کد مقاله | کد نشریه | سال انتشار | مقاله انگلیسی | نسخه تمام متن |
---|---|---|---|---|
535602 | 870357 | 2005 | 11 صفحه PDF | دانلود رایگان |
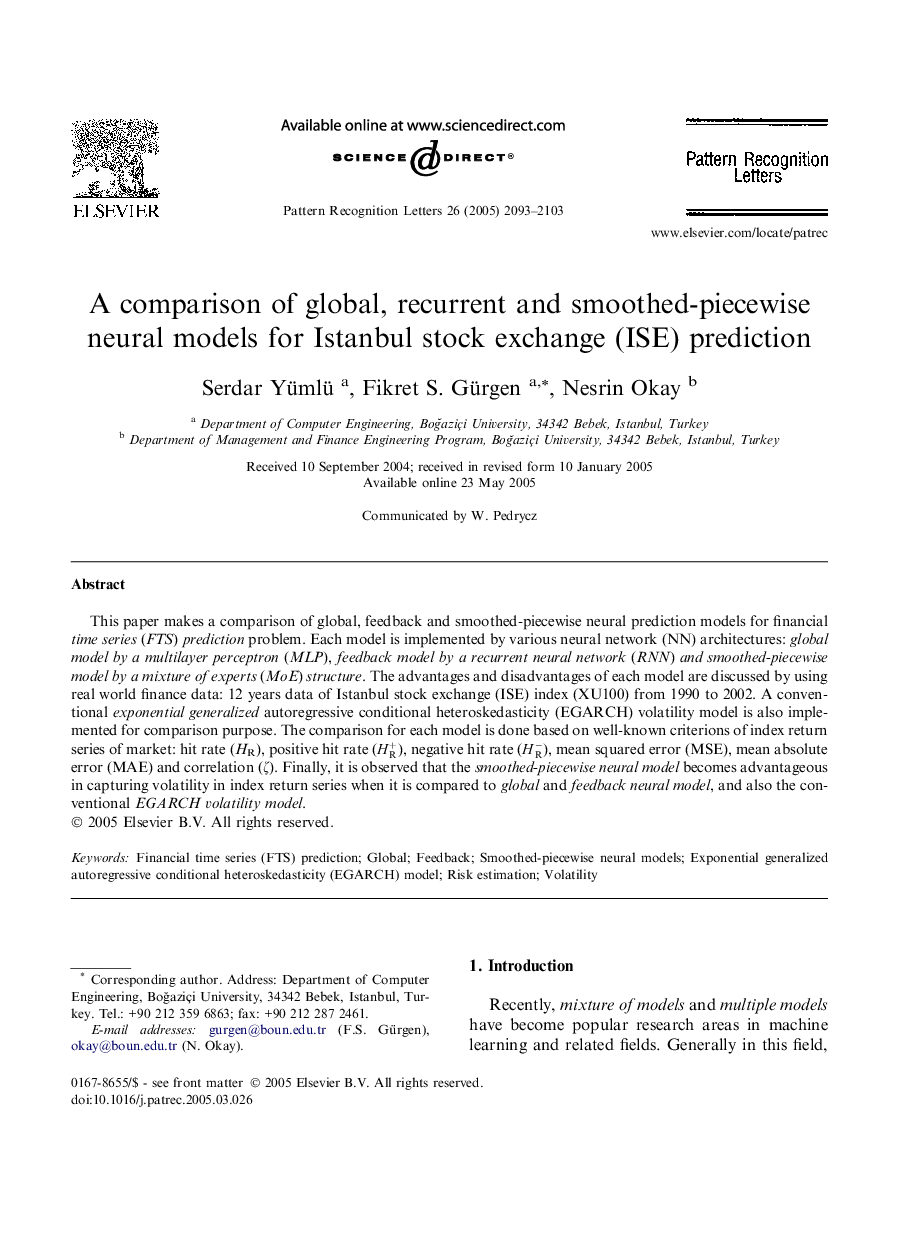
This paper makes a comparison of global, feedback and smoothed-piecewise neural prediction models for financial time series (FTS) prediction problem. Each model is implemented by various neural network (NN) architectures: global model by a multilayer perceptron (MLP), feedback model by a recurrent neural network (RNN) and smoothed-piecewise model by a mixture of experts (MoE) structure. The advantages and disadvantages of each model are discussed by using real world finance data: 12 years data of Istanbul stock exchange (ISE) index (XU100) from 1990 to 2002. A conventional exponential generalized autoregressive conditional heteroskedasticity (EGARCH) volatility model is also implemented for comparison purpose. The comparison for each model is done based on well-known criterions of index return series of market: hit rate (HR), positive hit rate (HR+), negative hit rate (HR-), mean squared error (MSE), mean absolute error (MAE) and correlation (ζ). Finally, it is observed that the smoothed-piecewise neural model becomes advantageous in capturing volatility in index return series when it is compared to global and feedback neural model, and also the conventional EGARCH volatility model.
Journal: Pattern Recognition Letters - Volume 26, Issue 13, 1 October 2005, Pages 2093–2103