کد مقاله | کد نشریه | سال انتشار | مقاله انگلیسی | نسخه تمام متن |
---|---|---|---|---|
533248 | 870083 | 2015 | 10 صفحه PDF | دانلود رایگان |
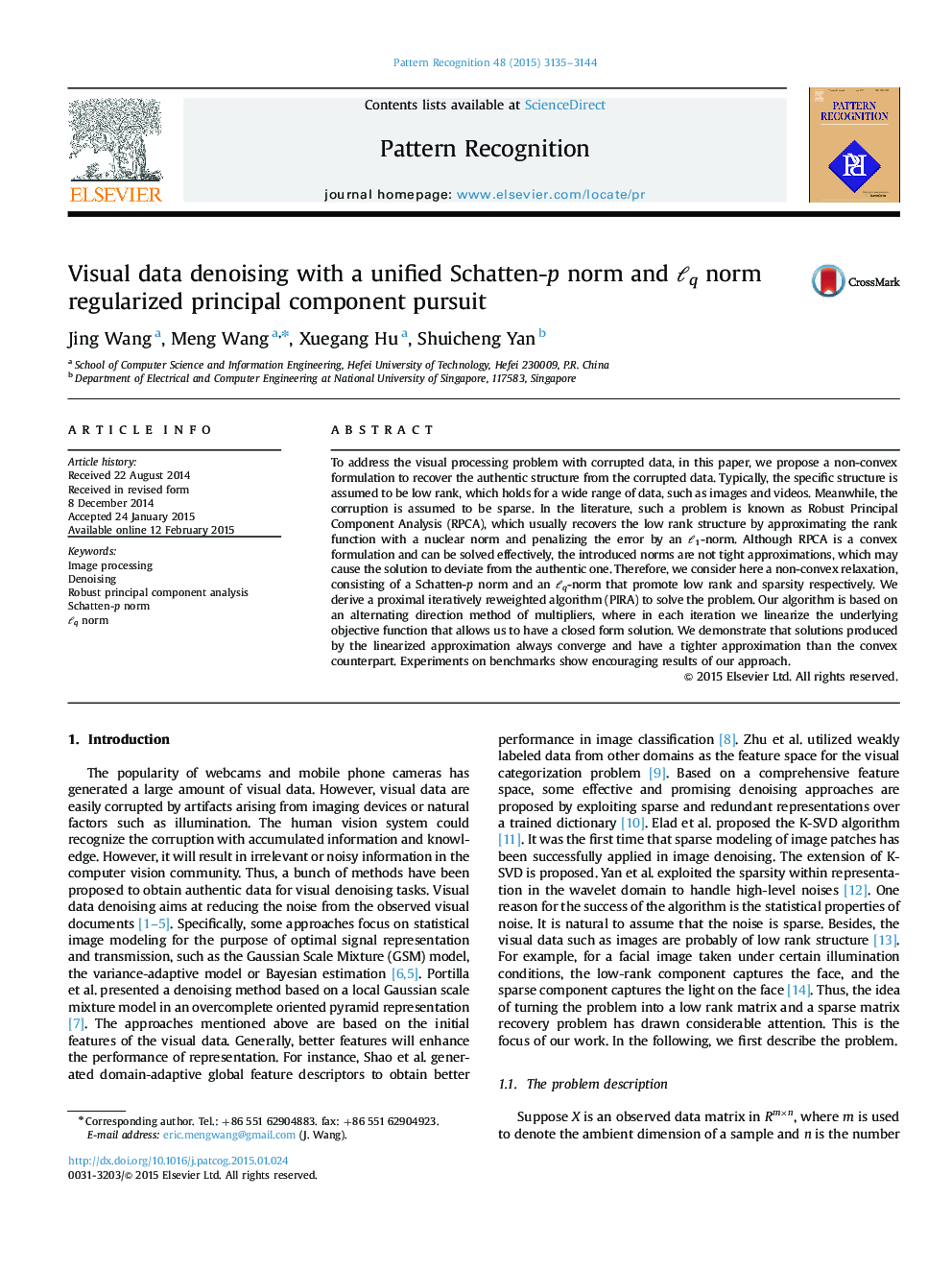
• We propose a (p; q-PCP) model for low-rank and sparse matrix recovery.
• A new Proximal Iteratively Reweighted Algorithm is presented to solve the problem.
• We show that our solutions can approximate the original problem.
• Experiments on a variety of datasets demonstrate the superiority of our approach.
To address the visual processing problem with corrupted data, in this paper, we propose a non-convex formulation to recover the authentic structure from the corrupted data. Typically, the specific structure is assumed to be low rank, which holds for a wide range of data, such as images and videos. Meanwhile, the corruption is assumed to be sparse. In the literature, such a problem is known as Robust Principal Component Analysis (RPCA), which usually recovers the low rank structure by approximating the rank function with a nuclear norm and penalizing the error by an ℓ1-norm. Although RPCA is a convex formulation and can be solved effectively, the introduced norms are not tight approximations, which may cause the solution to deviate from the authentic one. Therefore, we consider here a non-convex relaxation, consisting of a Schatten-p norm and an ℓq-norm that promote low rank and sparsity respectively. We derive a proximal iteratively reweighted algorithm (PIRA) to solve the problem. Our algorithm is based on an alternating direction method of multipliers, where in each iteration we linearize the underlying objective function that allows us to have a closed form solution. We demonstrate that solutions produced by the linearized approximation always converge and have a tighter approximation than the convex counterpart. Experiments on benchmarks show encouraging results of our approach.
Journal: Pattern Recognition - Volume 48, Issue 10, October 2015, Pages 3135–3144