کد مقاله | کد نشریه | سال انتشار | مقاله انگلیسی | نسخه تمام متن |
---|---|---|---|---|
533979 | 870197 | 2016 | 8 صفحه PDF | دانلود رایگان |
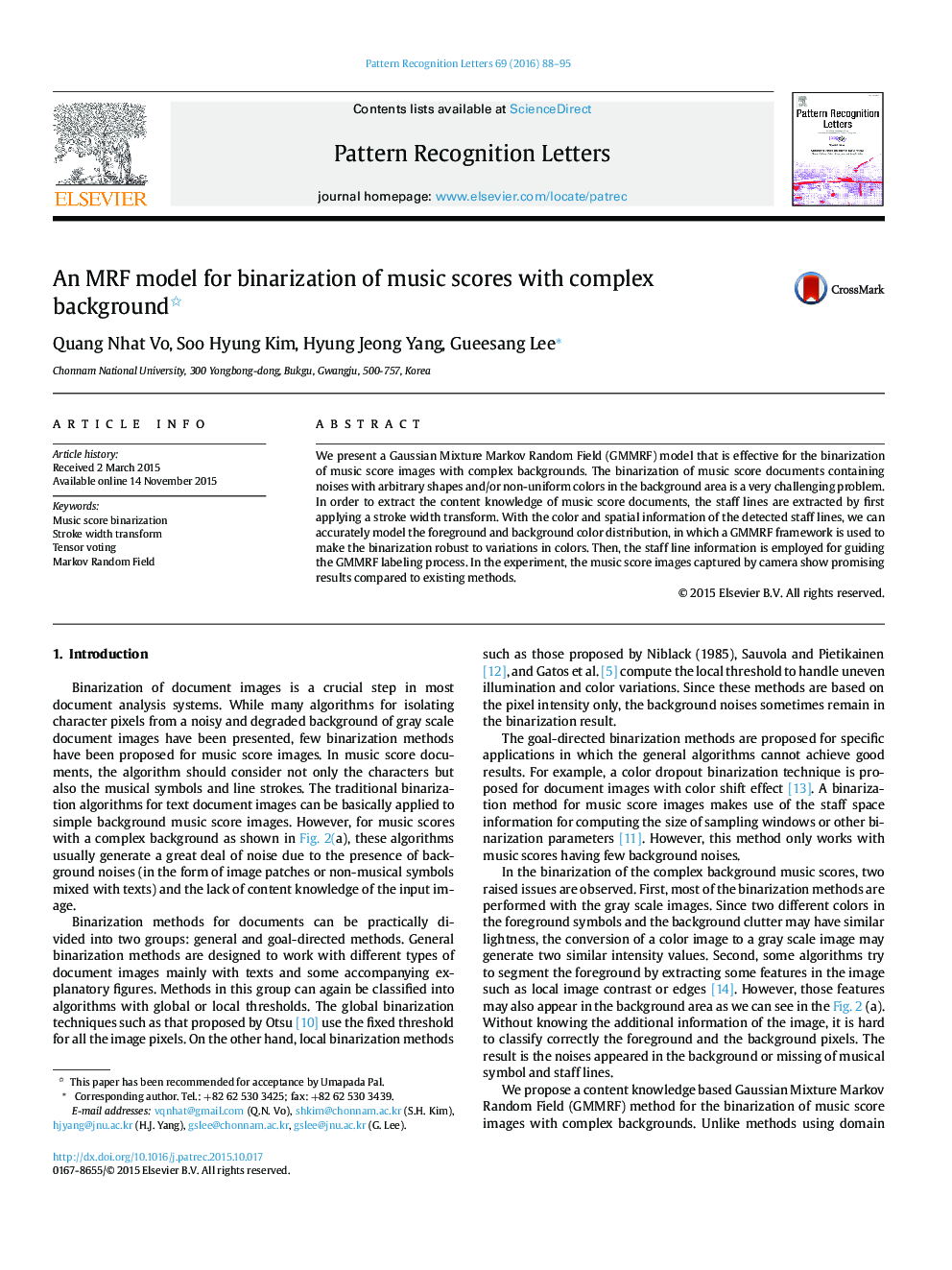
• Music score binarization in the presence of complex background is a challenging issue.
• Staff lines are used for the modeling of the color information of the foreground.
• The binarization is defined as the labeling process of the GMMRF model.
• Tensor voting based GMM is used for the color modeling.
We present a Gaussian Mixture Markov Random Field (GMMRF) model that is effective for the binarization of music score images with complex backgrounds. The binarization of music score documents containing noises with arbitrary shapes and/or non-uniform colors in the background area is a very challenging problem. In order to extract the content knowledge of music score documents, the staff lines are extracted by first applying a stroke width transform. With the color and spatial information of the detected staff lines, we can accurately model the foreground and background color distribution, in which a GMMRF framework is used to make the binarization robust to variations in colors. Then, the staff line information is employed for guiding the GMMRF labeling process. In the experiment, the music score images captured by camera show promising results compared to existing methods.
Journal: Pattern Recognition Letters - Volume 69, 1 January 2016, Pages 88–95