کد مقاله | کد نشریه | سال انتشار | مقاله انگلیسی | نسخه تمام متن |
---|---|---|---|---|
530933 | 869802 | 2013 | 11 صفحه PDF | دانلود رایگان |
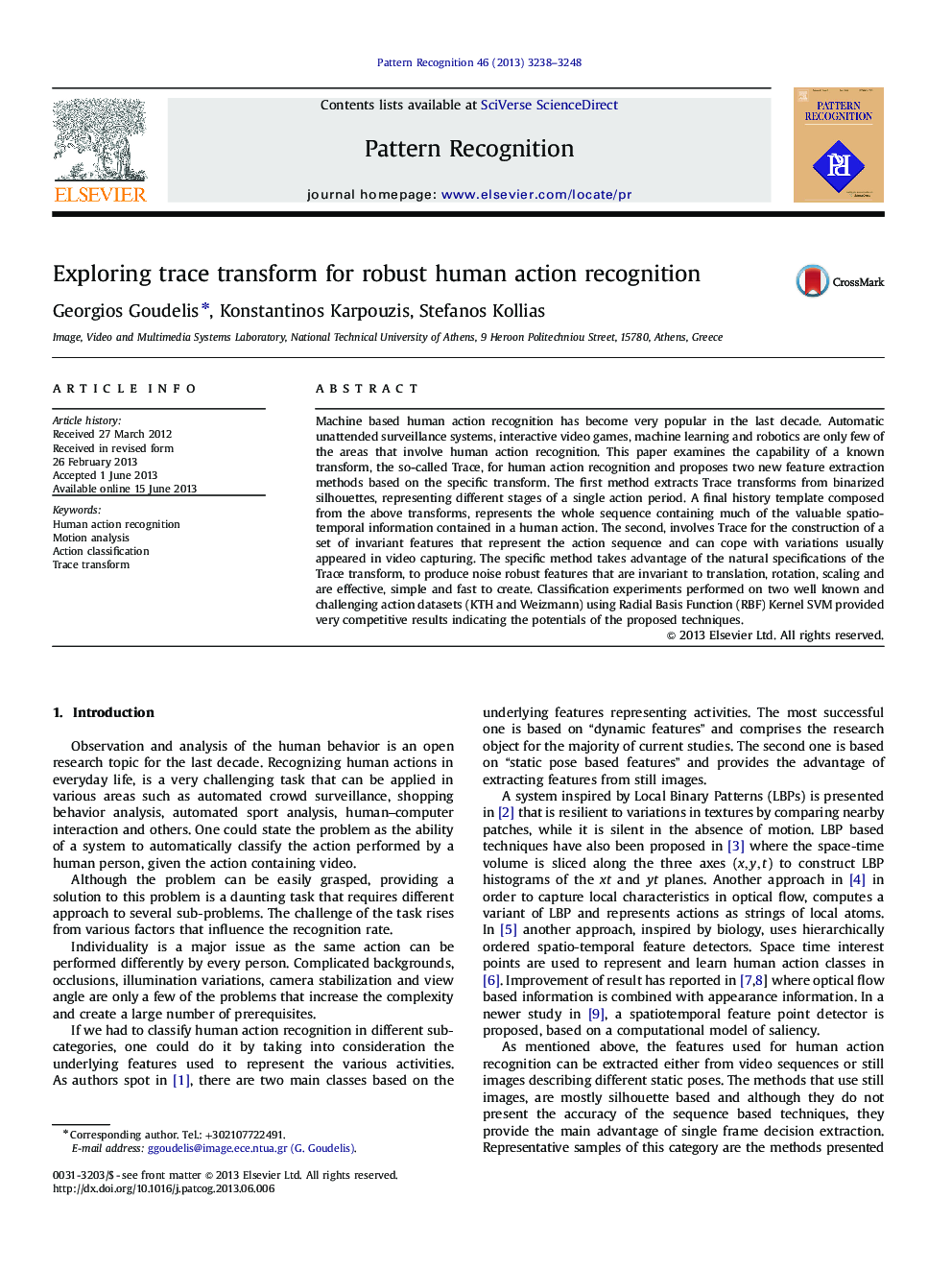
• We propose two novel feature extraction methods for Human Action Recognition (HAR).
• We examine for the first time, according to our knowledge, the capabilities of Trace transform for HAR.
• We introduce History Trace Templates (HTTs) and History Triple Features (HTFs) as a way to capture the spatiotemporal information of a human action.
• We employ different functionals of the Trace transform to calculate robust features and we introduce new features invariant to video caption variations.
• Experiments performed on noisy data without prior filtering, indicated great potentiality and very competitive results.
Machine based human action recognition has become very popular in the last decade. Automatic unattended surveillance systems, interactive video games, machine learning and robotics are only few of the areas that involve human action recognition. This paper examines the capability of a known transform, the so-called Trace, for human action recognition and proposes two new feature extraction methods based on the specific transform. The first method extracts Trace transforms from binarized silhouettes, representing different stages of a single action period. A final history template composed from the above transforms, represents the whole sequence containing much of the valuable spatio-temporal information contained in a human action. The second, involves Trace for the construction of a set of invariant features that represent the action sequence and can cope with variations usually appeared in video capturing. The specific method takes advantage of the natural specifications of the Trace transform, to produce noise robust features that are invariant to translation, rotation, scaling and are effective, simple and fast to create. Classification experiments performed on two well known and challenging action datasets (KTH and Weizmann) using Radial Basis Function (RBF) Kernel SVM provided very competitive results indicating the potentials of the proposed techniques.
Journal: Pattern Recognition - Volume 46, Issue 12, December 2013, Pages 3238–3248