کد مقاله | کد نشریه | سال انتشار | مقاله انگلیسی | نسخه تمام متن |
---|---|---|---|---|
531792 | 869876 | 2016 | 13 صفحه PDF | دانلود رایگان |
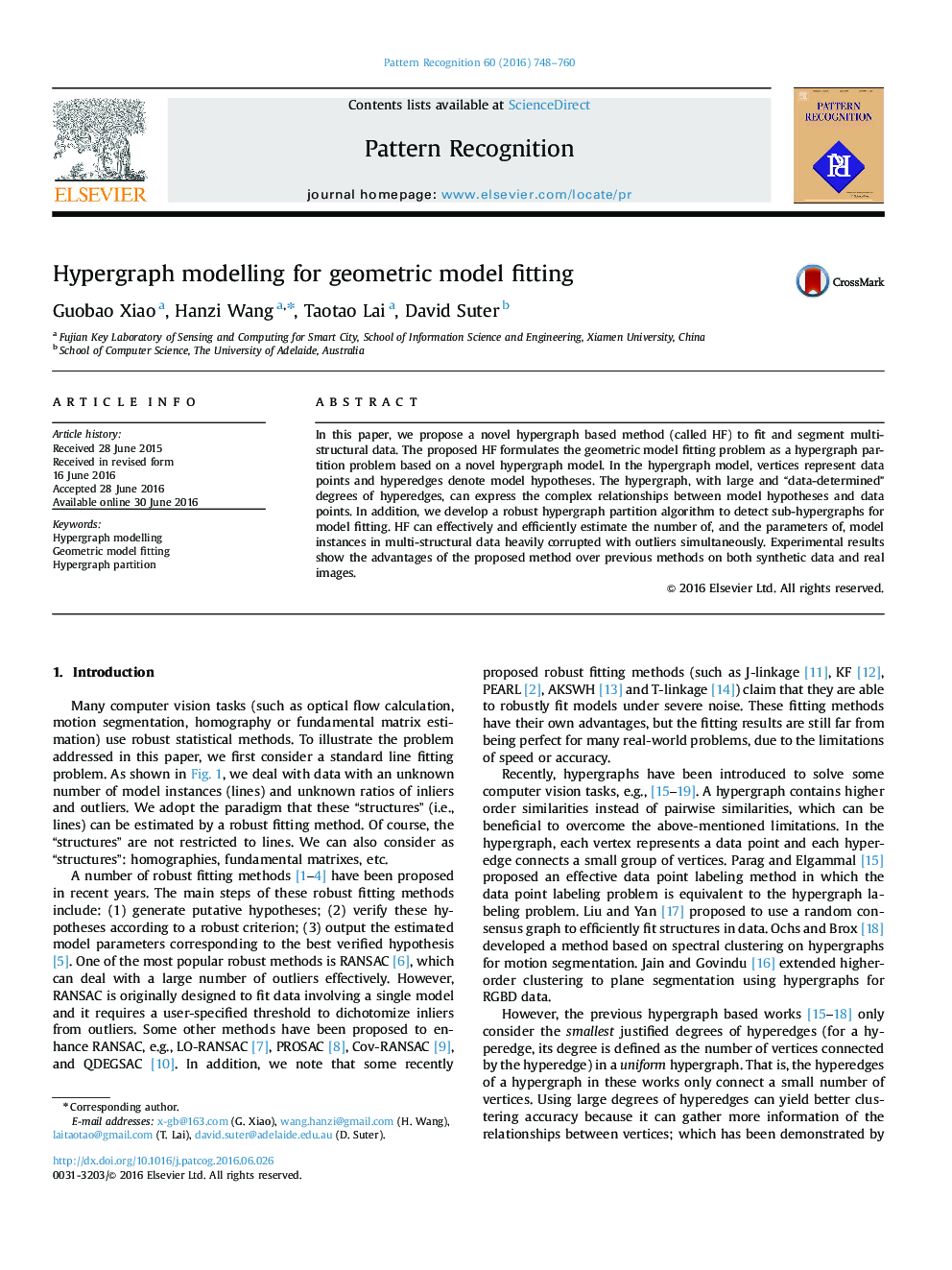
• We propose a novel hypergraph based method to fit and segment multi-structural data.
• The proposed method includes a hypergraph model with large degrees of hyperedges.
• The proposed method includes a robust hypergraph partition algorithm.
• Experimental results show that the proposed method is superior to some state-of-the-art fitting methods.
In this paper, we propose a novel hypergraph based method (called HF) to fit and segment multi-structural data. The proposed HF formulates the geometric model fitting problem as a hypergraph partition problem based on a novel hypergraph model. In the hypergraph model, vertices represent data points and hyperedges denote model hypotheses. The hypergraph, with large and “data-determined” degrees of hyperedges, can express the complex relationships between model hypotheses and data points. In addition, we develop a robust hypergraph partition algorithm to detect sub-hypergraphs for model fitting. HF can effectively and efficiently estimate the number of, and the parameters of, model instances in multi-structural data heavily corrupted with outliers simultaneously. Experimental results show the advantages of the proposed method over previous methods on both synthetic data and real images.
Journal: Pattern Recognition - Volume 60, December 2016, Pages 748–760