کد مقاله | کد نشریه | سال انتشار | مقاله انگلیسی | نسخه تمام متن |
---|---|---|---|---|
536017 | 870429 | 2011 | 13 صفحه PDF | دانلود رایگان |
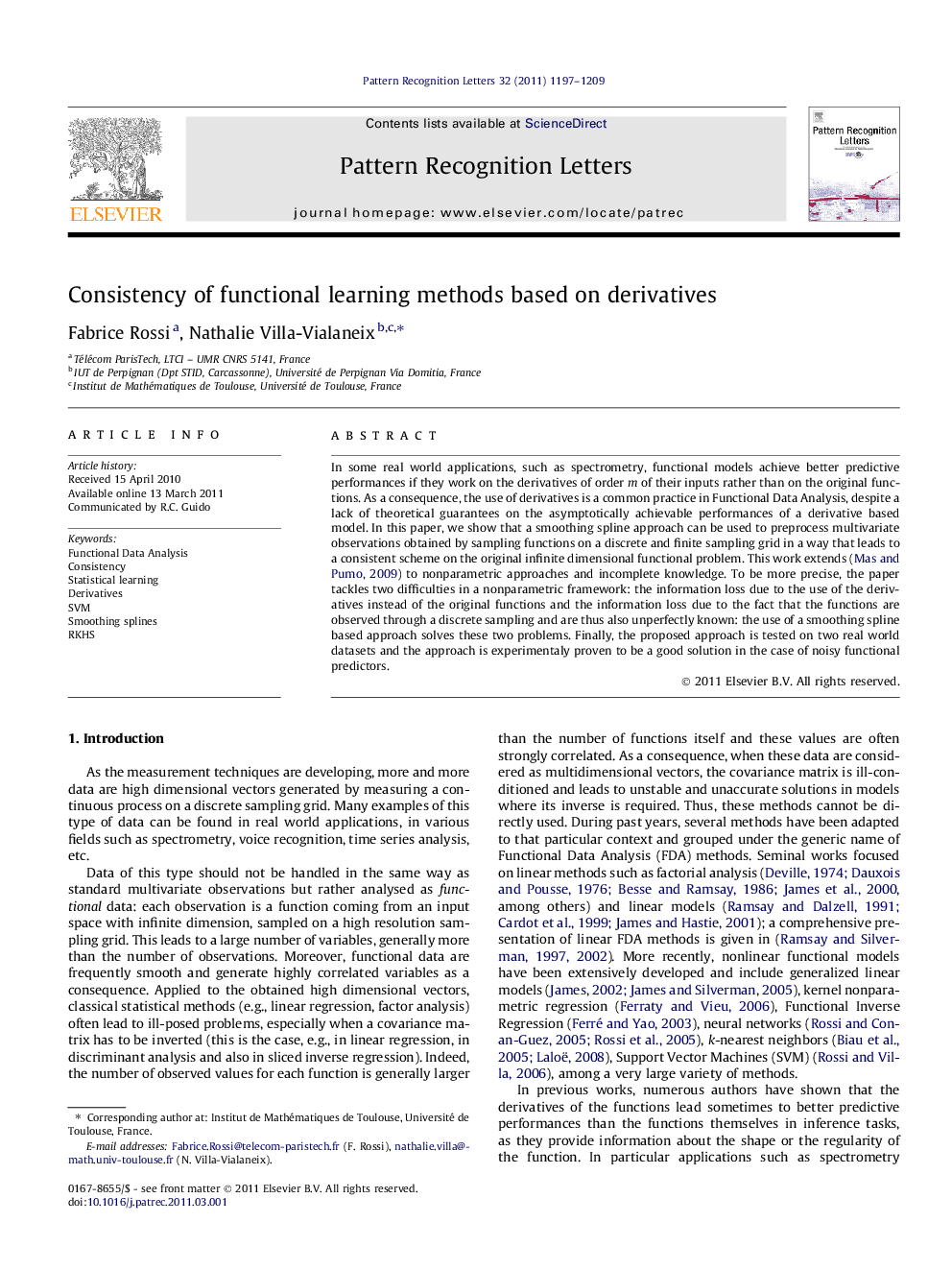
In some real world applications, such as spectrometry, functional models achieve better predictive performances if they work on the derivatives of order m of their inputs rather than on the original functions. As a consequence, the use of derivatives is a common practice in Functional Data Analysis, despite a lack of theoretical guarantees on the asymptotically achievable performances of a derivative based model. In this paper, we show that a smoothing spline approach can be used to preprocess multivariate observations obtained by sampling functions on a discrete and finite sampling grid in a way that leads to a consistent scheme on the original infinite dimensional functional problem. This work extends ( Mas and Pumo, 2009) to nonparametric approaches and incomplete knowledge. To be more precise, the paper tackles two difficulties in a nonparametric framework: the information loss due to the use of the derivatives instead of the original functions and the information loss due to the fact that the functions are observed through a discrete sampling and are thus also unperfectly known: the use of a smoothing spline based approach solves these two problems. Finally, the proposed approach is tested on two real world datasets and the approach is experimentaly proven to be a good solution in the case of noisy functional predictors.
► The use of derivatives in learning problems where the predictors are curves is dealt with.
► A spline based pre-processing equivalent to derivation on sampled functions is used.
► The consistency of the method is proved.
► The method is illustrated on spectrometric datasets.
► The simulations also include a study of the impact of noisy predictors.
Journal: Pattern Recognition Letters - Volume 32, Issue 8, 1 June 2011, Pages 1197–1209