کد مقاله | کد نشریه | سال انتشار | مقاله انگلیسی | نسخه تمام متن |
---|---|---|---|---|
534456 | 870254 | 2010 | 7 صفحه PDF | دانلود رایگان |
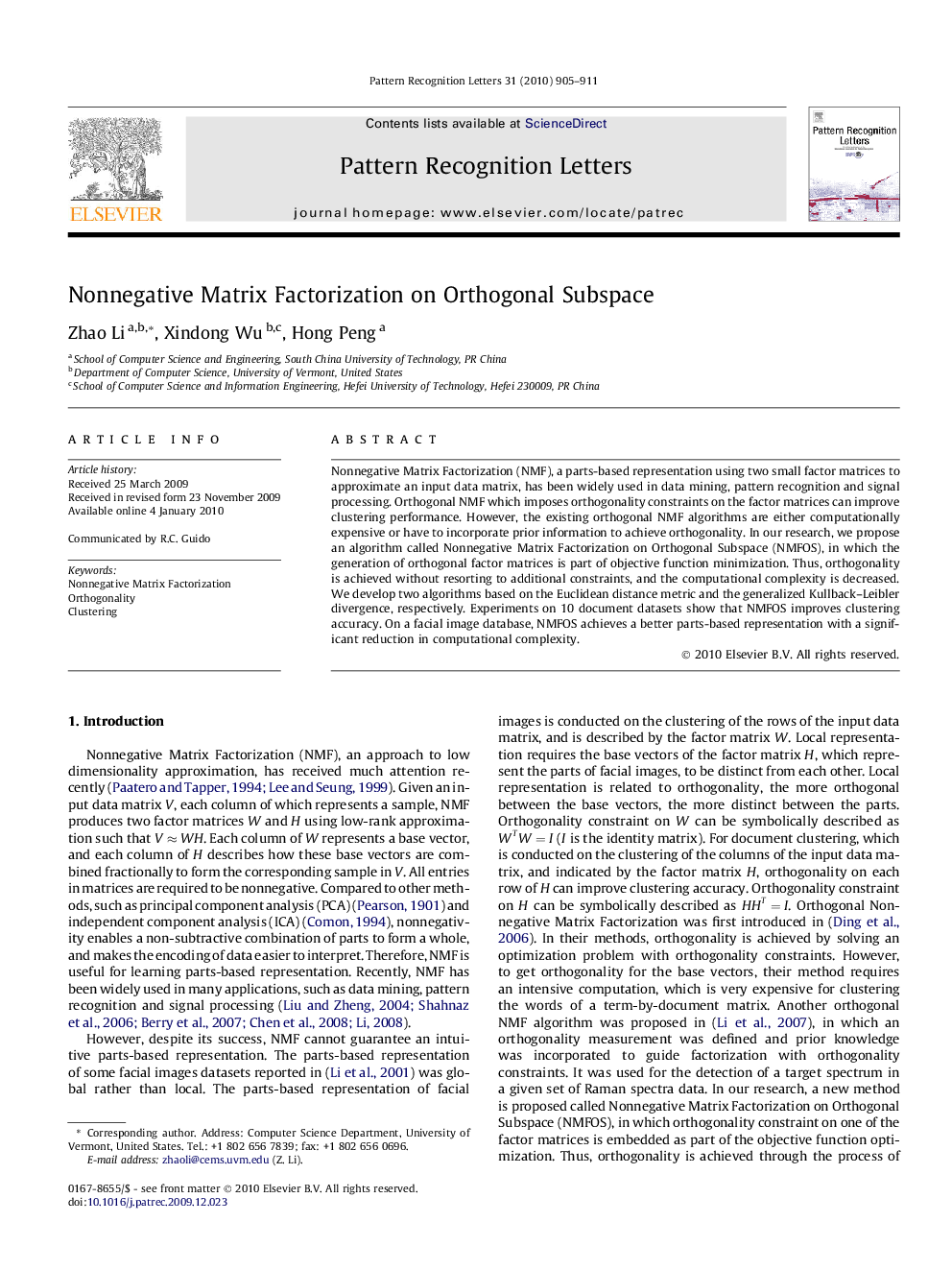
Nonnegative Matrix Factorization (NMF), a parts-based representation using two small factor matrices to approximate an input data matrix, has been widely used in data mining, pattern recognition and signal processing. Orthogonal NMF which imposes orthogonality constraints on the factor matrices can improve clustering performance. However, the existing orthogonal NMF algorithms are either computationally expensive or have to incorporate prior information to achieve orthogonality. In our research, we propose an algorithm called Nonnegative Matrix Factorization on Orthogonal Subspace (NMFOS), in which the generation of orthogonal factor matrices is part of objective function minimization. Thus, orthogonality is achieved without resorting to additional constraints, and the computational complexity is decreased. We develop two algorithms based on the Euclidean distance metric and the generalized Kullback–Leibler divergence, respectively. Experiments on 10 document datasets show that NMFOS improves clustering accuracy. On a facial image database, NMFOS achieves a better parts-based representation with a significant reduction in computational complexity.
Journal: Pattern Recognition Letters - Volume 31, Issue 9, 1 July 2010, Pages 905–911