کد مقاله | کد نشریه | سال انتشار | مقاله انگلیسی | نسخه تمام متن |
---|---|---|---|---|
562599 | 1451667 | 2014 | 10 صفحه PDF | دانلود رایگان |
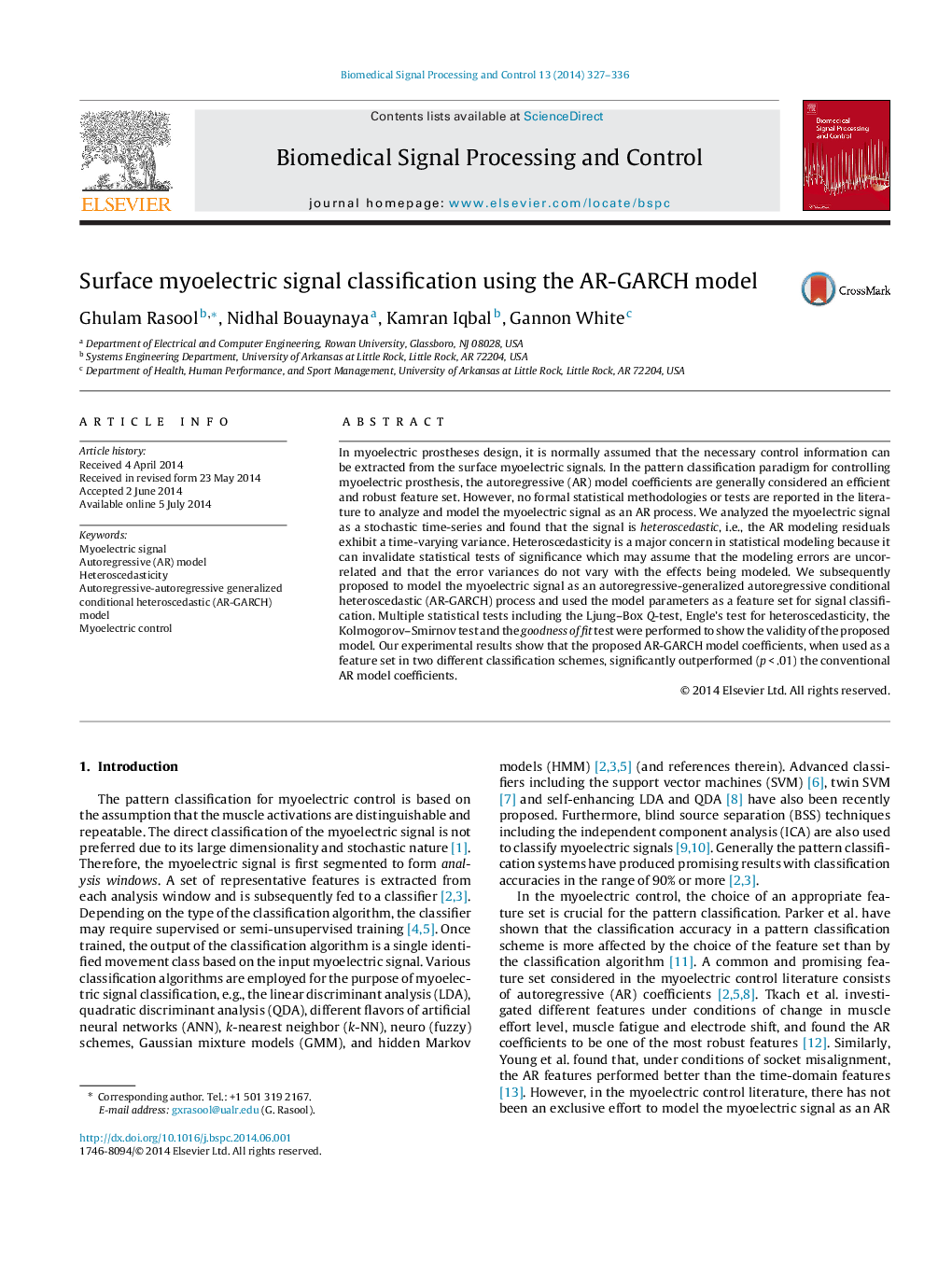
• We analyzed the myoelectric signal as a stochastic time series and found that the signal is heteroscedastic.
• We modeled myoelectric signal as autoregressive-generalized autoregressive conditional heteroscedastic (AR-GARCH) process.
• Estimated parameters of the AR-GARCH model formed the new feature set for the pattern classification.
• Statistical tests including Engle's test, Kolmogorov–Smirnov, goodness of fit and Ljung–Box Q-test were performed.
• Experimental results show that the AR-GARCH model significantly outperforms the conventional strategies.
In myoelectric prostheses design, it is normally assumed that the necessary control information can be extracted from the surface myoelectric signals. In the pattern classification paradigm for controlling myoelectric prosthesis, the autoregressive (AR) model coefficients are generally considered an efficient and robust feature set. However, no formal statistical methodologies or tests are reported in the literature to analyze and model the myoelectric signal as an AR process. We analyzed the myoelectric signal as a stochastic time-series and found that the signal is heteroscedastic, i.e., the AR modeling residuals exhibit a time-varying variance. Heteroscedasticity is a major concern in statistical modeling because it can invalidate statistical tests of significance which may assume that the modeling errors are uncorrelated and that the error variances do not vary with the effects being modeled. We subsequently proposed to model the myoelectric signal as an autoregressive-generalized autoregressive conditional heteroscedastic (AR-GARCH) process and used the model parameters as a feature set for signal classification. Multiple statistical tests including the Ljung–Box Q-test, Engle's test for heteroscedasticity, the Kolmogorov–Smirnov test and the goodness of fit test were performed to show the validity of the proposed model. Our experimental results show that the proposed AR-GARCH model coefficients, when used as a feature set in two different classification schemes, significantly outperformed (p < .01) the conventional AR model coefficients.
Journal: Biomedical Signal Processing and Control - Volume 13, September 2014, Pages 327–336