کد مقاله | کد نشریه | سال انتشار | مقاله انگلیسی | نسخه تمام متن |
---|---|---|---|---|
566547 | 875994 | 2013 | 10 صفحه PDF | دانلود رایگان |
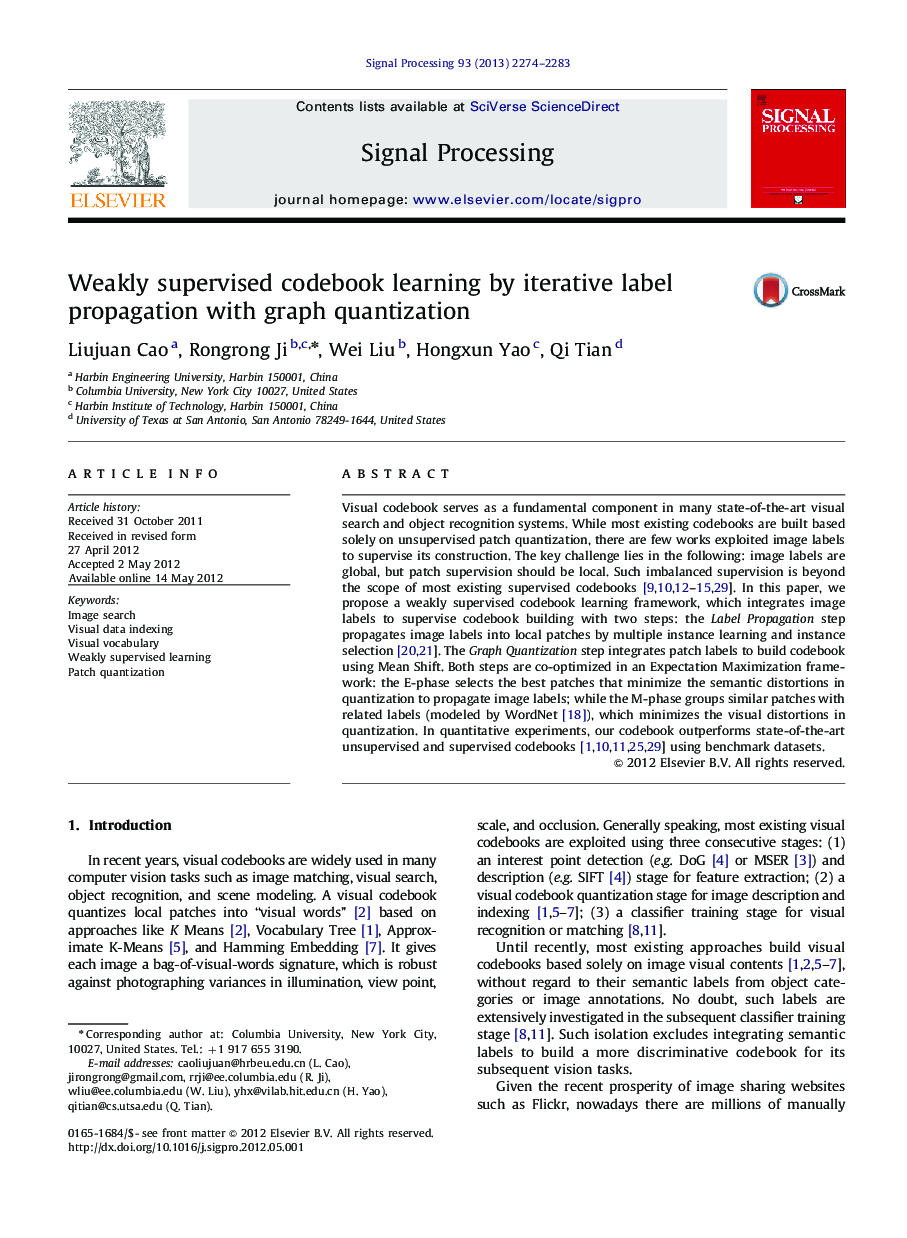
Visual codebook serves as a fundamental component in many state-of-the-art visual search and object recognition systems. While most existing codebooks are built based solely on unsupervised patch quantization, there are few works exploited image labels to supervise its construction. The key challenge lies in the following: image labels are global, but patch supervision should be local. Such imbalanced supervision is beyond the scope of most existing supervised codebooks [9], [10], [12], [13], [14], [15] and [29]. In this paper, we propose a weakly supervised codebook learning framework, which integrates image labels to supervise codebook building with two steps: the Label Propagation step propagates image labels into local patches by multiple instance learning and instance selection [20] and [21]. The Graph Quantization step integrates patch labels to build codebook using Mean Shift. Both steps are co-optimized in an Expectation Maximization framework: the E-phase selects the best patches that minimize the semantic distortions in quantization to propagate image labels; while the M-phase groups similar patches with related labels (modeled by WordNet [18]), which minimizes the visual distortions in quantization. In quantitative experiments, our codebook outperforms state-of-the-art unsupervised and supervised codebooks [1], [10], [11], [25] and [29] using benchmark datasets.
► Unsupervised visual codebook indexing cannot capture higher-level semantic of visual data.
► A weakly supervised codebook learning framework.
► Step 1: Label Propagation from image labels into local patches by multiple instance learning.
► Step 2: Graph Quantization to integrate patch labels to build codebook using Mean Shift.
► Quantitatively outperforms state-of-the-art unsupervised and supervised codebooks in benchmark datasets.
Journal: Signal Processing - Volume 93, Issue 8, August 2013, Pages 2274–2283