کد مقاله | کد نشریه | سال انتشار | مقاله انگلیسی | نسخه تمام متن |
---|---|---|---|---|
531746 | 869870 | 2007 | 7 صفحه PDF | دانلود رایگان |
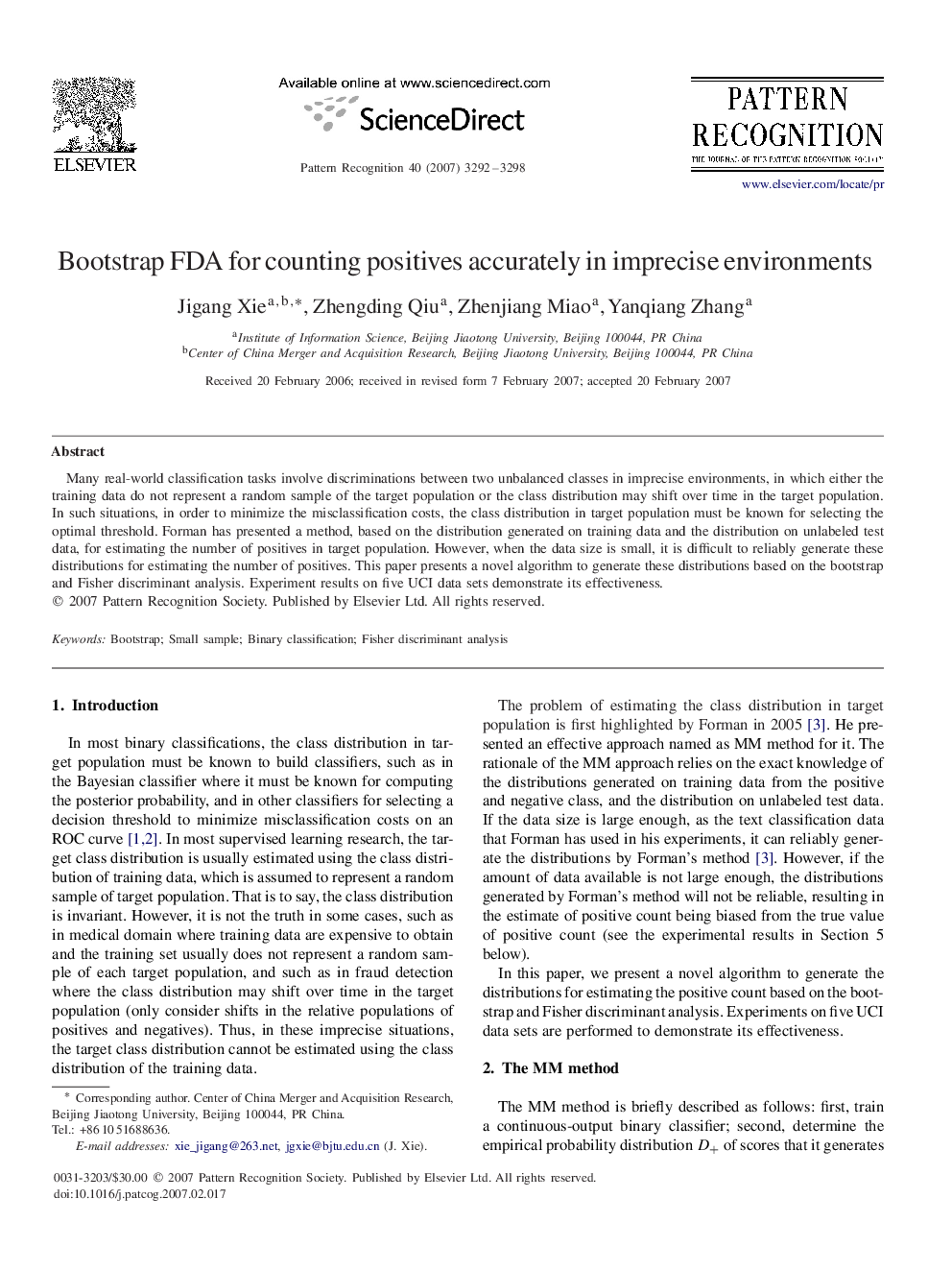
Many real-world classification tasks involve discriminations between two unbalanced classes in imprecise environments, in which either the training data do not represent a random sample of the target population or the class distribution may shift over time in the target population. In such situations, in order to minimize the misclassification costs, the class distribution in target population must be known for selecting the optimal threshold. Forman has presented a method, based on the distribution generated on training data and the distribution on unlabeled test data, for estimating the number of positives in target population. However, when the data size is small, it is difficult to reliably generate these distributions for estimating the number of positives. This paper presents a novel algorithm to generate these distributions based on the bootstrap and Fisher discriminant analysis. Experiment results on five UCI data sets demonstrate its effectiveness.
Journal: Pattern Recognition - Volume 40, Issue 11, November 2007, Pages 3292–3298