کد مقاله | کد نشریه | سال انتشار | مقاله انگلیسی | نسخه تمام متن |
---|---|---|---|---|
530774 | 869787 | 2014 | 7 صفحه PDF | دانلود رایگان |
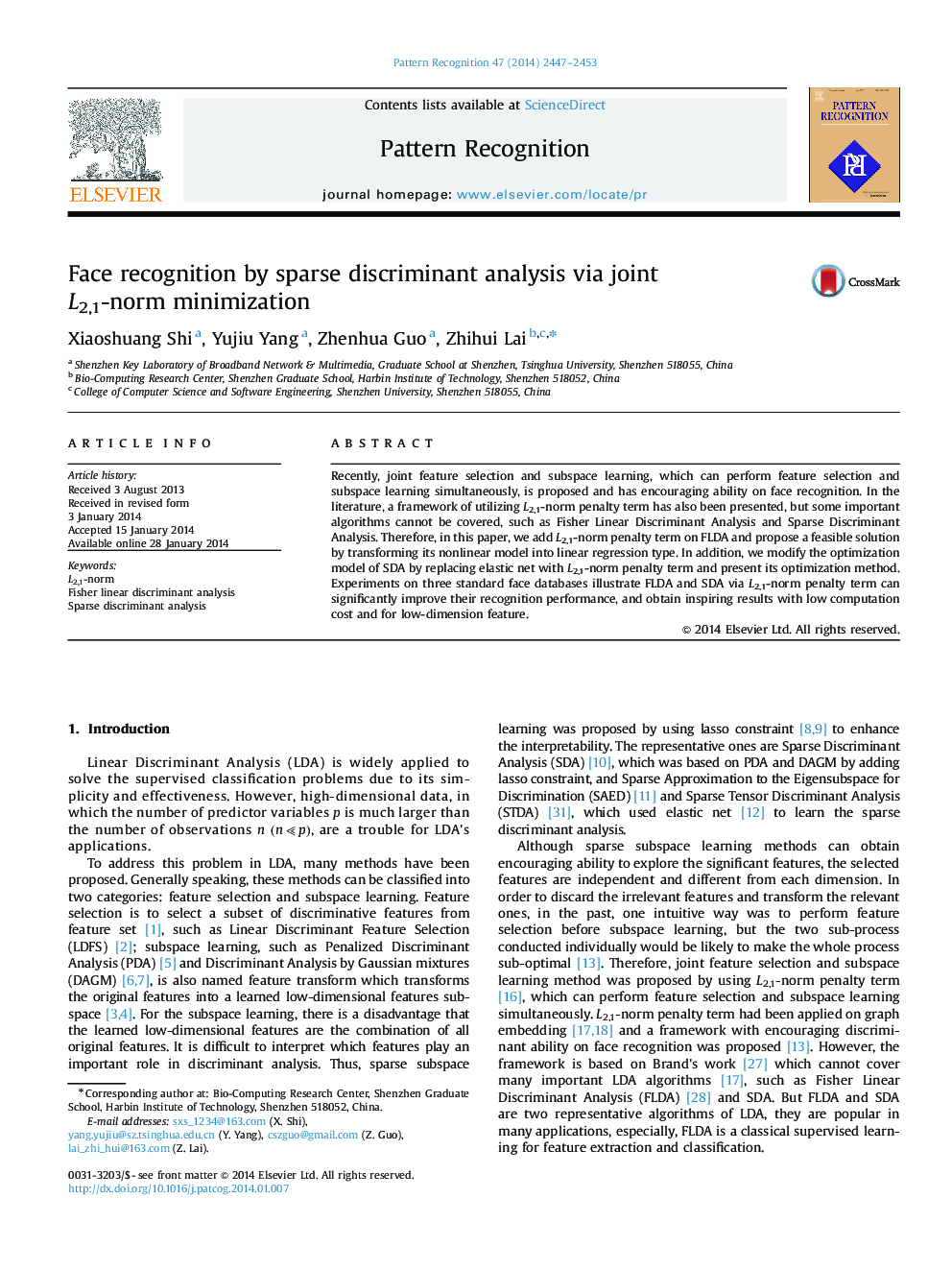
• Two novel Fisher Linear Discriminant Analysis (FLDA) methods based on L2,1-norm penalty are proposed.
• A modified Sparse Discriminant Analysis (SDA) based on L2,1-norm regularization is presented for jointly sparse feature extraction.
• L2,1-norm penalty on FLDA and SDA can significantly improve the recognition performance of FLDA and SDA, respectively.
Recently, joint feature selection and subspace learning, which can perform feature selection and subspace learning simultaneously, is proposed and has encouraging ability on face recognition. In the literature, a framework of utilizing L2,1-norm penalty term has also been presented, but some important algorithms cannot be covered, such as Fisher Linear Discriminant Analysis and Sparse Discriminant Analysis. Therefore, in this paper, we add L2,1-norm penalty term on FLDA and propose a feasible solution by transforming its nonlinear model into linear regression type. In addition, we modify the optimization model of SDA by replacing elastic net with L2,1-norm penalty term and present its optimization method. Experiments on three standard face databases illustrate FLDA and SDA via L2,1-norm penalty term can significantly improve their recognition performance, and obtain inspiring results with low computation cost and for low-dimension feature.
Journal: Pattern Recognition - Volume 47, Issue 7, July 2014, Pages 2447–2453