کد مقاله | کد نشریه | سال انتشار | مقاله انگلیسی | نسخه تمام متن |
---|---|---|---|---|
567441 | 876079 | 2006 | 12 صفحه PDF | دانلود رایگان |
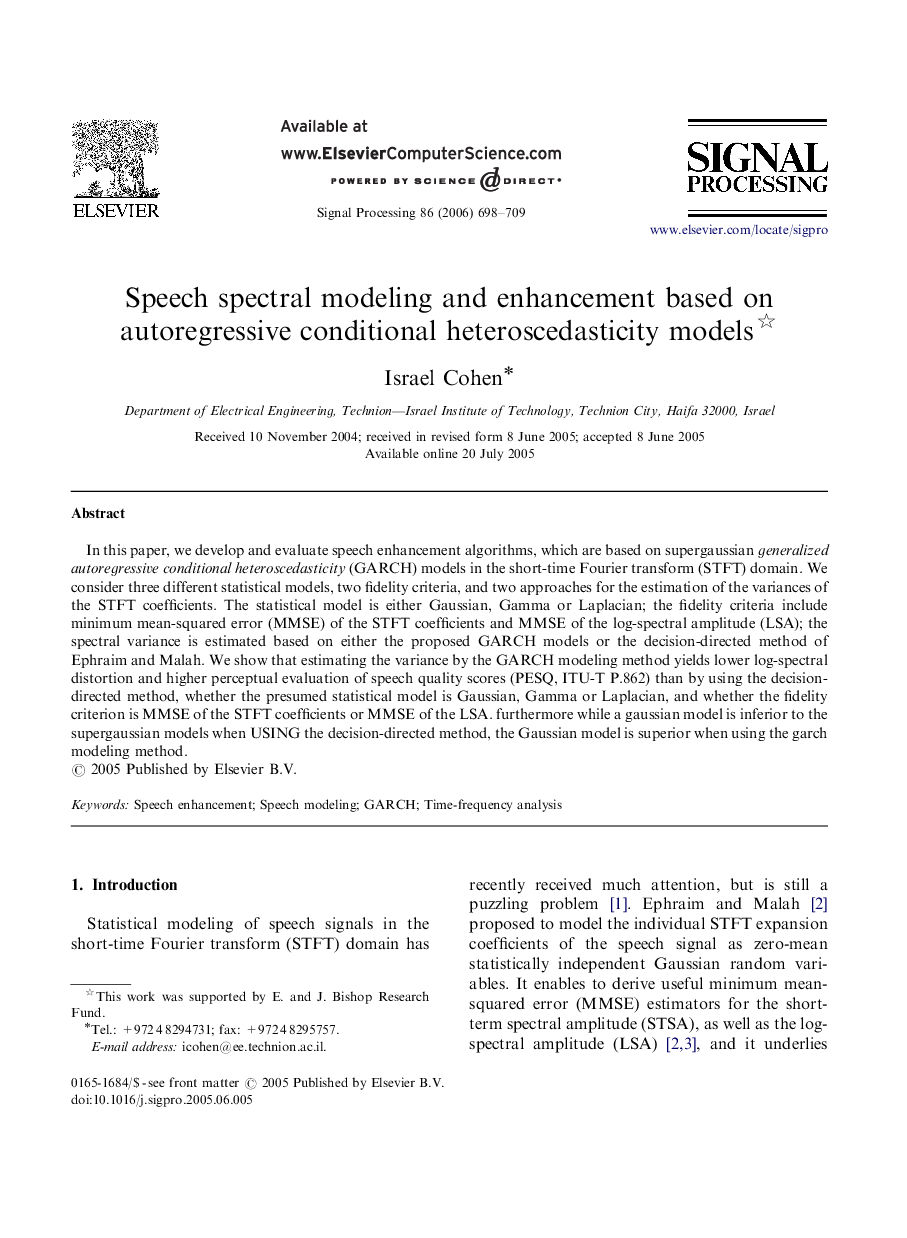
In this paper, we develop and evaluate speech enhancement algorithms, which are based on supergaussian generalized autoregressive conditional heteroscedasticity (GARCH) models in the short-time Fourier transform (STFT) domain. We consider three different statistical models, two fidelity criteria, and two approaches for the estimation of the variances of the STFT coefficients. The statistical model is either Gaussian, Gamma or Laplacian; the fidelity criteria include minimum mean-squared error (MMSE) of the STFT coefficients and MMSE of the log-spectral amplitude (LSA); the spectral variance is estimated based on either the proposed GARCH models or the decision-directed method of Ephraim and Malah. We show that estimating the variance by the GARCH modeling method yields lower log-spectral distortion and higher perceptual evaluation of speech quality scores (PESQ, ITU-T P.862) than by using the decision-directed method, whether the presumed statistical model is Gaussian, Gamma or Laplacian, and whether the fidelity criterion is MMSE of the STFT coefficients or MMSE of the LSA. furthermore while a gaussian model is inferior to the supergaussian models when USING the decision-directed method, the Gaussian model is superior when using the garch modeling method.
Journal: Signal Processing - Volume 86, Issue 4, April 2006, Pages 698–709