کد مقاله | کد نشریه | سال انتشار | مقاله انگلیسی | نسخه تمام متن |
---|---|---|---|---|
533357 | 870109 | 2012 | 11 صفحه PDF | دانلود رایگان |
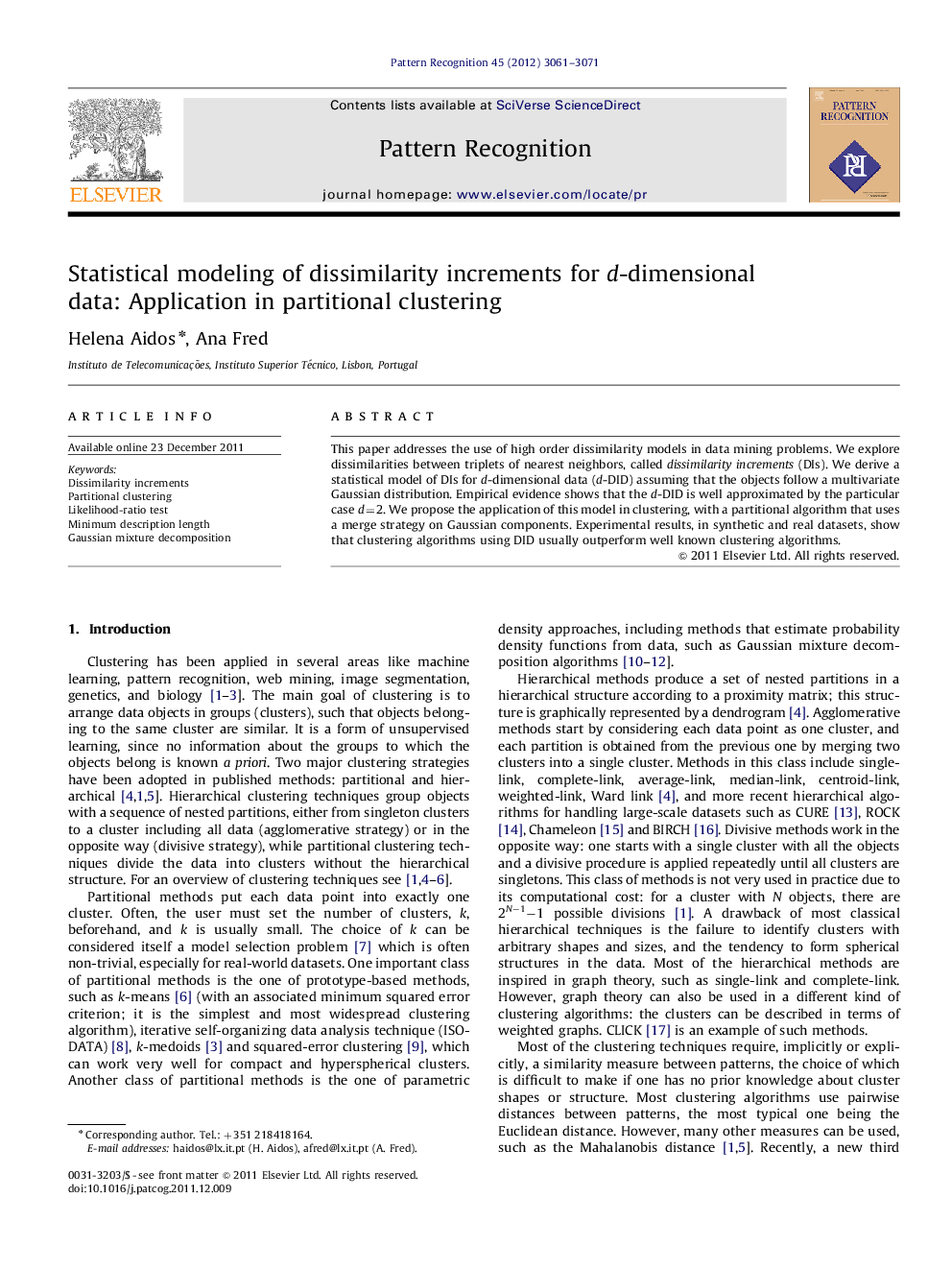
This paper addresses the use of high order dissimilarity models in data mining problems. We explore dissimilarities between triplets of nearest neighbors, called dissimilarity increments (DIs). We derive a statistical model of DIs for d-dimensional data (d-DID) assuming that the objects follow a multivariate Gaussian distribution. Empirical evidence shows that the d-DID is well approximated by the particular case d=2. We propose the application of this model in clustering, with a partitional algorithm that uses a merge strategy on Gaussian components. Experimental results, in synthetic and real datasets, show that clustering algorithms using DID usually outperform well known clustering algorithms.
► We derive a statistical model for dissimilarity increments in d-dimensional spaces.
► We propose GMDID: a Clustering algorithm using this model.
► We compare with other clustering techniques on multiple synthetic and real datasets.
► The results show GMDID's superior performance.
Journal: Pattern Recognition - Volume 45, Issue 9, September 2012, Pages 3061–3071