کد مقاله | کد نشریه | سال انتشار | مقاله انگلیسی | نسخه تمام متن |
---|---|---|---|---|
6295731 | 1617204 | 2015 | 26 صفحه PDF | دانلود رایگان |
عنوان انگلیسی مقاله ISI
MIAT: Modular R-wrappers for flexible implementation of MaxEnt distribution modelling
دانلود مقاله + سفارش ترجمه
دانلود مقاله ISI انگلیسی
رایگان برای ایرانیان
کلمات کلیدی
موضوعات مرتبط
علوم زیستی و بیوفناوری
علوم کشاورزی و بیولوژیک
بوم شناسی، تکامل، رفتار و سامانه شناسی
پیش نمایش صفحه اول مقاله
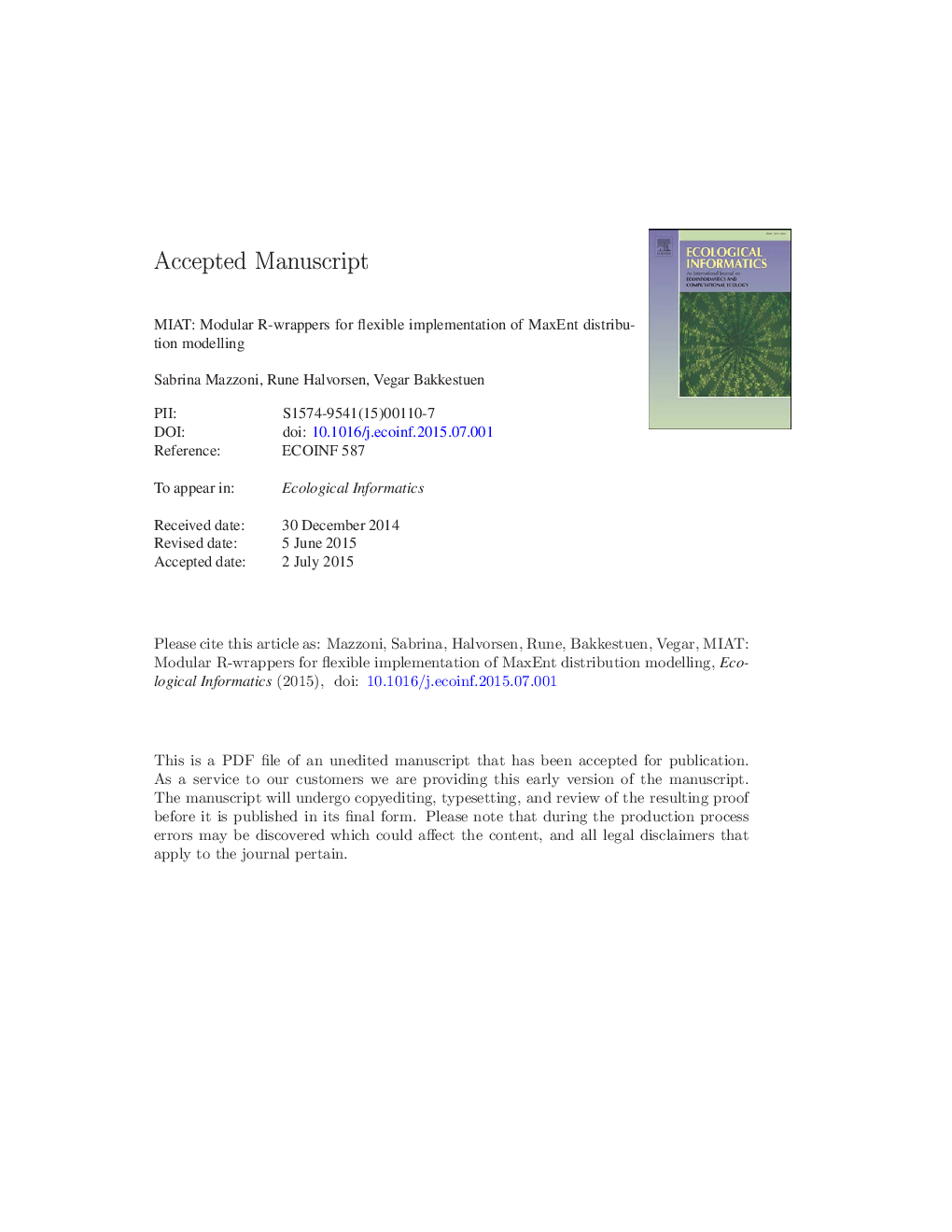
چکیده انگلیسی
The maximum entropy (MaxEnt) method has gained widespread use for distribution modelling, mostly because of the practical simplicity offered by the maxent.jar software. Whilst MaxEnt was originally described as a machine learning method, recent studies have shown that the method can be explained in terms of maximum likelihood estimation. This opens for using MaxEnt with new settings and options, such as new model selection and model assessment criteria, and improved user control of the variable selection process. New practical tools are needed to explore the new opportunities and assess if they enhance model performance and ecological interpretability of the models. We present a new conceptual framework, the Modular and functionally Integrated component-based Approach (MIA) framework for practical distribution modelling by which the core components of the DM process are decoupled and then wrapped together more flexibly into component-based functional modules. Computational object-oriented and workflow approaches are integrated with ecological, statistical and modelling theory in order to handle the complexity associated with the full modelling process in a practical way. Objects (variables, functions, results, etc.) are defined according to specific modelling parameters. Properties (e.g., identities and content) are inherited between objects and new objects are created in a flexible and automated, yet traceable way. We operationalise this framework for MaxEnt by the MIA Toolbox (MIAT), a set of flexible, modular R-scripts (available in supplementary appendices) wrapped around maxent.jar and existing R-functions. MIAT covers the full range of options and settings for the maximum likelihood implementation of MaxEnt and provide flexible guidance of users through the DM process. A trail of models of increasing complexity is built to enhance traceability and interpretability, and to suit different modelling purposes. We briefly outline research questions that can be addressed by the MIAT.
ناشر
Database: Elsevier - ScienceDirect (ساینس دایرکت)
Journal: Ecological Informatics - Volume 30, November 2015, Pages 215-221
Journal: Ecological Informatics - Volume 30, November 2015, Pages 215-221
نویسندگان
Sabrina Mazzoni, Rune Halvorsen, Vegar Bakkestuen,