کد مقاله | کد نشریه | سال انتشار | مقاله انگلیسی | نسخه تمام متن |
---|---|---|---|---|
534714 | 870283 | 2012 | 11 صفحه PDF | دانلود رایگان |
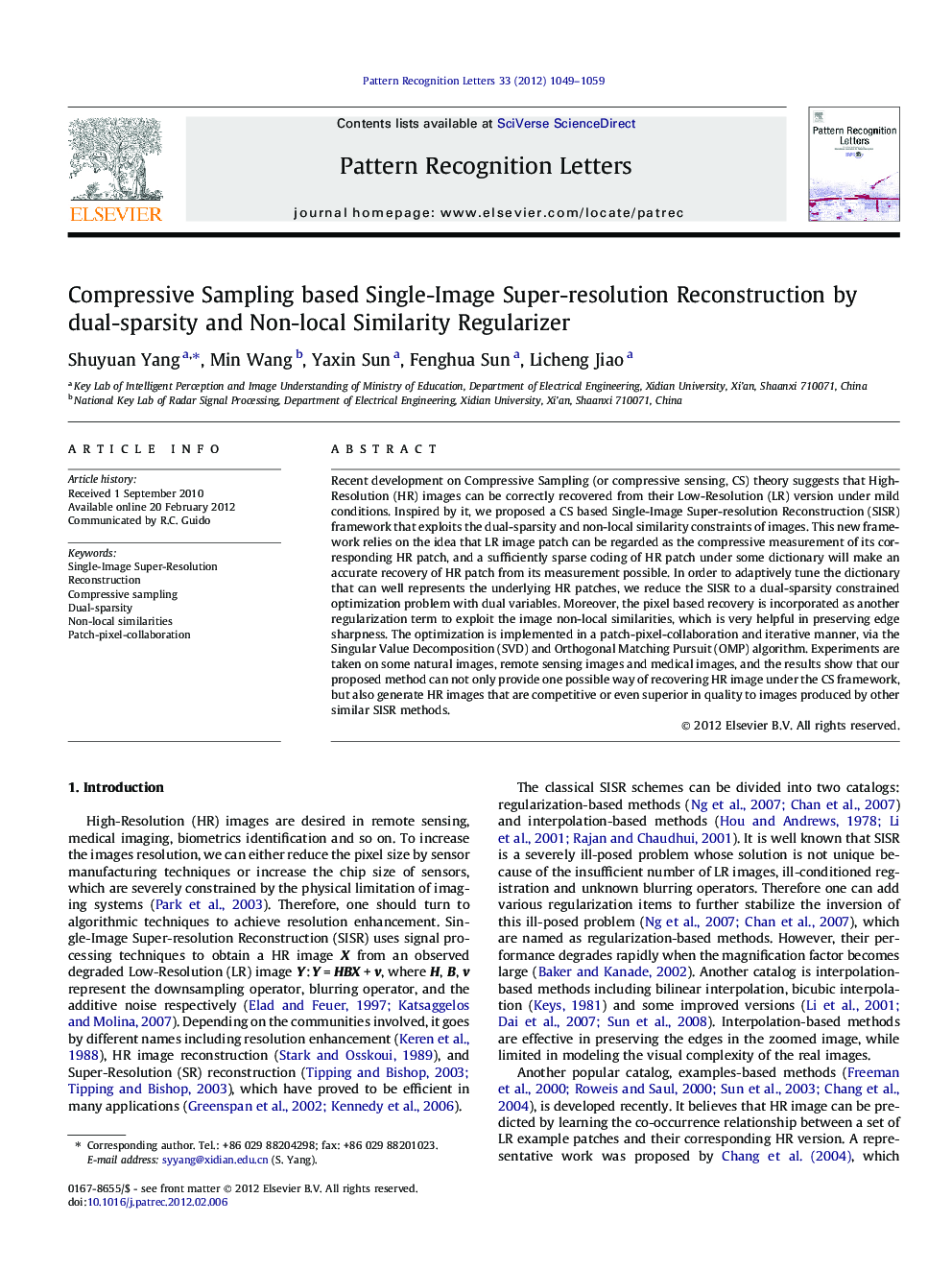
Recent development on Compressive Sampling (or compressive sensing, CS) theory suggests that High-Resolution (HR) images can be correctly recovered from their Low-Resolution (LR) version under mild conditions. Inspired by it, we proposed a CS based Single-Image Super-resolution Reconstruction (SISR) framework that exploits the dual-sparsity and non-local similarity constraints of images. This new framework relies on the idea that LR image patch can be regarded as the compressive measurement of its corresponding HR patch, and a sufficiently sparse coding of HR patch under some dictionary will make an accurate recovery of HR patch from its measurement possible. In order to adaptively tune the dictionary that can well represents the underlying HR patches, we reduce the SISR to a dual-sparsity constrained optimization problem with dual variables. Moreover, the pixel based recovery is incorporated as another regularization term to exploit the image non-local similarities, which is very helpful in preserving edge sharpness. The optimization is implemented in a patch-pixel-collaboration and iterative manner, via the Singular Value Decomposition (SVD) and Orthogonal Matching Pursuit (OMP) algorithm. Experiments are taken on some natural images, remote sensing images and medical images, and the results show that our proposed method can not only provide one possible way of recovering HR image under the CS framework, but also generate HR images that are competitive or even superior in quality to images produced by other similar SISR methods.
► Compressive sampling.
► Dual-sparsity.
► Non-local similarities.
► Patch-pixel-collaboration.
Journal: Pattern Recognition Letters - Volume 33, Issue 9, 1 July 2012, Pages 1049–1059