کد مقاله | کد نشریه | سال انتشار | مقاله انگلیسی | نسخه تمام متن |
---|---|---|---|---|
536405 | 870510 | 2013 | 10 صفحه PDF | دانلود رایگان |
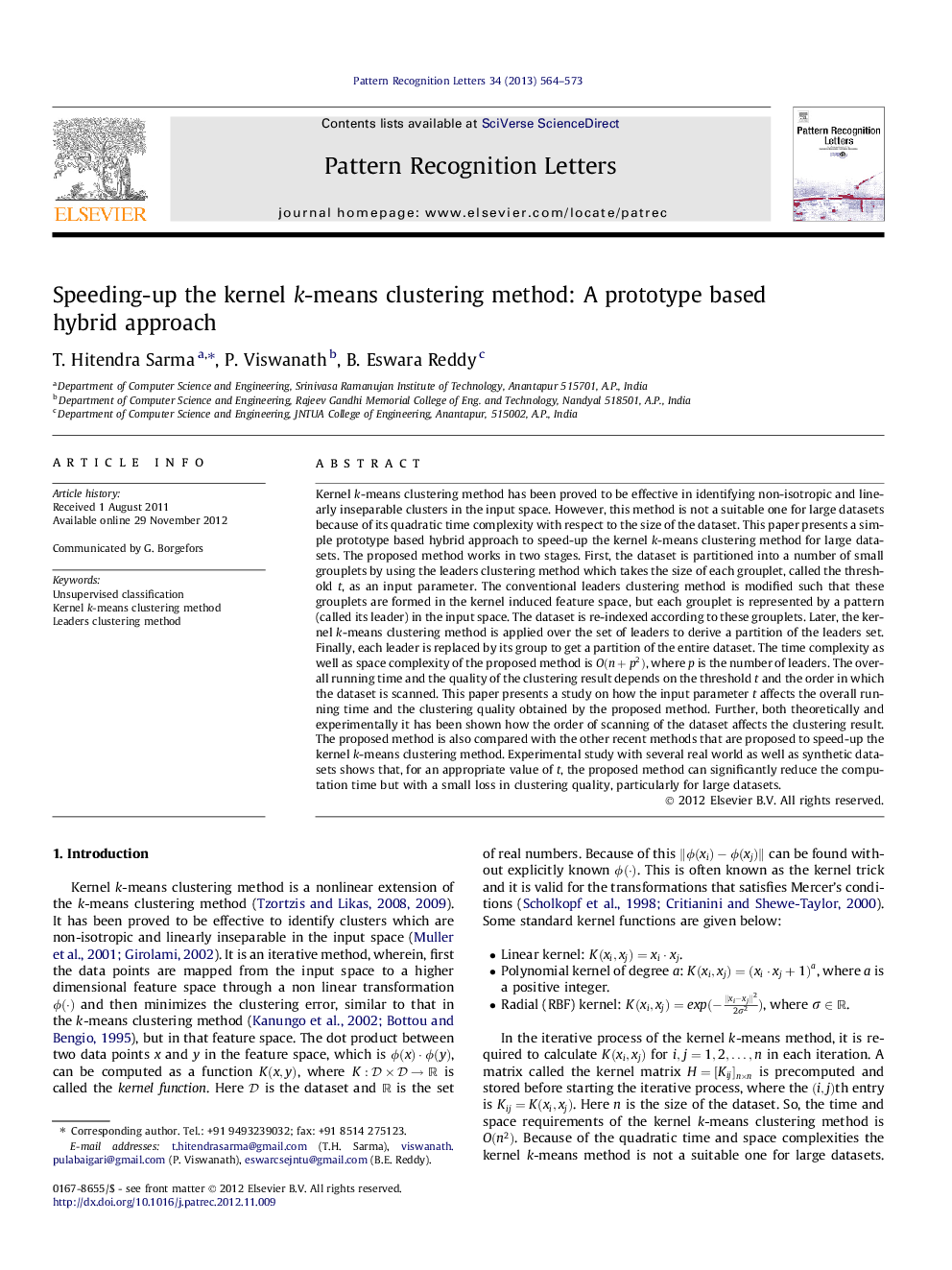
Kernel k-means clustering method has been proved to be effective in identifying non-isotropic and linearly inseparable clusters in the input space. However, this method is not a suitable one for large datasets because of its quadratic time complexity with respect to the size of the dataset. This paper presents a simple prototype based hybrid approach to speed-up the kernel k-means clustering method for large datasets. The proposed method works in two stages. First, the dataset is partitioned into a number of small grouplets by using the leaders clustering method which takes the size of each grouplet, called the threshold t, as an input parameter. The conventional leaders clustering method is modified such that these grouplets are formed in the kernel induced feature space, but each grouplet is represented by a pattern (called its leader) in the input space. The dataset is re-indexed according to these grouplets. Later, the kernel k -means clustering method is applied over the set of leaders to derive a partition of the leaders set. Finally, each leader is replaced by its group to get a partition of the entire dataset. The time complexity as well as space complexity of the proposed method is O(n+p2)O(n+p2), where p is the number of leaders. The overall running time and the quality of the clustering result depends on the threshold t and the order in which the dataset is scanned. This paper presents a study on how the input parameter t affects the overall running time and the clustering quality obtained by the proposed method. Further, both theoretically and experimentally it has been shown how the order of scanning of the dataset affects the clustering result. The proposed method is also compared with the other recent methods that are proposed to speed-up the kernel k-means clustering method. Experimental study with several real world as well as synthetic datasets shows that, for an appropriate value of t, the proposed method can significantly reduce the computation time but with a small loss in clustering quality, particularly for large datasets.
► Kernel k means is a good clustering method in finding non-isotropic clusters.
► Its time requirement is quadratic with respect to the number of patterns.
► The paper proposes a hybrid clustering approach.
► First group-lets are found, later clustering is done using these group-lets.
► Proposed method is a fast and scalable one to work with large data-sets.
Journal: Pattern Recognition Letters - Volume 34, Issue 5, 1 April 2013, Pages 564–573