کد مقاله | کد نشریه | سال انتشار | مقاله انگلیسی | نسخه تمام متن |
---|---|---|---|---|
536433 | 870523 | 2013 | 8 صفحه PDF | دانلود رایگان |
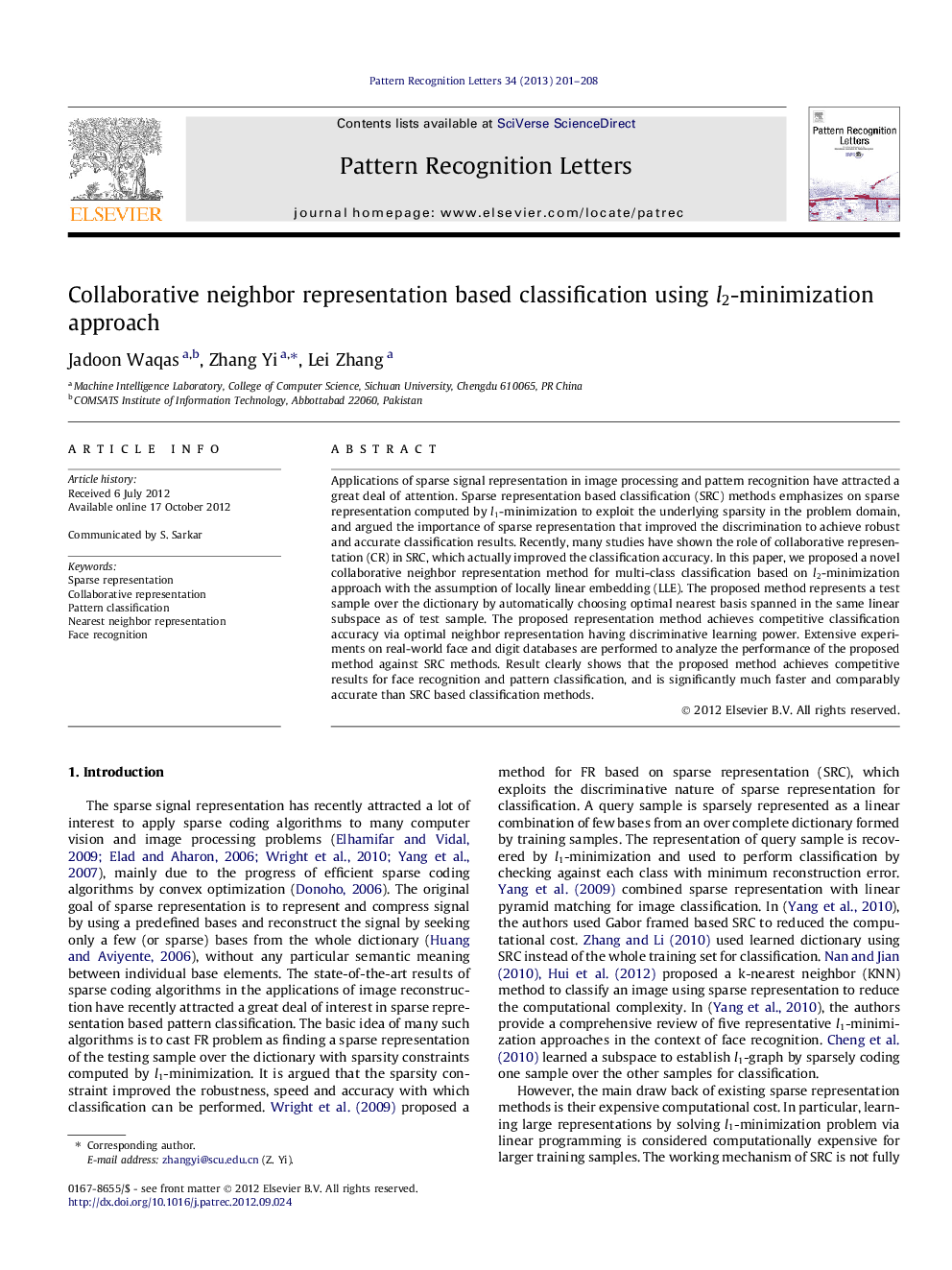
Applications of sparse signal representation in image processing and pattern recognition have attracted a great deal of attention. Sparse representation based classification (SRC) methods emphasizes on sparse representation computed by l1l1-minimization to exploit the underlying sparsity in the problem domain, and argued the importance of sparse representation that improved the discrimination to achieve robust and accurate classification results. Recently, many studies have shown the role of collaborative representation (CR) in SRC, which actually improved the classification accuracy. In this paper, we proposed a novel collaborative neighbor representation method for multi-class classification based on l2l2-minimization approach with the assumption of locally linear embedding (LLE). The proposed method represents a test sample over the dictionary by automatically choosing optimal nearest basis spanned in the same linear subspace as of test sample. The proposed representation method achieves competitive classification accuracy via optimal neighbor representation having discriminative learning power. Extensive experiments on real-world face and digit databases are performed to analyze the performance of the proposed method against SRC methods. Result clearly shows that the proposed method achieves competitive results for face recognition and pattern classification, and is significantly much faster and comparably accurate than SRC based classification methods.
► We proposed a novel classification method based on l2-minimization approach.
► Our method automatically selects optimal nearest bases from training dictionary to code a test sample.
► Our method improves the discrimination necessary to classify a test sample correctly.
► Experiments on real world face and digit datsets shows our method outperform SRC.
► Our method achieves acceptable classification accuracy for nonlinear datasets.
Journal: Pattern Recognition Letters - Volume 34, Issue 2, 15 January 2013, Pages 201–208