کد مقاله | کد نشریه | سال انتشار | مقاله انگلیسی | نسخه تمام متن |
---|---|---|---|---|
530860 | 869796 | 2011 | 19 صفحه PDF | دانلود رایگان |
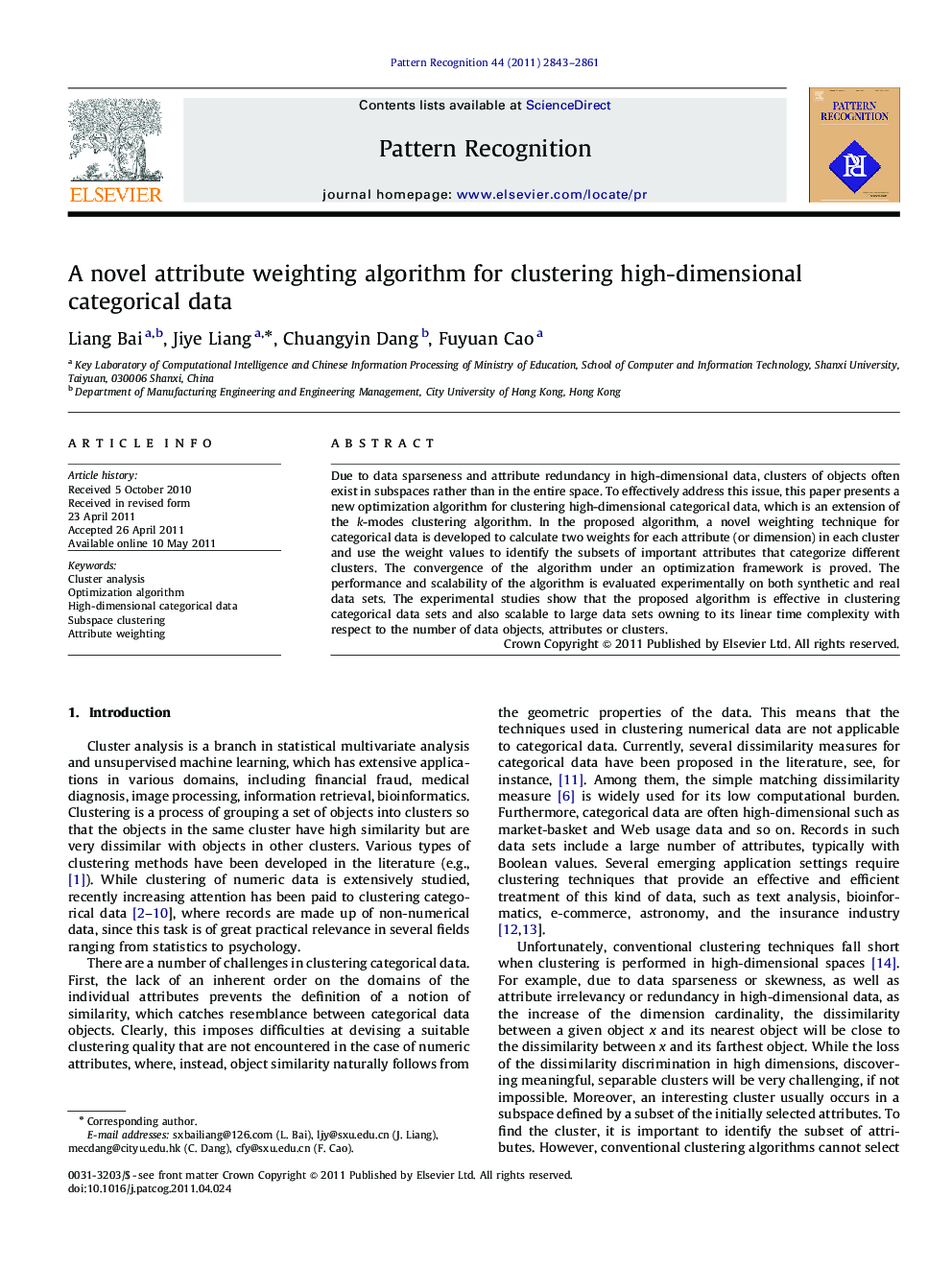
Due to data sparseness and attribute redundancy in high-dimensional data, clusters of objects often exist in subspaces rather than in the entire space. To effectively address this issue, this paper presents a new optimization algorithm for clustering high-dimensional categorical data, which is an extension of the k-modes clustering algorithm. In the proposed algorithm, a novel weighting technique for categorical data is developed to calculate two weights for each attribute (or dimension) in each cluster and use the weight values to identify the subsets of important attributes that categorize different clusters. The convergence of the algorithm under an optimization framework is proved. The performance and scalability of the algorithm is evaluated experimentally on both synthetic and real data sets. The experimental studies show that the proposed algorithm is effective in clustering categorical data sets and also scalable to large data sets owning to its linear time complexity with respect to the number of data objects, attributes or clusters.
► A new weighted dissimilarity measure is presented for categorical data.
► A mixed attribute weighting clustering algorithm is proposed.
► The updating formulas of the proposed algorithm are rigorously derived.
► The convergence of the proposed algorithm is proved.
► Experimental results show that the proposed algorithm is effective and efficient.
Journal: Pattern Recognition - Volume 44, Issue 12, December 2011, Pages 2843–2861