کد مقاله | کد نشریه | سال انتشار | مقاله انگلیسی | نسخه تمام متن |
---|---|---|---|---|
534857 | 870297 | 2011 | 8 صفحه PDF | دانلود رایگان |
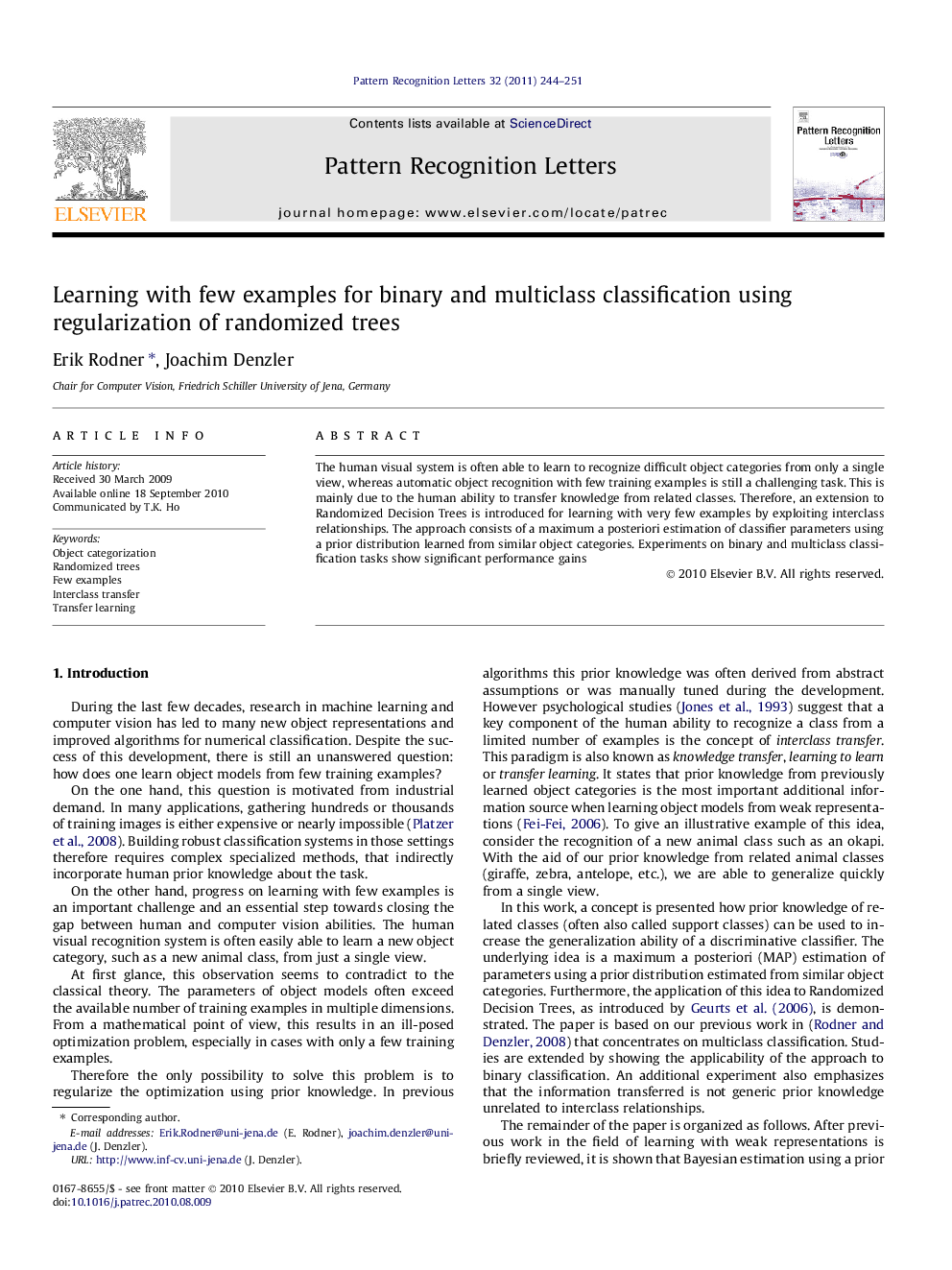
The human visual system is often able to learn to recognize difficult object categories from only a single view, whereas automatic object recognition with few training examples is still a challenging task. This is mainly due to the human ability to transfer knowledge from related classes. Therefore, an extension to Randomized Decision Trees is introduced for learning with very few examples by exploiting interclass relationships. The approach consists of a maximum a posteriori estimation of classifier parameters using a prior distribution learned from similar object categories. Experiments on binary and multiclass classification tasks show significant performance gains
Research highlights
► Randomized Decision Trees can be extended to cope with few training examples.
► MAP estimation of leaf probabilities enables to incorporate prior information.
► A contrained Gaussian distribution can be used to represent prior knowledge.
► Binary and multiclass image categorization benefits from regularized trees.
Journal: Pattern Recognition Letters - Volume 32, Issue 2, 15 January 2011, Pages 244–251