کد مقاله | کد نشریه | سال انتشار | مقاله انگلیسی | نسخه تمام متن |
---|---|---|---|---|
536408 | 870510 | 2013 | 8 صفحه PDF | دانلود رایگان |
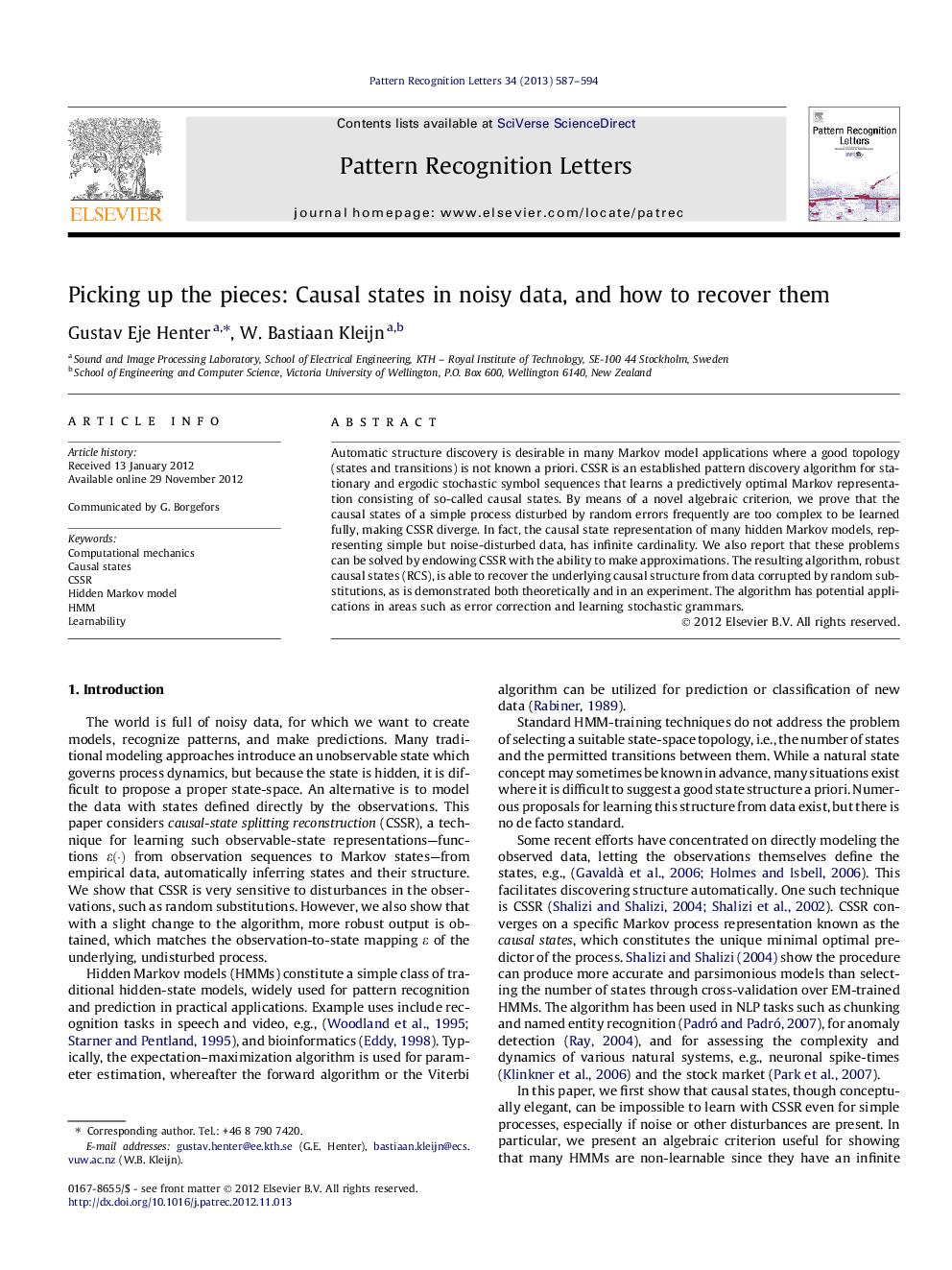
Automatic structure discovery is desirable in many Markov model applications where a good topology (states and transitions) is not known a priori. CSSR is an established pattern discovery algorithm for stationary and ergodic stochastic symbol sequences that learns a predictively optimal Markov representation consisting of so-called causal states. By means of a novel algebraic criterion, we prove that the causal states of a simple process disturbed by random errors frequently are too complex to be learned fully, making CSSR diverge. In fact, the causal state representation of many hidden Markov models, representing simple but noise-disturbed data, has infinite cardinality. We also report that these problems can be solved by endowing CSSR with the ability to make approximations. The resulting algorithm, robust causal states (RCS), is able to recover the underlying causal structure from data corrupted by random substitutions, as is demonstrated both theoretically and in an experiment. The algorithm has potential applications in areas such as error correction and learning stochastic grammars.
► CSSR is an unsupervised algorithm for building Markov models of data.
► We show CSSR cannot learn many processes that have been disturbed by noise.
► This is based on showing that certain HMMs are not CSSR-learnable.
► Our robust algorithm is able to recover underlying structure also from disturbed data.
Journal: Pattern Recognition Letters - Volume 34, Issue 5, 1 April 2013, Pages 587–594