کد مقاله | کد نشریه | سال انتشار | مقاله انگلیسی | نسخه تمام متن |
---|---|---|---|---|
555161 | 1451305 | 2012 | 9 صفحه PDF | دانلود رایگان |
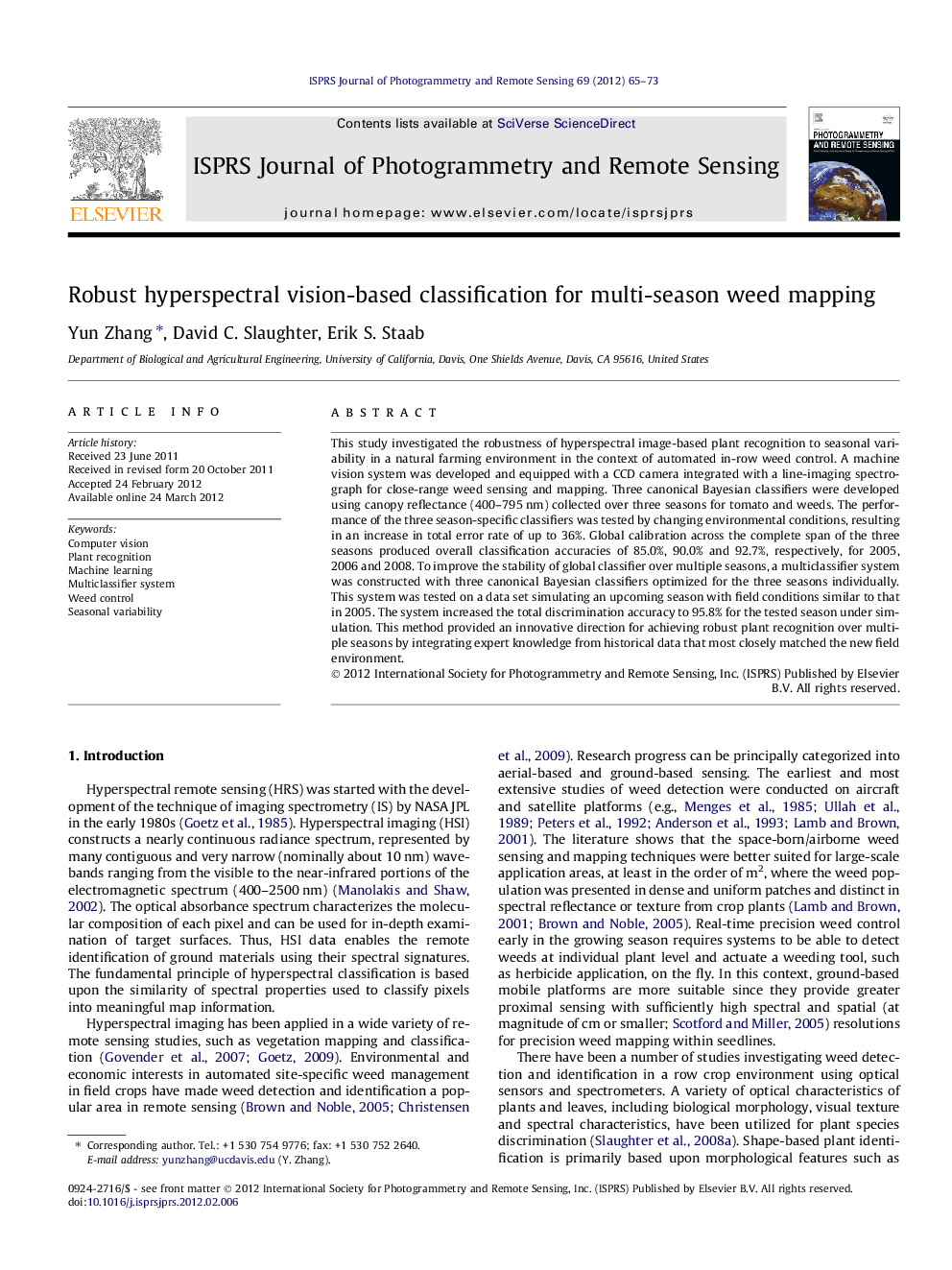
This study investigated the robustness of hyperspectral image-based plant recognition to seasonal variability in a natural farming environment in the context of automated in-row weed control. A machine vision system was developed and equipped with a CCD camera integrated with a line-imaging spectrograph for close-range weed sensing and mapping. Three canonical Bayesian classifiers were developed using canopy reflectance (400–795 nm) collected over three seasons for tomato and weeds. The performance of the three season-specific classifiers was tested by changing environmental conditions, resulting in an increase in total error rate of up to 36%. Global calibration across the complete span of the three seasons produced overall classification accuracies of 85.0%, 90.0% and 92.7%, respectively, for 2005, 2006 and 2008. To improve the stability of global classifier over multiple seasons, a multiclassifier system was constructed with three canonical Bayesian classifiers optimized for the three seasons individually. This system was tested on a data set simulating an upcoming season with field conditions similar to that in 2005. The system increased the total discrimination accuracy to 95.8% for the tested season under simulation. This method provided an innovative direction for achieving robust plant recognition over multiple seasons by integrating expert knowledge from historical data that most closely matched the new field environment.
Journal: ISPRS Journal of Photogrammetry and Remote Sensing - Volume 69, April 2012, Pages 65–73