کد مقاله | کد نشریه | سال انتشار | مقاله انگلیسی | نسخه تمام متن |
---|---|---|---|---|
562587 | 1451667 | 2014 | 9 صفحه PDF | دانلود رایگان |
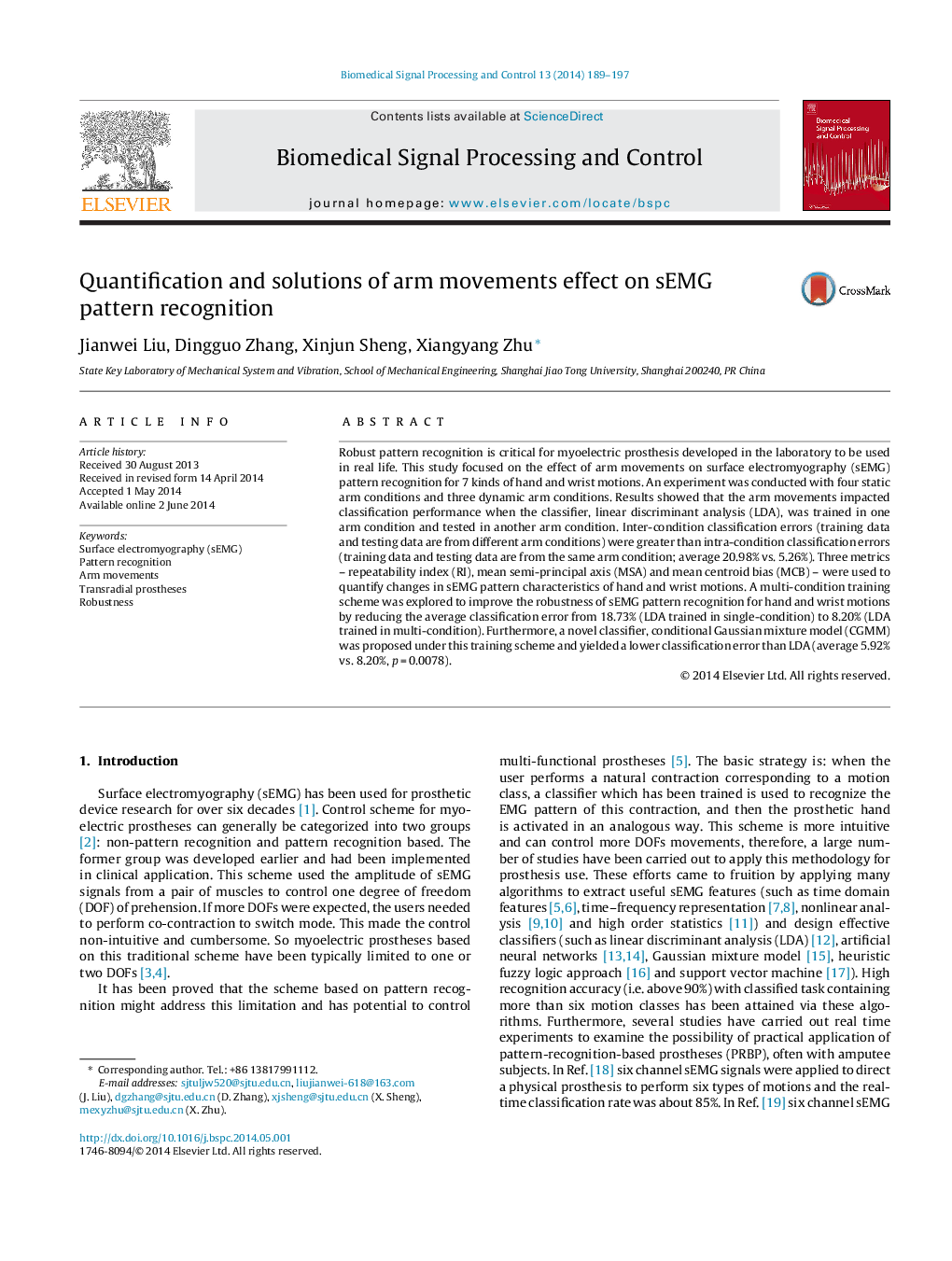
• Arm movements, especially the dynamic change of arm position, have adverse effect on surface electromyography pattern recognition of wrist or hand motions, which results in very high classification errors.
• We adopted three metrics to quantify the changes of characteristics in the feature space due to arm movements.
• Arm movements caused the deviation of class centroid and made the intra-class dispersion greater.
• A robust training scheme was used to eliminate this effect and a novel classifier, conditional Gaussian mixture model, was proposed to improve the classification performance under this training scheme.
Robust pattern recognition is critical for myoelectric prosthesis developed in the laboratory to be used in real life. This study focused on the effect of arm movements on surface electromyography (sEMG) pattern recognition for 7 kinds of hand and wrist motions. An experiment was conducted with four static arm conditions and three dynamic arm conditions. Results showed that the arm movements impacted classification performance when the classifier, linear discriminant analysis (LDA), was trained in one arm condition and tested in another arm condition. Inter-condition classification errors (training data and testing data are from different arm conditions) were greater than intra-condition classification errors (training data and testing data are from the same arm condition; average 20.98% vs. 5.26%). Three metrics – repeatability index (RI), mean semi-principal axis (MSA) and mean centroid bias (MCB) – were used to quantify changes in sEMG pattern characteristics of hand and wrist motions. A multi-condition training scheme was explored to improve the robustness of sEMG pattern recognition for hand and wrist motions by reducing the average classification error from 18.73% (LDA trained in single-condition) to 8.20% (LDA trained in multi-condition). Furthermore, a novel classifier, conditional Gaussian mixture model (CGMM) was proposed under this training scheme and yielded a lower classification error than LDA (average 5.92% vs. 8.20%, p = 0.0078).
Journal: Biomedical Signal Processing and Control - Volume 13, September 2014, Pages 189–197