کد مقاله | کد نشریه | سال انتشار | مقاله انگلیسی | نسخه تمام متن |
---|---|---|---|---|
534230 | 870236 | 2011 | 6 صفحه PDF | دانلود رایگان |
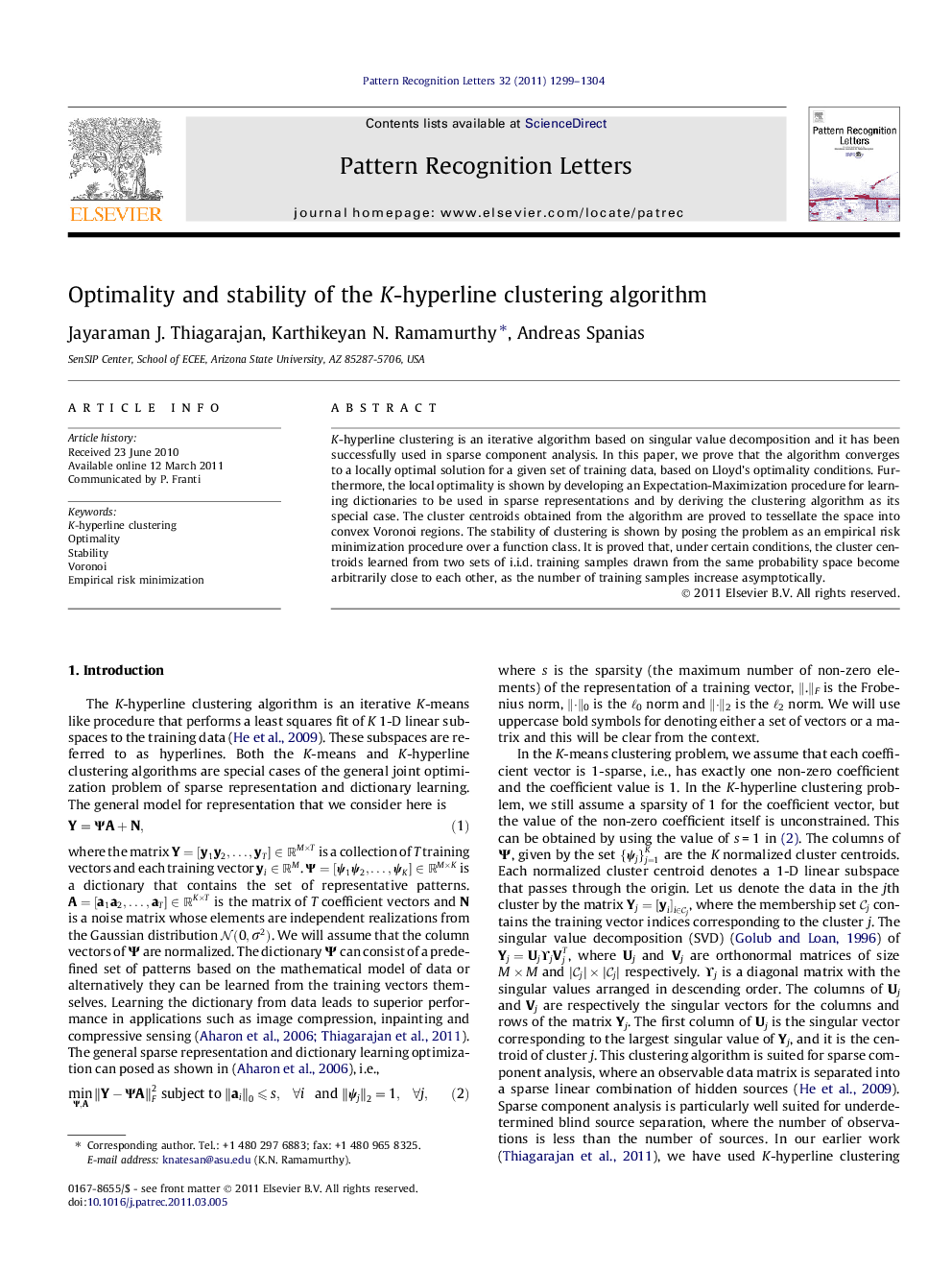
K-hyperline clustering is an iterative algorithm based on singular value decomposition and it has been successfully used in sparse component analysis. In this paper, we prove that the algorithm converges to a locally optimal solution for a given set of training data, based on Lloyd’s optimality conditions. Furthermore, the local optimality is shown by developing an Expectation-Maximization procedure for learning dictionaries to be used in sparse representations and by deriving the clustering algorithm as its special case. The cluster centroids obtained from the algorithm are proved to tessellate the space into convex Voronoi regions. The stability of clustering is shown by posing the problem as an empirical risk minimization procedure over a function class. It is proved that, under certain conditions, the cluster centroids learned from two sets of i.i.d. training samples drawn from the same probability space become arbitrarily close to each other, as the number of training samples increase asymptotically.
► The distortion function class of K-hyperline clustering is uniform Donsker
► The K-centroids of the clustering tesselate the space into 2 K Voronoi regions
► Local optimality proved using Lloyd’s conditions and an EM formulation
► Stability follows from uniform Donsker distortion class and geometry of clustering.
Journal: Pattern Recognition Letters - Volume 32, Issue 9, 1 July 2011, Pages 1299–1304