کد مقاله | کد نشریه | سال انتشار | مقاله انگلیسی | نسخه تمام متن |
---|---|---|---|---|
562235 | 1451943 | 2016 | 12 صفحه PDF | دانلود رایگان |
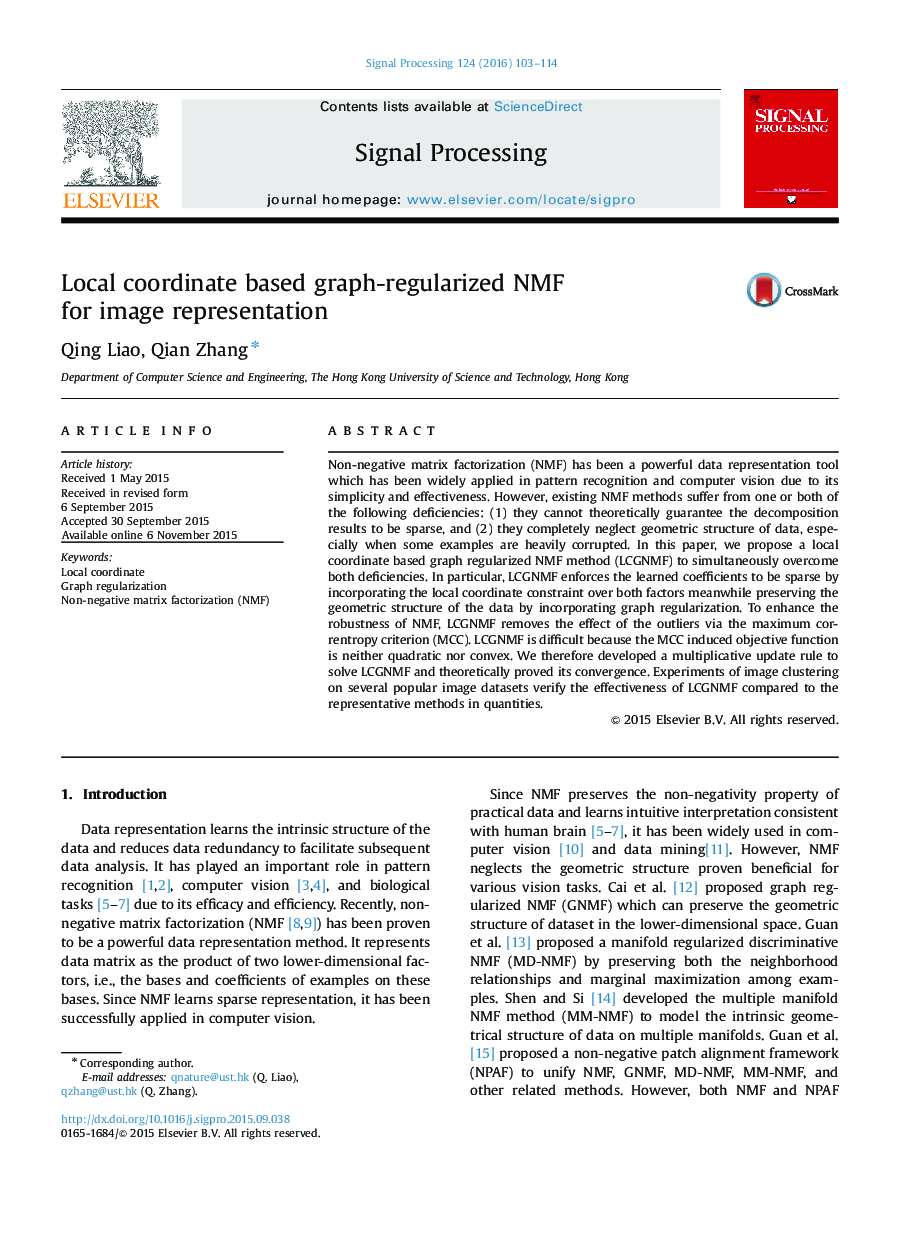
Non-negative matrix factorization (NMF) has been a powerful data representation tool which has been widely applied in pattern recognition and computer vision due to its simplicity and effectiveness. However, existing NMF methods suffer from one or both of the following deficiencies: (1) they cannot theoretically guarantee the decomposition results to be sparse, and (2) they completely neglect geometric structure of data, especially when some examples are heavily corrupted. In this paper, we propose a local coordinate based graph regularized NMF method (LCGNMF) to simultaneously overcome both deficiencies. In particular, LCGNMF enforces the learned coefficients to be sparse by incorporating the local coordinate constraint over both factors meanwhile preserving the geometric structure of the data by incorporating graph regularization. To enhance the robustness of NMF, LCGNMF removes the effect of the outliers via the maximum correntropy criterion (MCC). LCGNMF is difficult because the MCC induced objective function is neither quadratic nor convex. We therefore developed a multiplicative update rule to solve LCGNMF and theoretically proved its convergence. Experiments of image clustering on several popular image datasets verify the effectiveness of LCGNMF compared to the representative methods in quantities.
Journal: Signal Processing - Volume 124, July 2016, Pages 103–114