کد مقاله | کد نشریه | سال انتشار | مقاله انگلیسی | نسخه تمام متن |
---|---|---|---|---|
564178 | 875575 | 2012 | 12 صفحه PDF | دانلود رایگان |
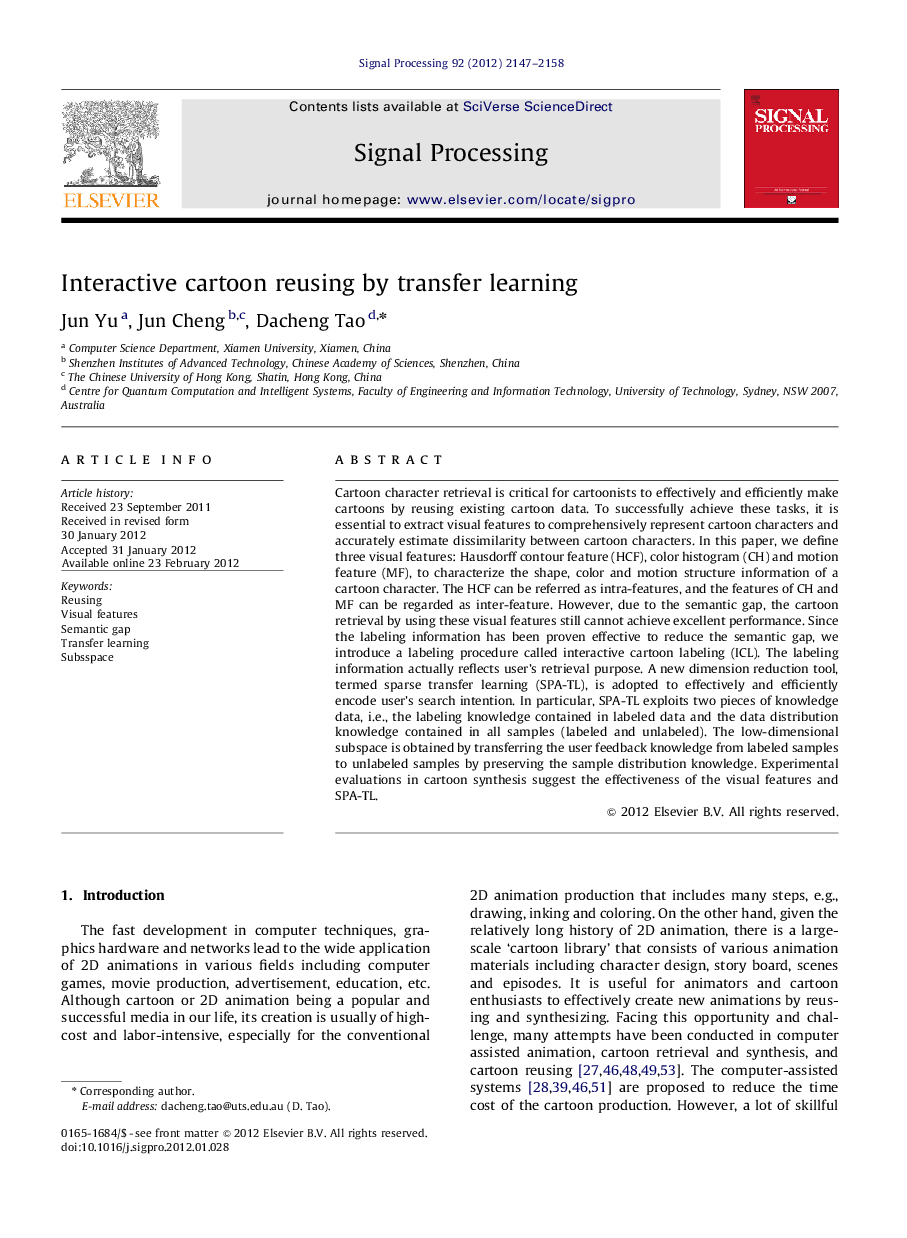
Cartoon character retrieval is critical for cartoonists to effectively and efficiently make cartoons by reusing existing cartoon data. To successfully achieve these tasks, it is essential to extract visual features to comprehensively represent cartoon characters and accurately estimate dissimilarity between cartoon characters. In this paper, we define three visual features: Hausdorff contour feature (HCF), color histogram (CH) and motion feature (MF), to characterize the shape, color and motion structure information of a cartoon character. The HCF can be referred as intra-features, and the features of CH and MF can be regarded as inter-feature. However, due to the semantic gap, the cartoon retrieval by using these visual features still cannot achieve excellent performance. Since the labeling information has been proven effective to reduce the semantic gap, we introduce a labeling procedure called interactive cartoon labeling (ICL). The labeling information actually reflects user’s retrieval purpose. A new dimension reduction tool, termed sparse transfer learning (SPA-TL), is adopted to effectively and efficiently encode user’s search intention. In particular, SPA-TL exploits two pieces of knowledge data, i.e., the labeling knowledge contained in labeled data and the data distribution knowledge contained in all samples (labeled and unlabeled). The low-dimensional subspace is obtained by transferring the user feedback knowledge from labeled samples to unlabeled samples by preserving the sample distribution knowledge. Experimental evaluations in cartoon synthesis suggest the effectiveness of the visual features and SPA-TL.
► Sparse transfer learning is used to explore user’s intention for cartoon synthesis.
► We present sparse transfer learning to effectively and efficiently encode user’s search intention.
► Experimental evaluations in cartoon synthesis suggest the effectiveness of our study.
Journal: Signal Processing - Volume 92, Issue 9, September 2012, Pages 2147–2158