کد مقاله | کد نشریه | سال انتشار | مقاله انگلیسی | نسخه تمام متن |
---|---|---|---|---|
563045 | 875467 | 2013 | 12 صفحه PDF | دانلود رایگان |
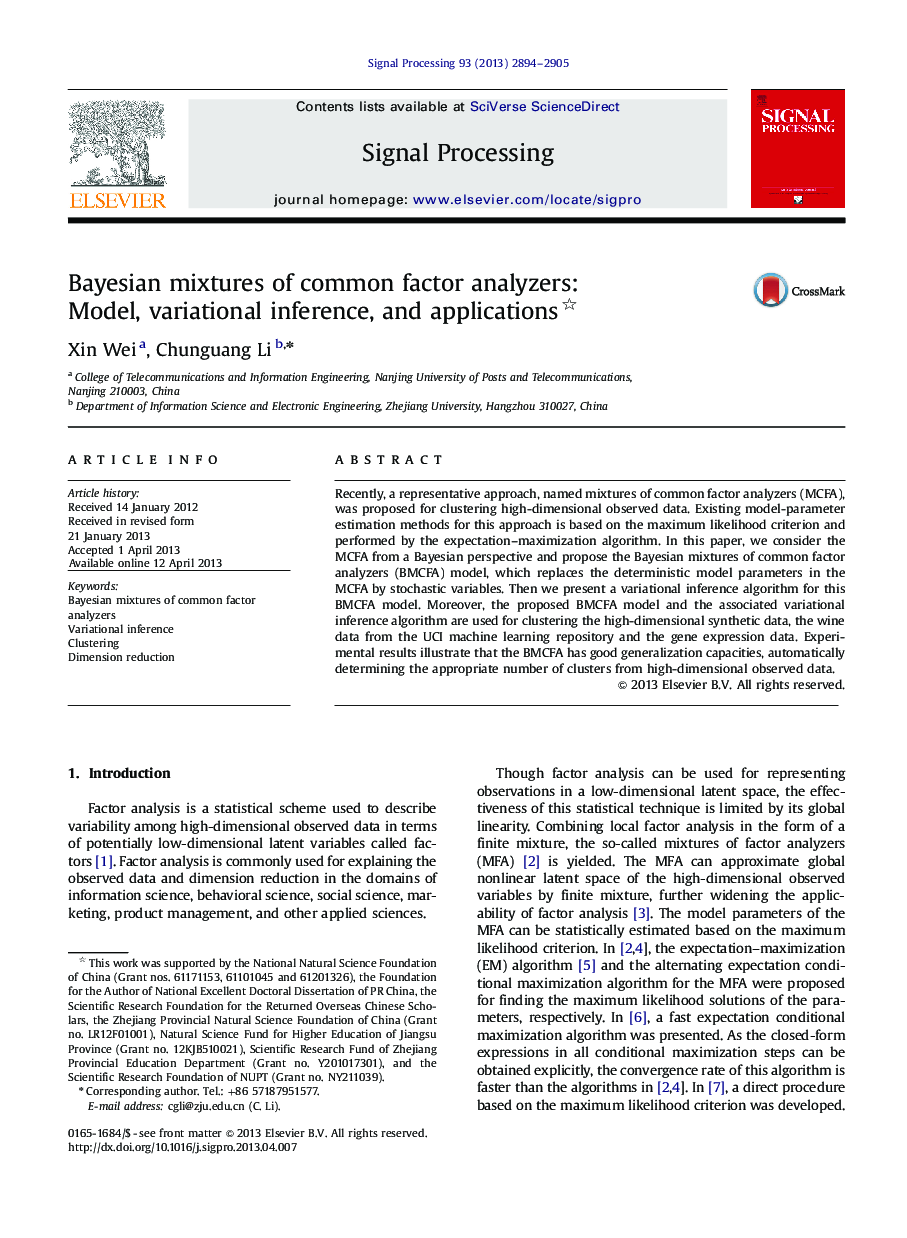
• We propose the Bayesian mixtures of common factor analyzer (BMCFA) model.
• We derive an efficient variational Bayesian inference algorithm for the BMCFA model.
• The BMCFA approach (model+variational inference) is applied to the problems of clustering high-dimensional data sets.
• This approach can automatically determine the appropriate number of clusters.
Recently, a representative approach, named mixtures of common factor analyzers (MCFA), was proposed for clustering high-dimensional observed data. Existing model-parameter estimation methods for this approach is based on the maximum likelihood criterion and performed by the expectation–maximization algorithm. In this paper, we consider the MCFA from a Bayesian perspective and propose the Bayesian mixtures of common factor analyzers (BMCFA) model, which replaces the deterministic model parameters in the MCFA by stochastic variables. Then we present a variational inference algorithm for this BMCFA model. Moreover, the proposed BMCFA model and the associated variational inference algorithm are used for clustering the high-dimensional synthetic data, the wine data from the UCI machine learning repository and the gene expression data. Experimental results illustrate that the BMCFA has good generalization capacities, automatically determining the appropriate number of clusters from high-dimensional observed data.
Journal: Signal Processing - Volume 93, Issue 11, November 2013, Pages 2894–2905