کد مقاله | کد نشریه | سال انتشار | مقاله انگلیسی | نسخه تمام متن |
---|---|---|---|---|
533235 | 870083 | 2015 | 10 صفحه PDF | دانلود رایگان |
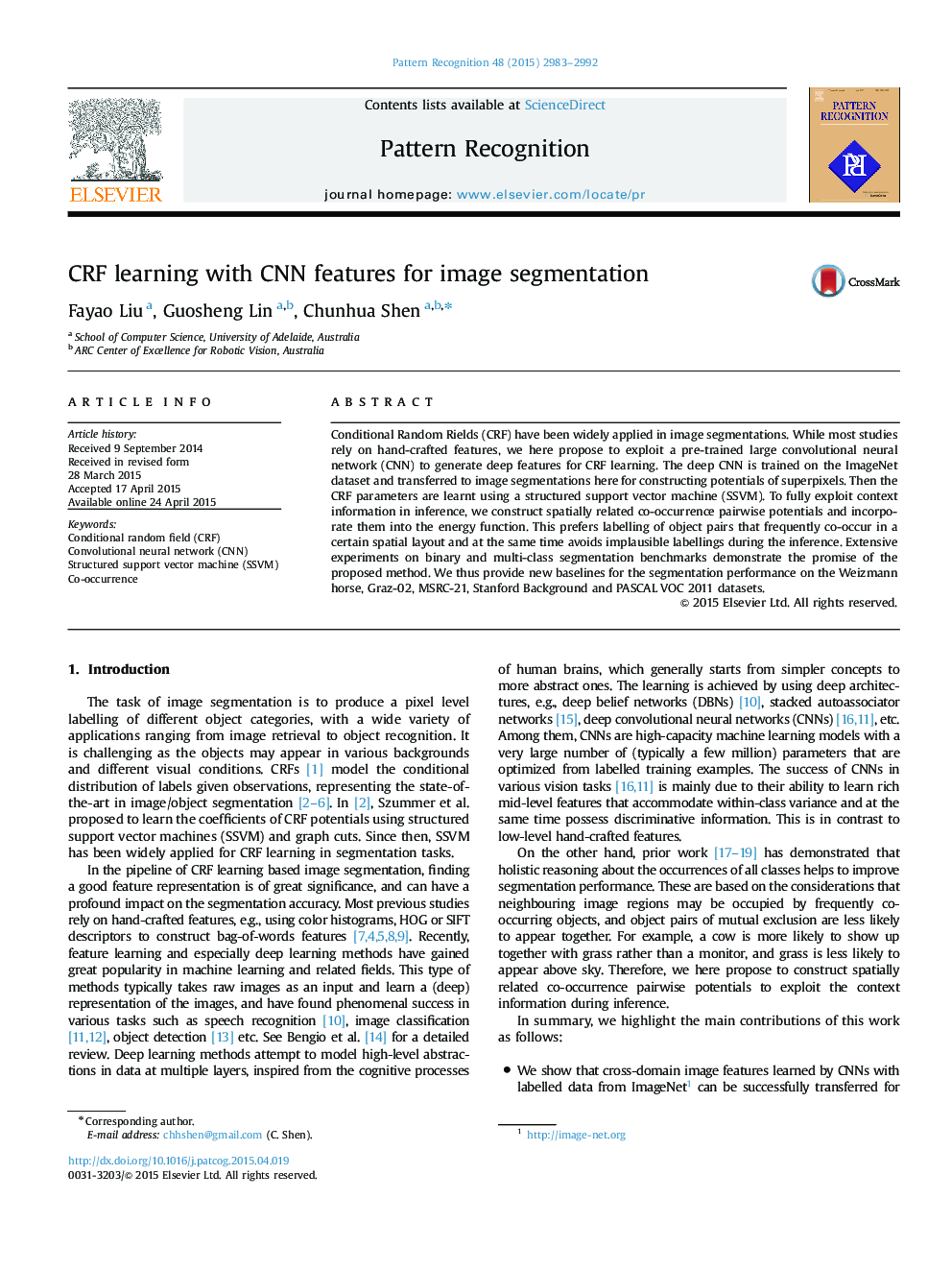
• A deep CNN pretrained on ImageNet generalizes well to various segmentation datasets.
• Deep features significantly outperform BoW and unsupervisd feature learning.
• Combining deep CNN features with CRF yields new state-of-the-art results.
• Incorporating spatial related co-occurrence potentials further improves the accuracy.
Conditional Random Rields (CRF) have been widely applied in image segmentations. While most studies rely on hand-crafted features, we here propose to exploit a pre-trained large convolutional neural network (CNN) to generate deep features for CRF learning. The deep CNN is trained on the ImageNet dataset and transferred to image segmentations here for constructing potentials of superpixels. Then the CRF parameters are learnt using a structured support vector machine (SSVM). To fully exploit context information in inference, we construct spatially related co-occurrence pairwise potentials and incorporate them into the energy function. This prefers labelling of object pairs that frequently co-occur in a certain spatial layout and at the same time avoids implausible labellings during the inference. Extensive experiments on binary and multi-class segmentation benchmarks demonstrate the promise of the proposed method. We thus provide new baselines for the segmentation performance on the Weizmann horse, Graz-02, MSRC-21, Stanford Background and PASCAL VOC 2011 datasets.
Journal: Pattern Recognition - Volume 48, Issue 10, October 2015, Pages 2983–2992