کد مقاله | کد نشریه | سال انتشار | مقاله انگلیسی | نسخه تمام متن |
---|---|---|---|---|
536530 | 870551 | 2011 | 7 صفحه PDF | دانلود رایگان |
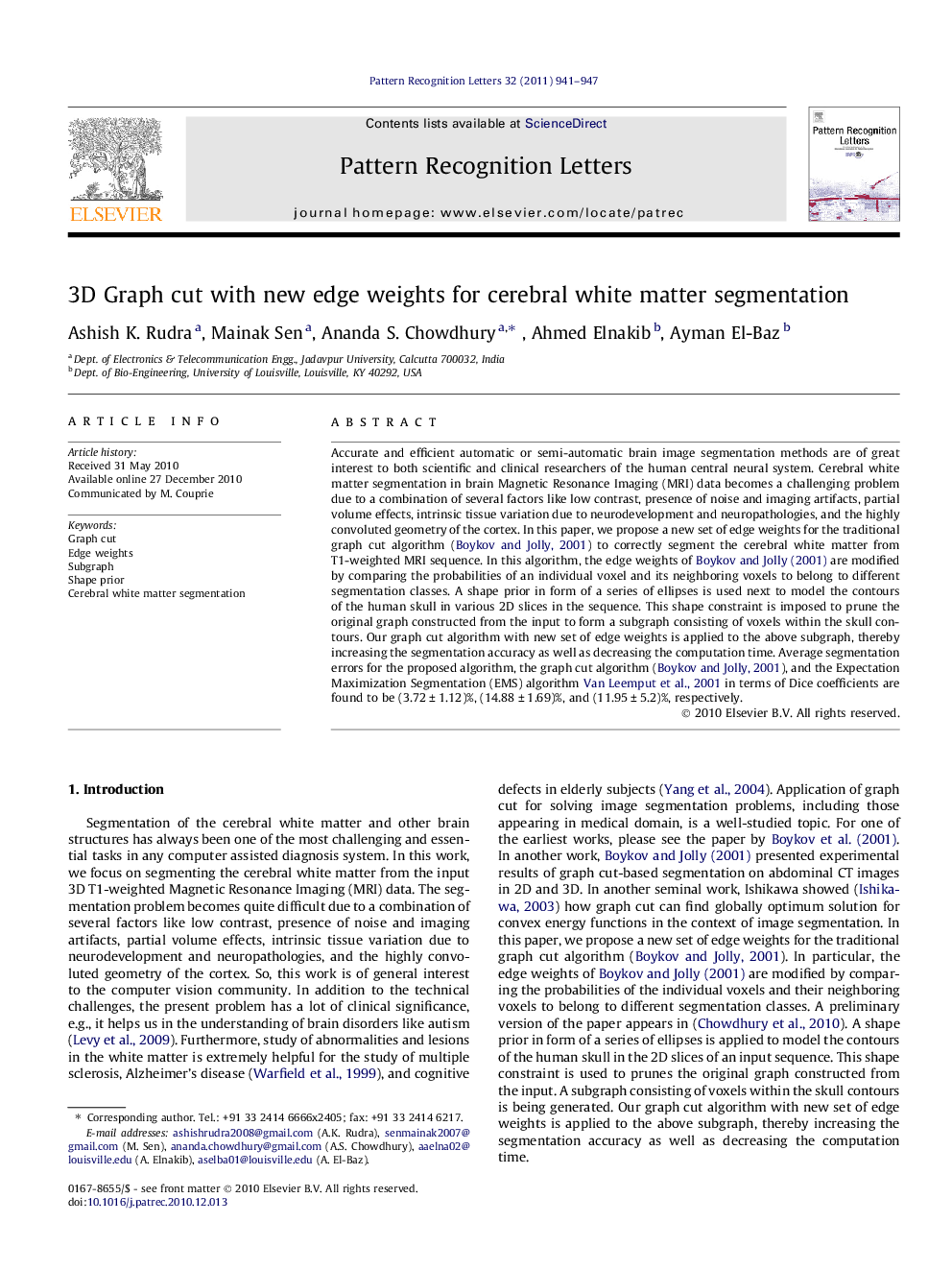
Accurate and efficient automatic or semi-automatic brain image segmentation methods are of great interest to both scientific and clinical researchers of the human central neural system. Cerebral white matter segmentation in brain Magnetic Resonance Imaging (MRI) data becomes a challenging problem due to a combination of several factors like low contrast, presence of noise and imaging artifacts, partial volume effects, intrinsic tissue variation due to neurodevelopment and neuropathologies, and the highly convoluted geometry of the cortex. In this paper, we propose a new set of edge weights for the traditional graph cut algorithm (Boykov and Jolly, 2001) to correctly segment the cerebral white matter from T1-weighted MRI sequence. In this algorithm, the edge weights of Boykov and Jolly (2001) are modified by comparing the probabilities of an individual voxel and its neighboring voxels to belong to different segmentation classes. A shape prior in form of a series of ellipses is used next to model the contours of the human skull in various 2D slices in the sequence. This shape constraint is imposed to prune the original graph constructed from the input to form a subgraph consisting of voxels within the skull contours. Our graph cut algorithm with new set of edge weights is applied to the above subgraph, thereby increasing the segmentation accuracy as well as decreasing the computation time. Average segmentation errors for the proposed algorithm, the graph cut algorithm (Boykov and Jolly, 2001), and the Expectation Maximization Segmentation (EMS) algorithm Van Leemput et al., 2001 in terms of Dice coefficients are found to be (3.72 ± 1.12)%, (14.88 ± 1.69)%, and (11.95 ± 5.2)%, respectively.
Research highlights
► Design of a probabilistic capacity-scaled graph cut algorithm. The probabilistic capacity scaling effectively handles low contrast between the cerebral white matter and the surrounding anatomical structures in the MRI data.
► Introduction of a voxel-wise parameter estimation strategy for graph cut based on the probabilities of its surrounding voxels to belong to the object class and the background class. This step assigns suitable weights to the smoothness and the data terms of each individual voxel based on its location.
► Use of a simple yet effective geometric shape prior. The shape constraint generates a subgraph by pruning a significant part of the 3D graph (constructed from the input). The proposed algorithm is applied on the newly formed subgraph which results in a significant improvement of execution time of the algorithm as well as the accuracy of segmentation.
Journal: Pattern Recognition Letters - Volume 32, Issue 7, 1 May 2011, Pages 941–947