کد مقاله | کد نشریه | سال انتشار | مقاله انگلیسی | نسخه تمام متن |
---|---|---|---|---|
532721 | 869986 | 2009 | 14 صفحه PDF | دانلود رایگان |
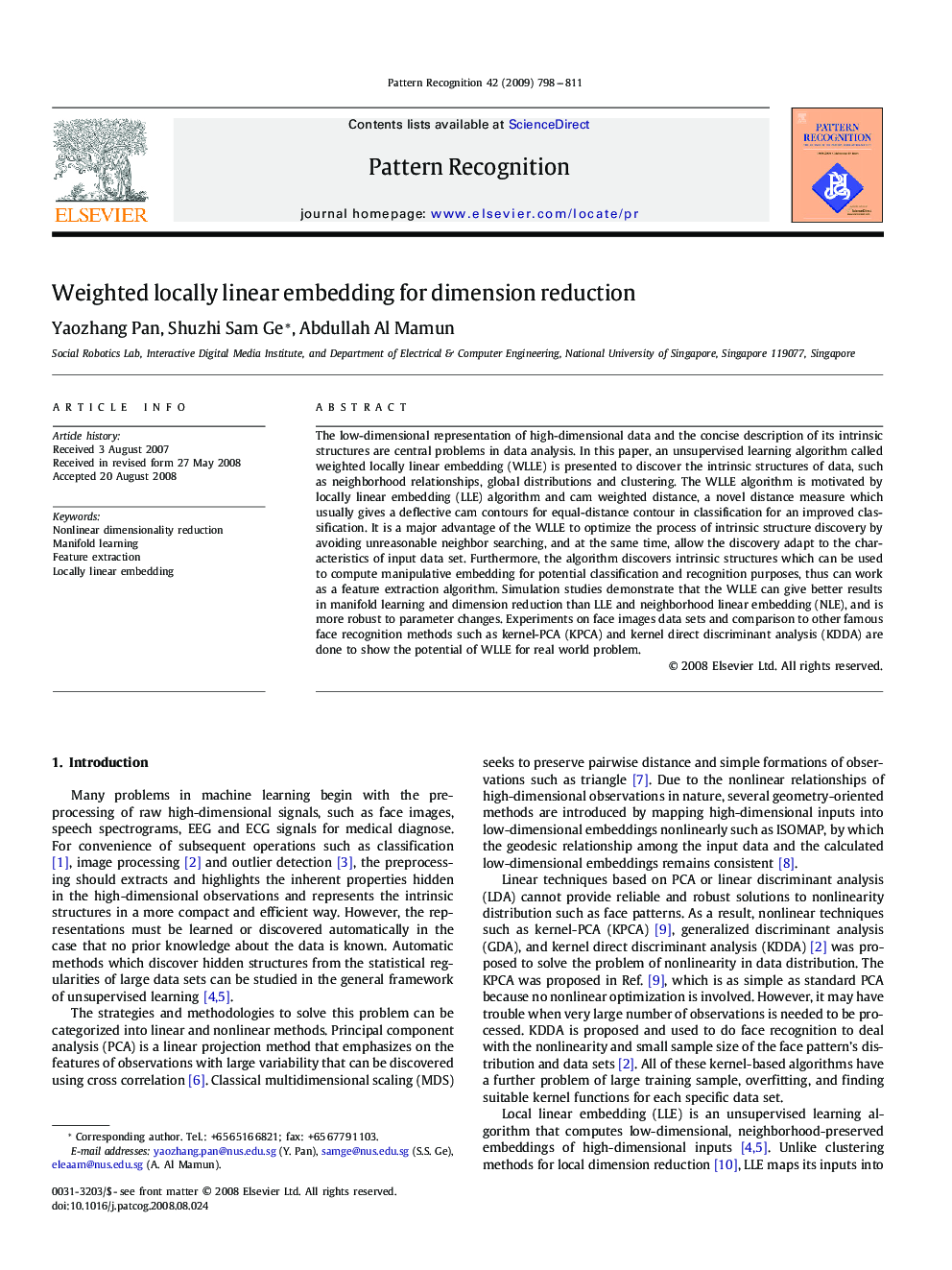
The low-dimensional representation of high-dimensional data and the concise description of its intrinsic structures are central problems in data analysis. In this paper, an unsupervised learning algorithm called weighted locally linear embedding (WLLE) is presented to discover the intrinsic structures of data, such as neighborhood relationships, global distributions and clustering. The WLLE algorithm is motivated by locally linear embedding (LLE) algorithm and cam weighted distance, a novel distance measure which usually gives a deflective cam contours for equal-distance contour in classification for an improved classification. It is a major advantage of the WLLE to optimize the process of intrinsic structure discovery by avoiding unreasonable neighbor searching, and at the same time, allow the discovery adapt to the characteristics of input data set. Furthermore, the algorithm discovers intrinsic structures which can be used to compute manipulative embedding for potential classification and recognition purposes, thus can work as a feature extraction algorithm. Simulation studies demonstrate that the WLLE can give better results in manifold learning and dimension reduction than LLE and neighborhood linear embedding (NLE), and is more robust to parameter changes. Experiments on face images data sets and comparison to other famous face recognition methods such as kernel-PCA (KPCA) and kernel direct discriminant analysis (KDDA) are done to show the potential of WLLE for real world problem.
Journal: Pattern Recognition - Volume 42, Issue 5, May 2009, Pages 798–811