کد مقاله | کد نشریه | سال انتشار | مقاله انگلیسی | نسخه تمام متن |
---|---|---|---|---|
535929 | 870412 | 2011 | 8 صفحه PDF | دانلود رایگان |
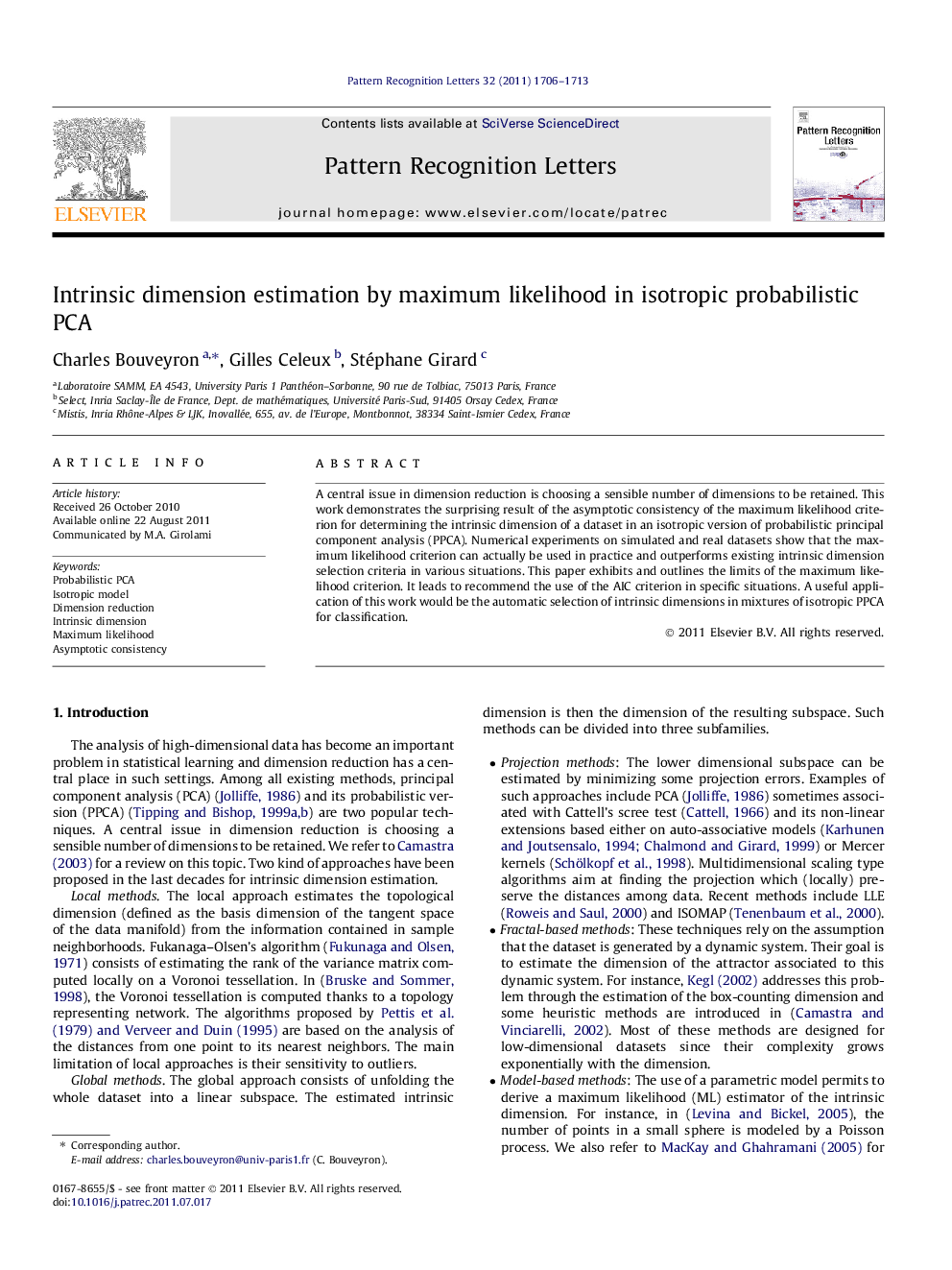
A central issue in dimension reduction is choosing a sensible number of dimensions to be retained. This work demonstrates the surprising result of the asymptotic consistency of the maximum likelihood criterion for determining the intrinsic dimension of a dataset in an isotropic version of probabilistic principal component analysis (PPCA). Numerical experiments on simulated and real datasets show that the maximum likelihood criterion can actually be used in practice and outperforms existing intrinsic dimension selection criteria in various situations. This paper exhibits and outlines the limits of the maximum likelihood criterion. It leads to recommend the use of the AIC criterion in specific situations. A useful application of this work would be the automatic selection of intrinsic dimensions in mixtures of isotropic PPCA for classification.
► This work considers an isotropic version of the PPCA model.
► The consistency of the ML criterion for intrinsic dimension estimation is proved.
► Numerical experiments show that the ML criterion can actually be used in practice.
► This paper also exhibits the limits of the ML criterion in practice.
► It leads to recommend the use of the AIC criterion in specific situations.
Journal: Pattern Recognition Letters - Volume 32, Issue 14, 15 October 2011, Pages 1706–1713