کد مقاله | کد نشریه | سال انتشار | مقاله انگلیسی | نسخه تمام متن |
---|---|---|---|---|
531811 | 869876 | 2016 | 11 صفحه PDF | دانلود رایگان |
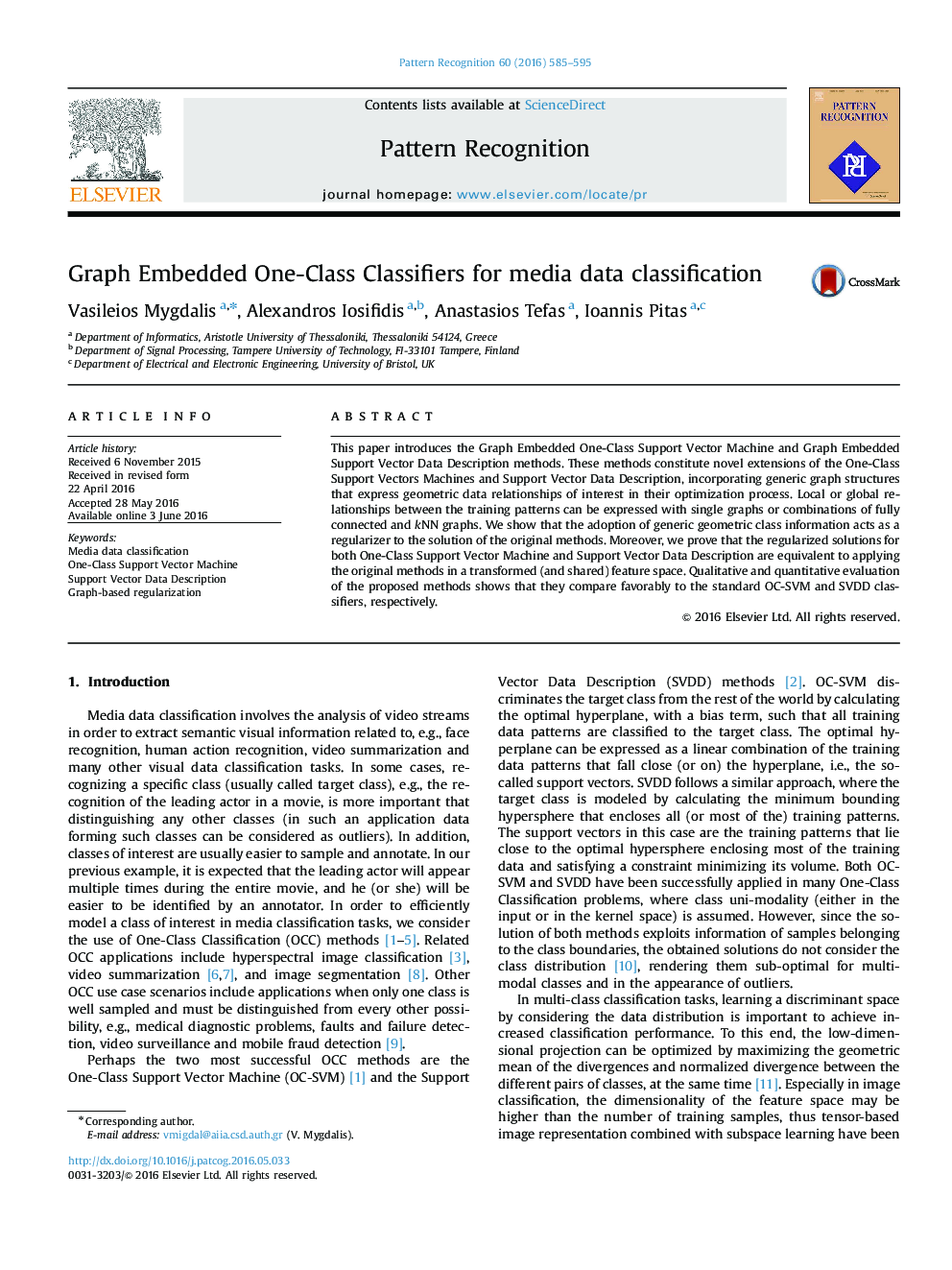
• Introducing the Graph-Embedded One-Class SVM and Graph Embedded SVDD methods.
• Exploiting data relationships in the optimization process.
• Adopting generic geometric class information acts as a regularizer.
• The regularized solutions for both proposed methods are equivalent.
• They compare favorably to the standard OC-SVM and SVDD classifiers.
This paper introduces the Graph Embedded One-Class Support Vector Machine and Graph Embedded Support Vector Data Description methods. These methods constitute novel extensions of the One-Class Support Vectors Machines and Support Vector Data Description, incorporating generic graph structures that express geometric data relationships of interest in their optimization process. Local or global relationships between the training patterns can be expressed with single graphs or combinations of fully connected and kNN graphs. We show that the adoption of generic geometric class information acts as a regularizer to the solution of the original methods. Moreover, we prove that the regularized solutions for both One-Class Support Vector Machine and Support Vector Data Description are equivalent to applying the original methods in a transformed (and shared) feature space. Qualitative and quantitative evaluation of the proposed methods shows that they compare favorably to the standard OC-SVM and SVDD classifiers, respectively.
Journal: Pattern Recognition - Volume 60, December 2016, Pages 585–595