کد مقاله | کد نشریه | سال انتشار | مقاله انگلیسی | نسخه تمام متن |
---|---|---|---|---|
531057 | 869807 | 2010 | 11 صفحه PDF | دانلود رایگان |
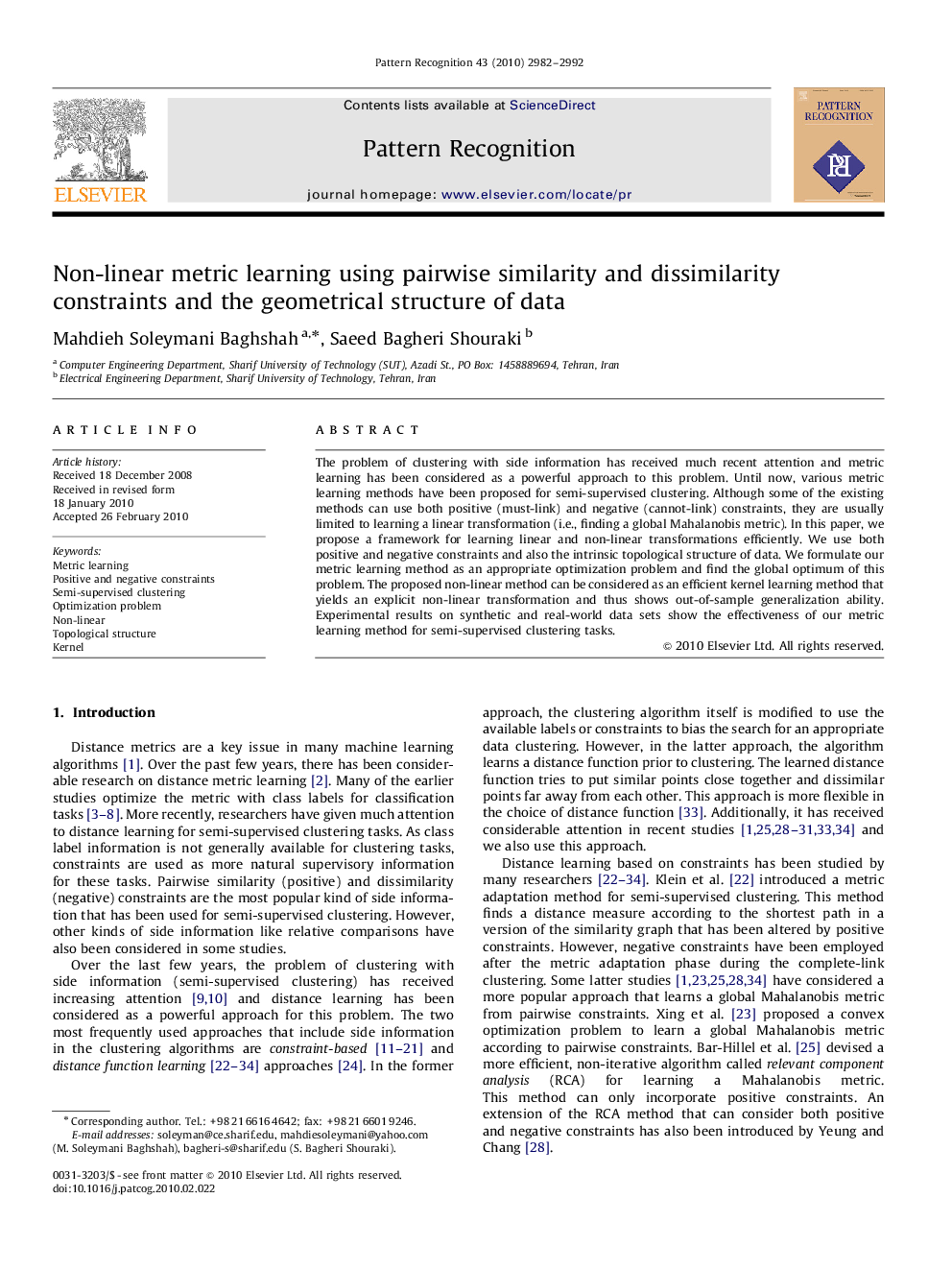
The problem of clustering with side information has received much recent attention and metric learning has been considered as a powerful approach to this problem. Until now, various metric learning methods have been proposed for semi-supervised clustering. Although some of the existing methods can use both positive (must-link) and negative (cannot-link) constraints, they are usually limited to learning a linear transformation (i.e., finding a global Mahalanobis metric). In this paper, we propose a framework for learning linear and non-linear transformations efficiently. We use both positive and negative constraints and also the intrinsic topological structure of data. We formulate our metric learning method as an appropriate optimization problem and find the global optimum of this problem. The proposed non-linear method can be considered as an efficient kernel learning method that yields an explicit non-linear transformation and thus shows out-of-sample generalization ability. Experimental results on synthetic and real-world data sets show the effectiveness of our metric learning method for semi-supervised clustering tasks.
Journal: Pattern Recognition - Volume 43, Issue 8, August 2010, Pages 2982–2992