کد مقاله | کد نشریه | سال انتشار | مقاله انگلیسی | نسخه تمام متن |
---|---|---|---|---|
535752 | 870374 | 2013 | 7 صفحه PDF | دانلود رایگان |
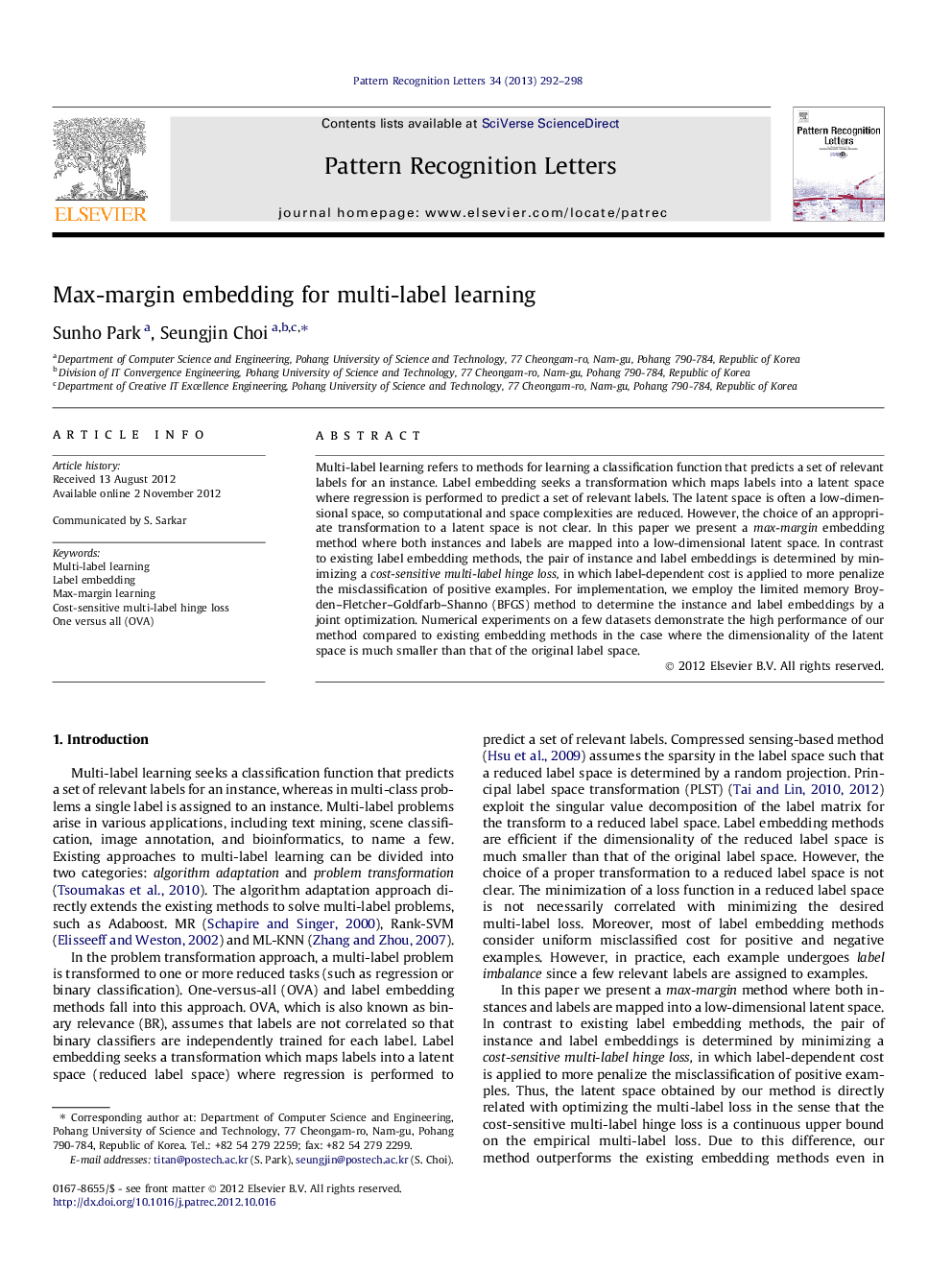
Multi-label learning refers to methods for learning a classification function that predicts a set of relevant labels for an instance. Label embedding seeks a transformation which maps labels into a latent space where regression is performed to predict a set of relevant labels. The latent space is often a low-dimensional space, so computational and space complexities are reduced. However, the choice of an appropriate transformation to a latent space is not clear. In this paper we present a max-margin embedding method where both instances and labels are mapped into a low-dimensional latent space. In contrast to existing label embedding methods, the pair of instance and label embeddings is determined by minimizing a cost-sensitive multi-label hinge loss, in which label-dependent cost is applied to more penalize the misclassification of positive examples. For implementation, we employ the limited memory Broyden–Fletcher–Goldfarb–Shanno (BFGS) method to determine the instance and label embeddings by a joint optimization. Numerical experiments on a few datasets demonstrate the high performance of our method compared to existing embedding methods in the case where the dimensionality of the latent space is much smaller than that of the original label space.
► Label embedding for multi-label problems finds a reduced latent space of labels.
► The latent space was not necessarily correlated with minimizing a multi-label loss.
► We give a max-margin embedding method that minimizes a cost-sensitive multi-label hinge loss.
► Our method is superior to the case of the lower dimensional latent space.
► Our method is effective when positive examples are much less than negative examples.
Journal: Pattern Recognition Letters - Volume 34, Issue 3, 1 February 2013, Pages 292–298