کد مقاله | کد نشریه | سال انتشار | مقاله انگلیسی | نسخه تمام متن |
---|---|---|---|---|
531081 | 869808 | 2013 | 14 صفحه PDF | دانلود رایگان |
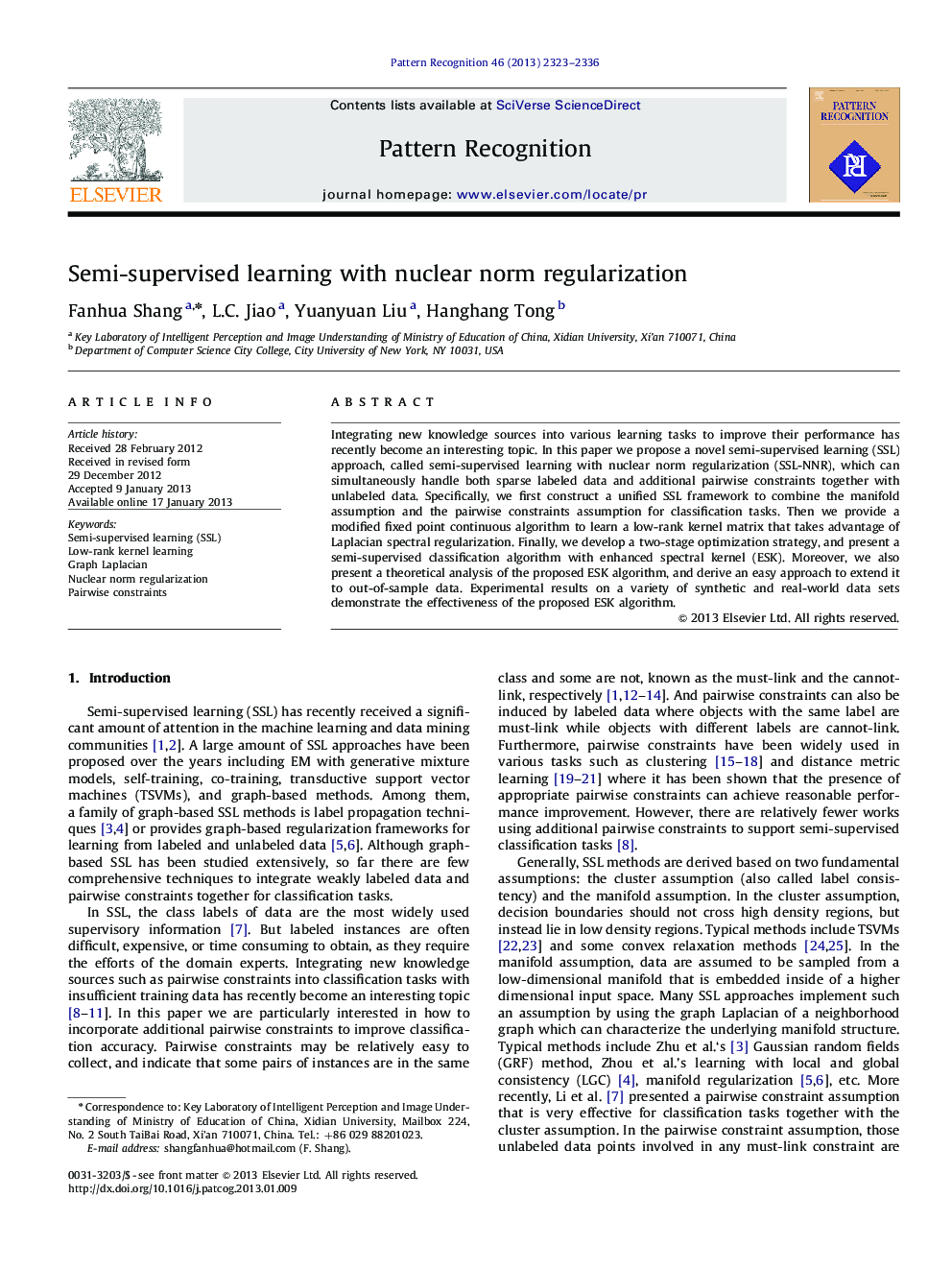
Integrating new knowledge sources into various learning tasks to improve their performance has recently become an interesting topic. In this paper we propose a novel semi-supervised learning (SSL) approach, called semi-supervised learning with nuclear norm regularization (SSL-NNR), which can simultaneously handle both sparse labeled data and additional pairwise constraints together with unlabeled data. Specifically, we first construct a unified SSL framework to combine the manifold assumption and the pairwise constraints assumption for classification tasks. Then we provide a modified fixed point continuous algorithm to learn a low-rank kernel matrix that takes advantage of Laplacian spectral regularization. Finally, we develop a two-stage optimization strategy, and present a semi-supervised classification algorithm with enhanced spectral kernel (ESK). Moreover, we also present a theoretical analysis of the proposed ESK algorithm, and derive an easy approach to extend it to out-of-sample data. Experimental results on a variety of synthetic and real-world data sets demonstrate the effectiveness of the proposed ESK algorithm.
► A unified SSL framework with mixed knowledge information (MKI) is constructed.
► MKI can handle labeled data and constraints together with unlabeled data.
► A modified fixed point continuous algorithm (MFPC) is presented.
► An SSL approach with enhanced spectral kernel (ESK) is proposed.
► Promising experimental results on a variety of data sets are provided.
Journal: Pattern Recognition - Volume 46, Issue 8, August 2013, Pages 2323–2336