کد مقاله | کد نشریه | سال انتشار | مقاله انگلیسی | نسخه تمام متن |
---|---|---|---|---|
536493 | 870544 | 2011 | 5 صفحه PDF | دانلود رایگان |
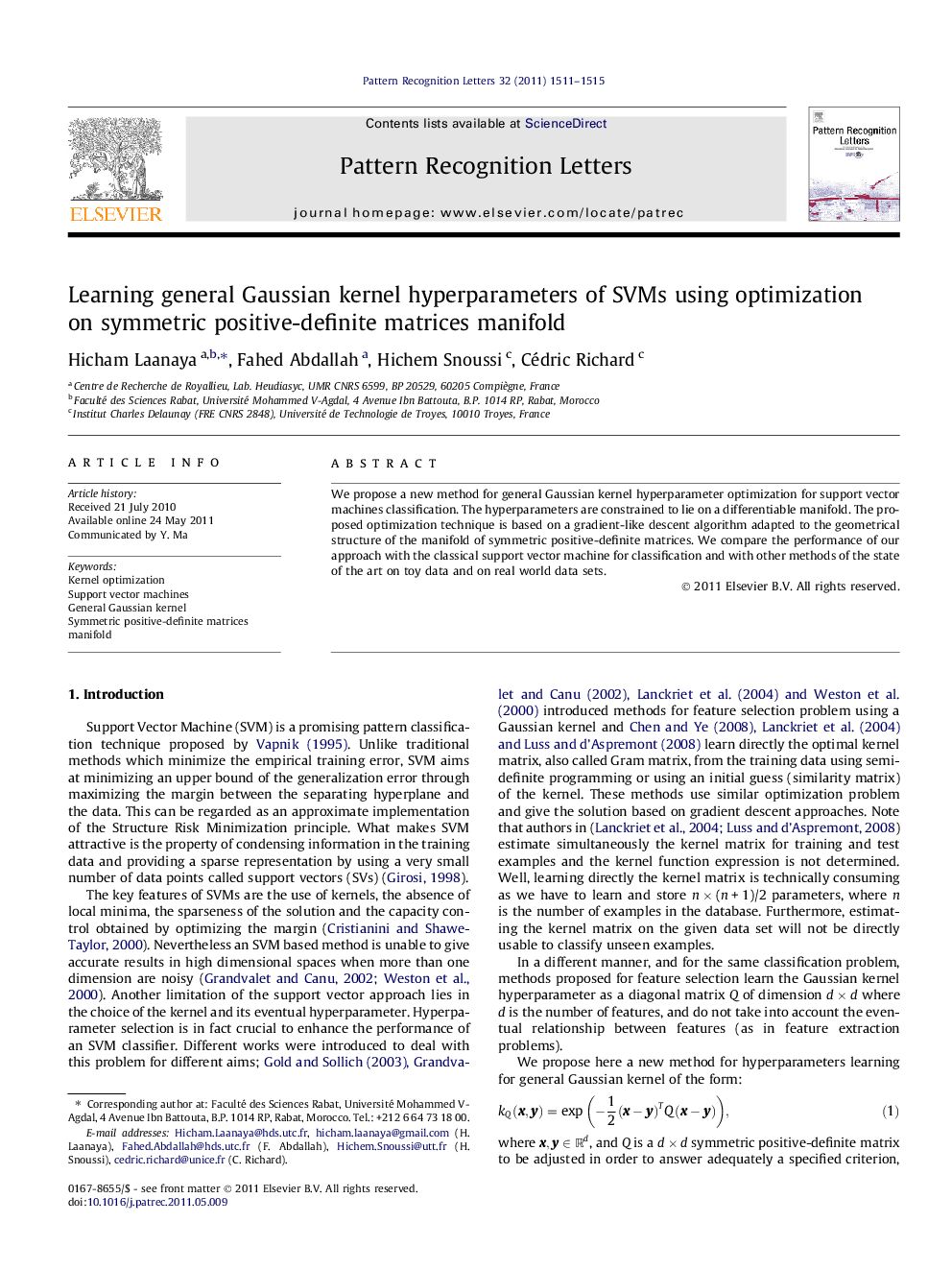
We propose a new method for general Gaussian kernel hyperparameter optimization for support vector machines classification. The hyperparameters are constrained to lie on a differentiable manifold. The proposed optimization technique is based on a gradient-like descent algorithm adapted to the geometrical structure of the manifold of symmetric positive-definite matrices. We compare the performance of our approach with the classical support vector machine for classification and with other methods of the state of the art on toy data and on real world data sets.
► New method for general Gaussian kernel hyperparameter optimization for SVM.
► Optimization technique is based on a gradient-like descent algorithm.
► The optimization is adapted to the manifold of symmetric positive-definite matrices.
► This new method adapts the orientation detect correlations in the input data.
Journal: Pattern Recognition Letters - Volume 32, Issue 13, 1 October 2011, Pages 1511–1515