کد مقاله | کد نشریه | سال انتشار | مقاله انگلیسی | نسخه تمام متن |
---|---|---|---|---|
535792 | 870379 | 2012 | 11 صفحه PDF | دانلود رایگان |
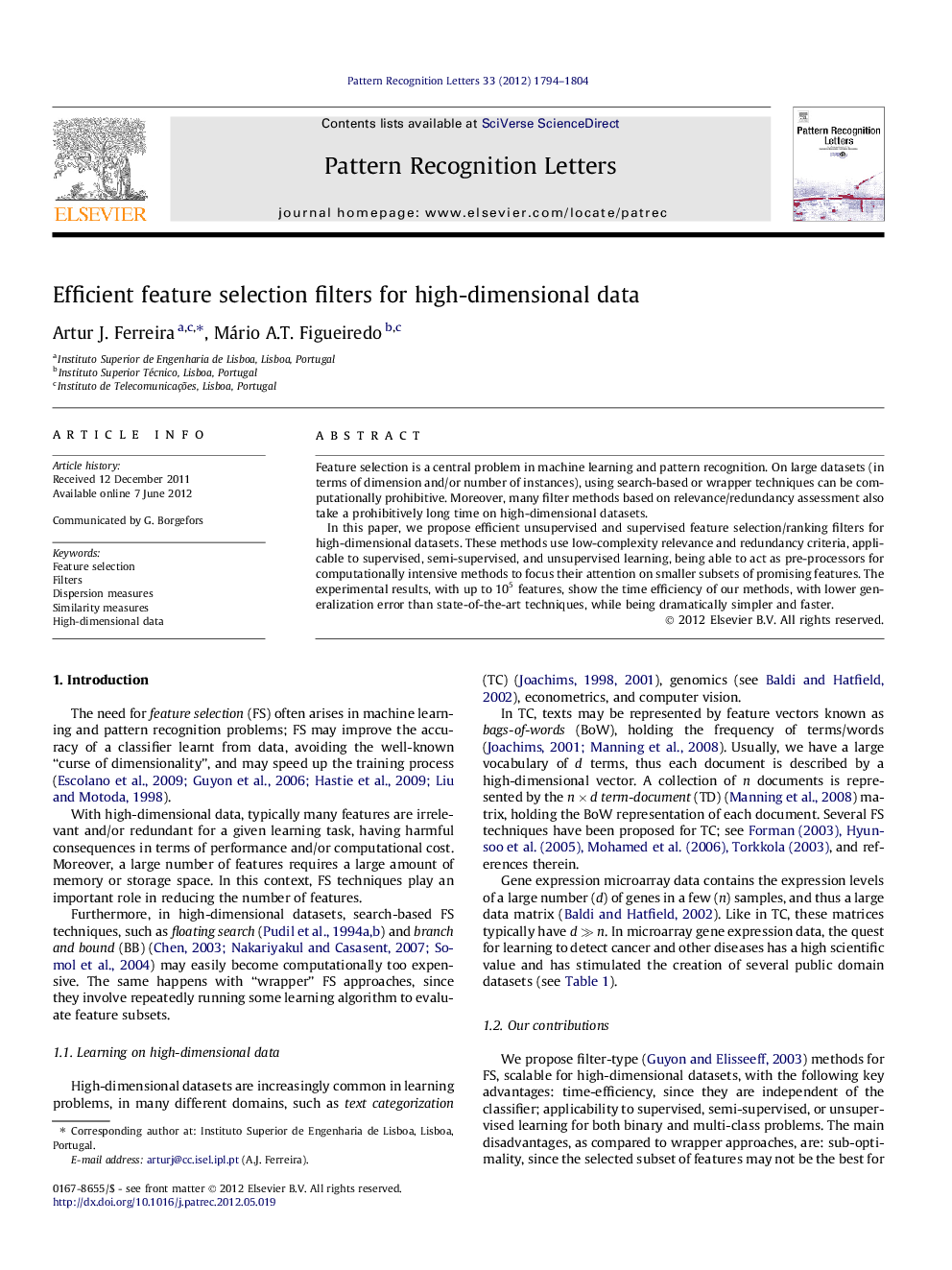
Feature selection is a central problem in machine learning and pattern recognition. On large datasets (in terms of dimension and/or number of instances), using search-based or wrapper techniques can be computationally prohibitive. Moreover, many filter methods based on relevance/redundancy assessment also take a prohibitively long time on high-dimensional datasets.In this paper, we propose efficient unsupervised and supervised feature selection/ranking filters for high-dimensional datasets. These methods use low-complexity relevance and redundancy criteria, applicable to supervised, semi-supervised, and unsupervised learning, being able to act as pre-processors for computationally intensive methods to focus their attention on smaller subsets of promising features. The experimental results, with up to 105105 features, show the time efficiency of our methods, with lower generalization error than state-of-the-art techniques, while being dramatically simpler and faster.
► Two new log-linear time filter unsupervised feature selection methods.
► Relevance computed by dispersion; redundancy computed efficiently.
► Suited for binary and multi-class high-dimensional datasets.
► Very fast FS results on standard benchmark datasets.
Journal: Pattern Recognition Letters - Volume 33, Issue 13, 1 October 2012, Pages 1794–1804