کد مقاله | کد نشریه | سال انتشار | مقاله انگلیسی | نسخه تمام متن |
---|---|---|---|---|
563314 | 875488 | 2008 | 14 صفحه PDF | دانلود رایگان |
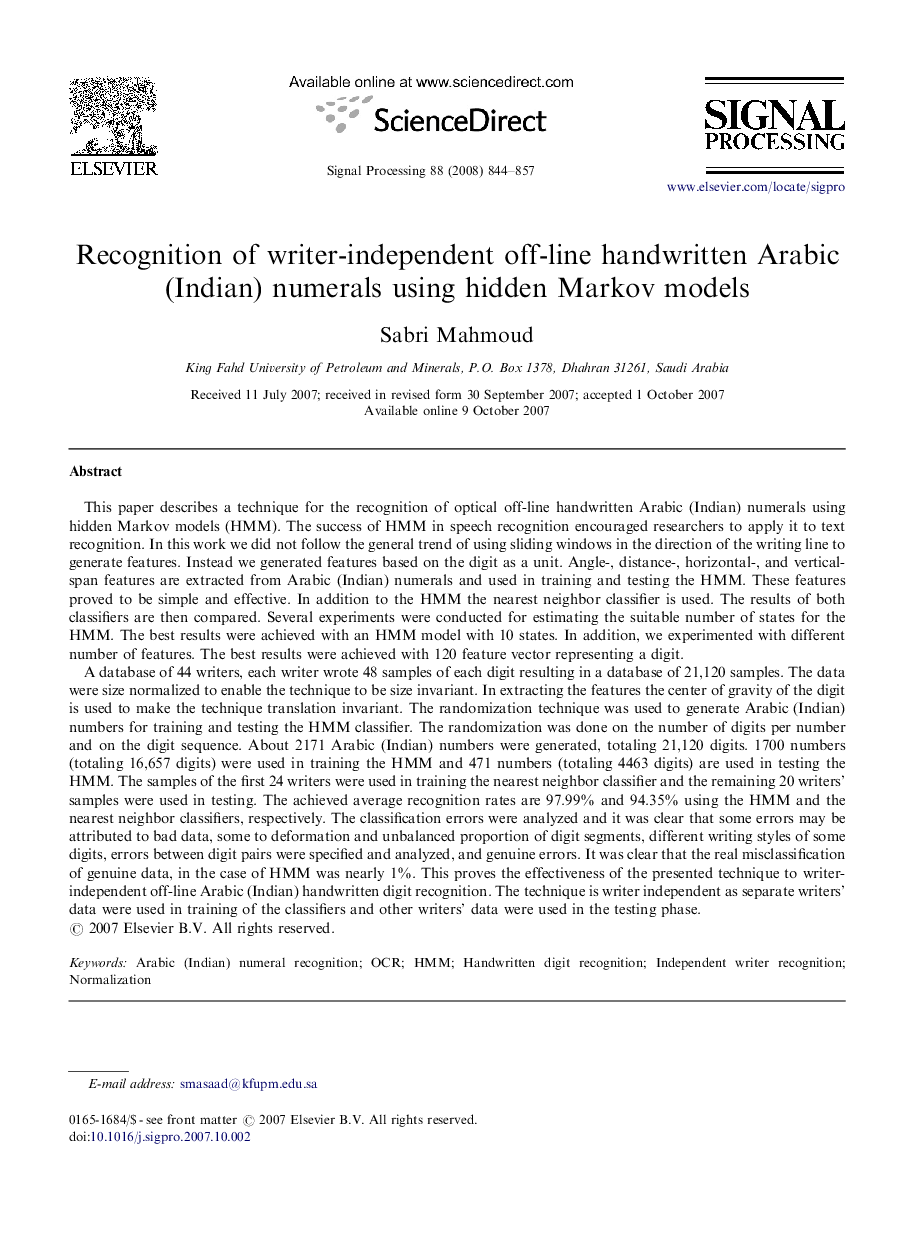
This paper describes a technique for the recognition of optical off-line handwritten Arabic (Indian) numerals using hidden Markov models (HMM). The success of HMM in speech recognition encouraged researchers to apply it to text recognition. In this work we did not follow the general trend of using sliding windows in the direction of the writing line to generate features. Instead we generated features based on the digit as a unit. Angle-, distance-, horizontal-, and vertical-span features are extracted from Arabic (Indian) numerals and used in training and testing the HMM. These features proved to be simple and effective. In addition to the HMM the nearest neighbor classifier is used. The results of both classifiers are then compared. Several experiments were conducted for estimating the suitable number of states for the HMM. The best results were achieved with an HMM model with 10 states. In addition, we experimented with different number of features. The best results were achieved with 120 feature vector representing a digit.A database of 44 writers, each writer wrote 48 samples of each digit resulting in a database of 21,120 samples. The data were size normalized to enable the technique to be size invariant. In extracting the features the center of gravity of the digit is used to make the technique translation invariant. The randomization technique was used to generate Arabic (Indian) numbers for training and testing the HMM classifier. The randomization was done on the number of digits per number and on the digit sequence. About 2171 Arabic (Indian) numbers were generated, totaling 21,120 digits. 1700 numbers (totaling 16,657 digits) were used in training the HMM and 471 numbers (totaling 4463 digits) are used in testing the HMM. The samples of the first 24 writers were used in training the nearest neighbor classifier and the remaining 20 writers’ samples were used in testing. The achieved average recognition rates are 97.99% and 94.35% using the HMM and the nearest neighbor classifiers, respectively. The classification errors were analyzed and it was clear that some errors may be attributed to bad data, some to deformation and unbalanced proportion of digit segments, different writing styles of some digits, errors between digit pairs were specified and analyzed, and genuine errors. It was clear that the real misclassification of genuine data, in the case of HMM was nearly 1%. This proves the effectiveness of the presented technique to writer-independent off-line Arabic (Indian) handwritten digit recognition. The technique is writer independent as separate writers’ data were used in training of the classifiers and other writers’ data were used in the testing phase.
Journal: Signal Processing - Volume 88, Issue 4, April 2008, Pages 844–857