کد مقاله | کد نشریه | سال انتشار | مقاله انگلیسی | نسخه تمام متن |
---|---|---|---|---|
563461 | 875497 | 2007 | 11 صفحه PDF | دانلود رایگان |
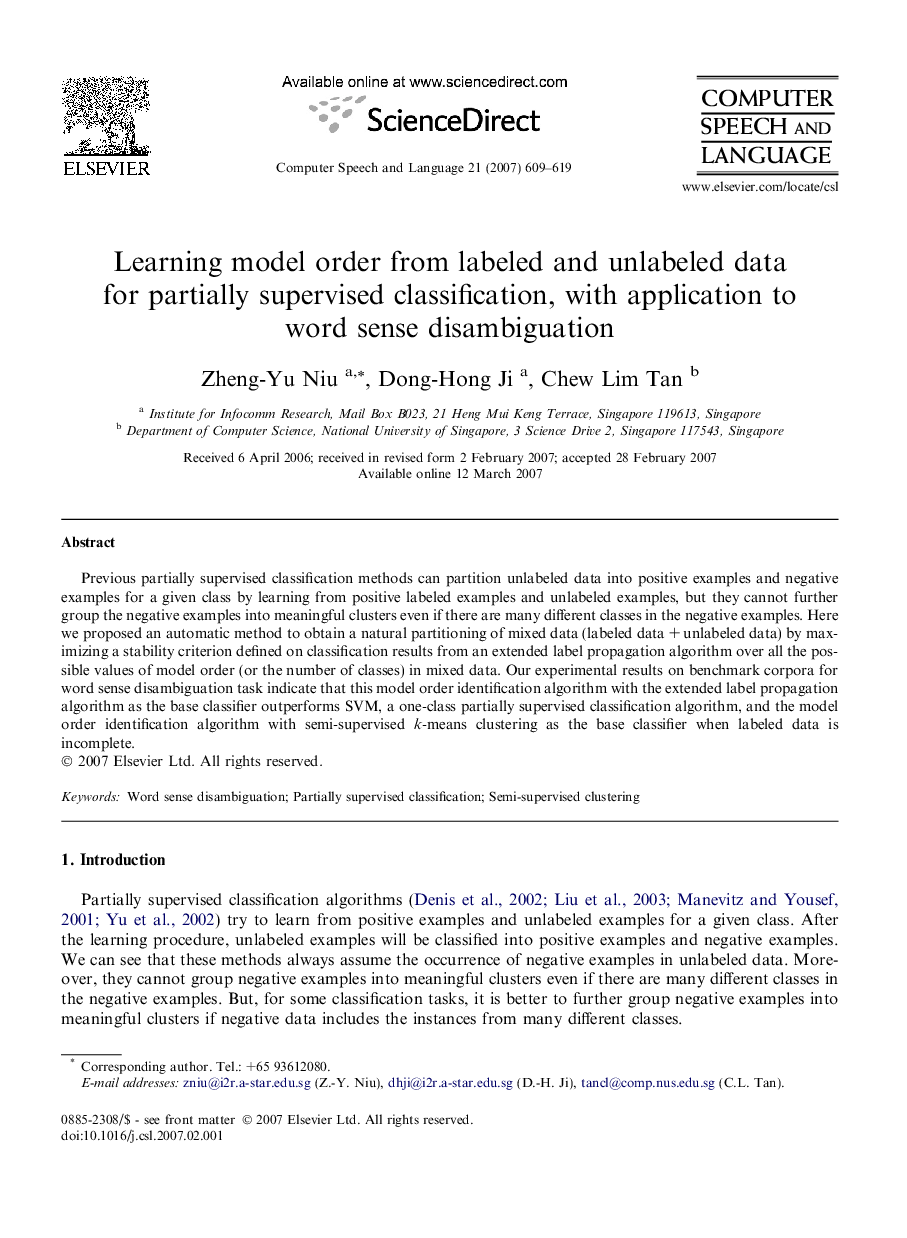
Previous partially supervised classification methods can partition unlabeled data into positive examples and negative examples for a given class by learning from positive labeled examples and unlabeled examples, but they cannot further group the negative examples into meaningful clusters even if there are many different classes in the negative examples. Here we proposed an automatic method to obtain a natural partitioning of mixed data (labeled data + unlabeled data) by maximizing a stability criterion defined on classification results from an extended label propagation algorithm over all the possible values of model order (or the number of classes) in mixed data. Our experimental results on benchmark corpora for word sense disambiguation task indicate that this model order identification algorithm with the extended label propagation algorithm as the base classifier outperforms SVM, a one-class partially supervised classification algorithm, and the model order identification algorithm with semi-supervised k-means clustering as the base classifier when labeled data is incomplete.
Journal: Computer Speech & Language - Volume 21, Issue 4, October 2007, Pages 609–619