کد مقاله | کد نشریه | سال انتشار | مقاله انگلیسی | نسخه تمام متن |
---|---|---|---|---|
566537 | 875994 | 2013 | 9 صفحه PDF | دانلود رایگان |
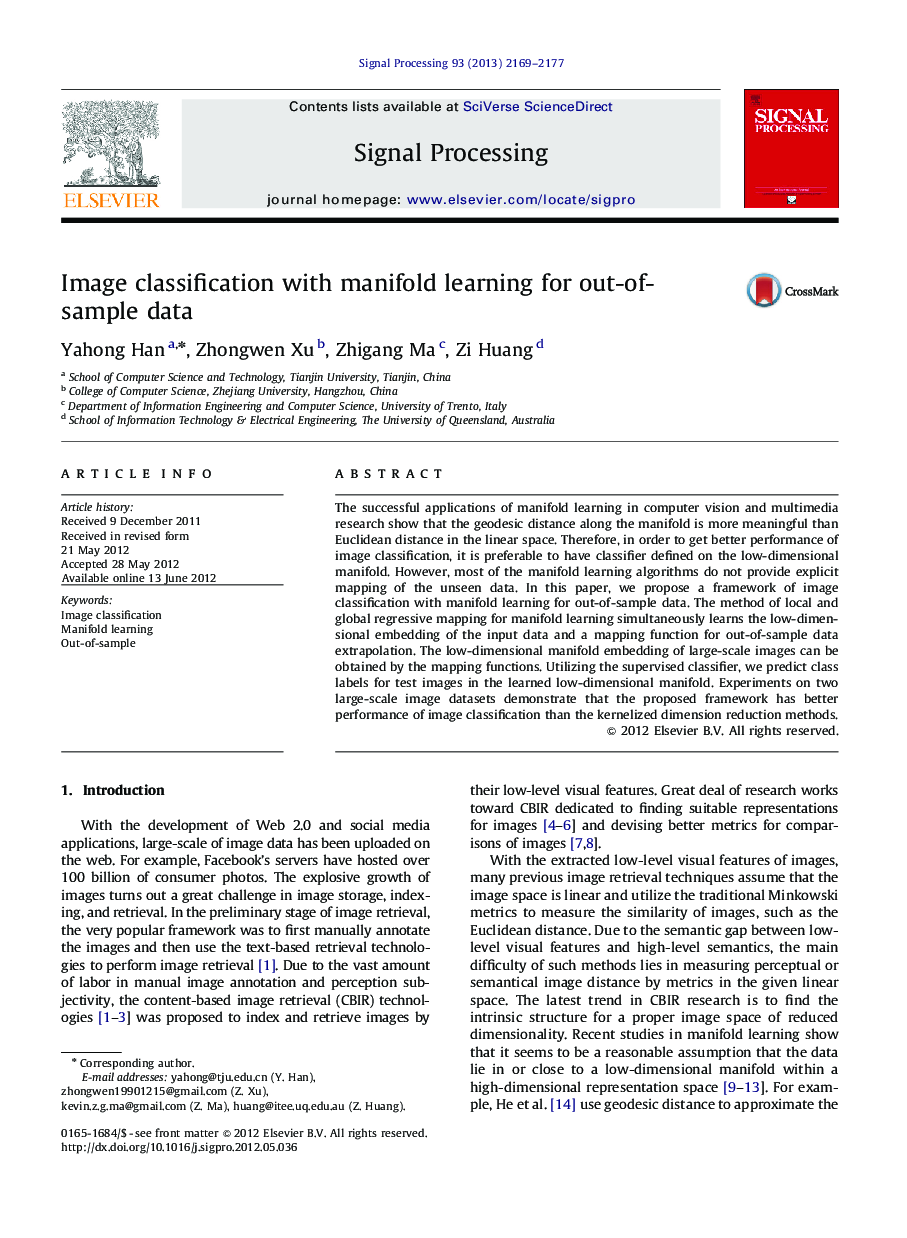
The successful applications of manifold learning in computer vision and multimedia research show that the geodesic distance along the manifold is more meaningful than Euclidean distance in the linear space. Therefore, in order to get better performance of image classification, it is preferable to have classifier defined on the low-dimensional manifold. However, most of the manifold learning algorithms do not provide explicit mapping of the unseen data. In this paper, we propose a framework of image classification with manifold learning for out-of-sample data. The method of local and global regressive mapping for manifold learning simultaneously learns the low-dimensional embedding of the input data and a mapping function for out-of-sample data extrapolation. The low-dimensional manifold embedding of large-scale images can be obtained by the mapping functions. Utilizing the supervised classifier, we predict class labels for test images in the learned low-dimensional manifold. Experiments on two large-scale image datasets demonstrate that the proposed framework has better performance of image classification than the kernelized dimension reduction methods.
► We propose a framework of image classification with manifold learning for out-of-sample data.
► We learn the low-dimensional embedding of the input images.
► We learn a mapping function for out-of-sample images.
► We get better performance compared with kernelized dimension reduction methods.
Journal: Signal Processing - Volume 93, Issue 8, August 2013, Pages 2169–2177