-of-the-art methods. (3) The incorporation of Mahalanobis distances is very valuable for the identification of relevant features. (4) The proposed method is more robust than the compared methods. (5) In case of complex data distributions the new approach is able to provide better results.
Journal: Pattern Recognition - Volume 47, Issue 8, August 2014, Pages 2582–2595