کد مقاله | کد نشریه | سال انتشار | مقاله انگلیسی | نسخه تمام متن |
---|---|---|---|---|
534526 | 870262 | 2014 | 9 صفحه PDF | دانلود رایگان |
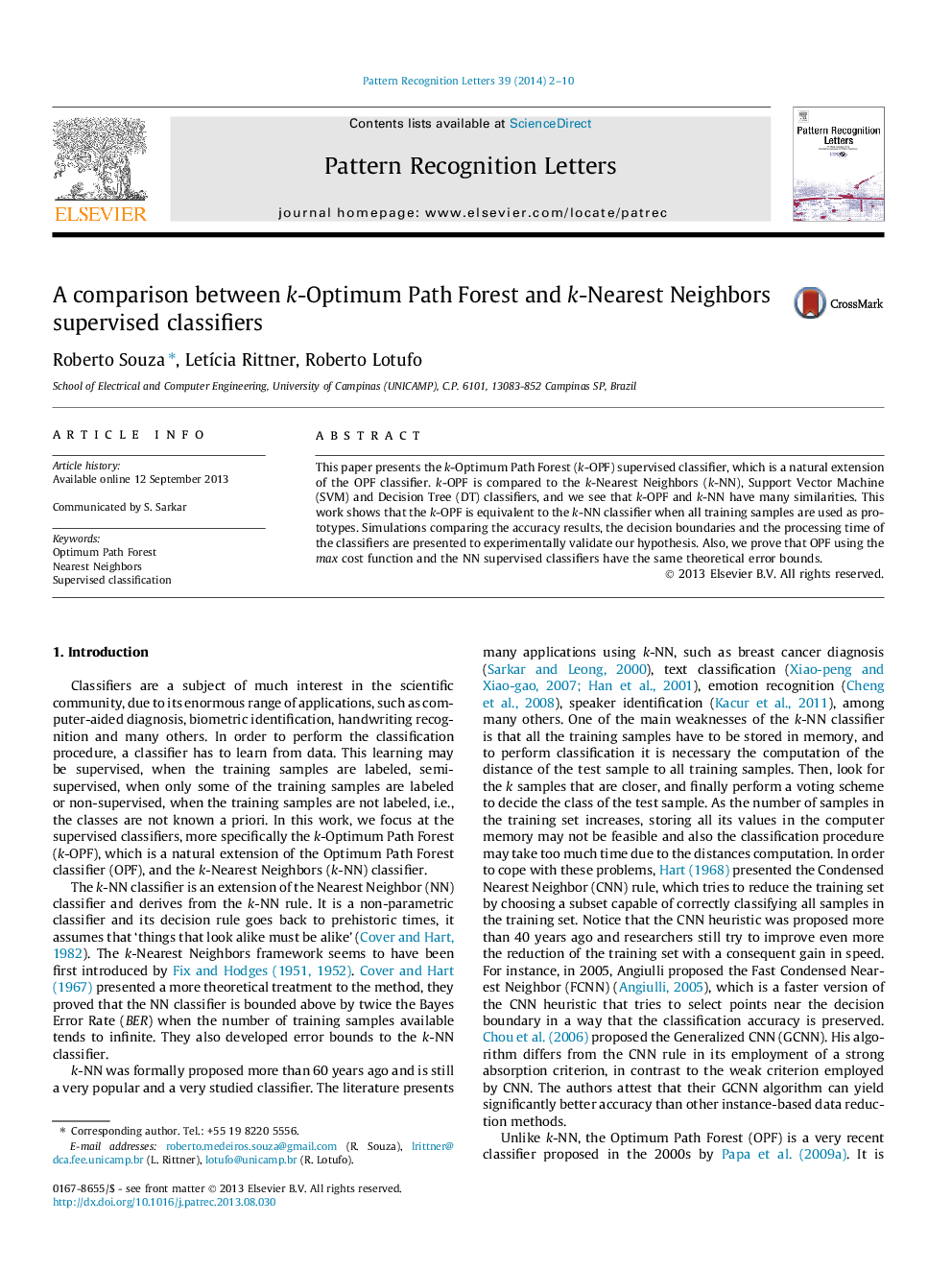
• Definition of the k-Optimum Path Forest (k-OPF) classifier.
• k-OPF is equivalent to k-NN when all training samples are used as prototypes.
• Definition of the k-OPF cost ordering property.
• Simulations comparing k-OPF, k-NN, SVM and DT classifiers.
• k-OPF using the costs ordering property is usually faster than k-NN during prediction.
• Proof that OPF and NN theoretical error bounds are the same.
This paper presents the k-Optimum Path Forest (k-OPF) supervised classifier, which is a natural extension of the OPF classifier. k-OPF is compared to the k-Nearest Neighbors (k-NN), Support Vector Machine (SVM) and Decision Tree (DT) classifiers, and we see that k-OPF and k-NN have many similarities. This work shows that the k-OPF is equivalent to the k-NN classifier when all training samples are used as prototypes. Simulations comparing the accuracy results, the decision boundaries and the processing time of the classifiers are presented to experimentally validate our hypothesis. Also, we prove that OPF using the max cost function and the NN supervised classifiers have the same theoretical error bounds.
Journal: Pattern Recognition Letters - Volume 39, 1 April 2014, Pages 2–10